Health Data Analytics is changing the way we identify and treat diseases. The goal now is to understand as much as possible about a patient and avoid health-related complications as early as possible.
Health Data Analytics is the process of performing various analytical operations on historical and current data to predict and identify trends and patterns, get actionable insights and control the spread of diseases.
This article will discuss Health Data Analytics (Healthcare Analytics), its applications, and Data Visualization in Healthcare Analytics.
Table of Contents
Introduction to Health Data Analytics
- The foundation of Health Data Analytics is robust Data Analysis. Data Analysis refers to performing operations on a dataset to get insights and use them to improve an organization’s decision-making process.
- Health Data Analytics is basically the analysis of data using different techniques to discover patterns and trends and make use of this information to take actionable steps and guide the decision-making process.
- The main use case of Health Data Analytics is managing and controlling the spread of diseases.
- The analysis of this data could be based on patient care efforts, positive/negative feedback, or other real-time information that can help in the decision-making.
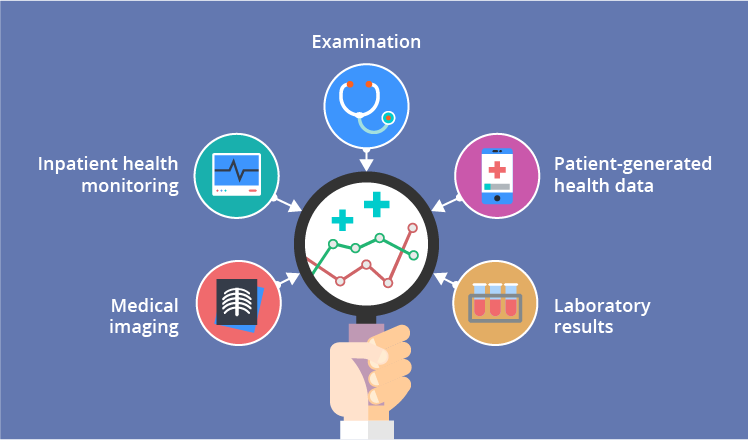
The crux of Data Analytics in Healthcare is disease control amongst others.
Are you looking for an ETL tool to migrate your data in one location to perform analytics? Migrating your data can become seamless with Hevo’s no-code intuitive platform. With Hevo, you can:
- Automate Data Extraction: Effortlessly pull data from various sources and destinations with 150+ pre-built connectors.
- Transform Data effortlessly: Use Hevo’s drag-and-drop feature to transform data with just a few clicks.
- Seamless Data Loading: Quickly load your transformed data into your desired destinations, such as BigQuery.
- Transparent Pricing: Hevo offers transparent pricing with no hidden fees, allowing you to budget effectively while scaling your data integration needs.
Try Hevo and join a growing community of 2000+ data professionals who rely on us for seamless and efficient migrations.
Get Started with Hevo for FreeApplications of Health Data Analytics
Implementing a Health Data Analytics Suite can help Healthcare providers to leverage data for insights in various fields of operations. Many healthcare providers collaborate with a specialized healthcare software agency to design and deploy these analytics suites, ensuring they are customized to their specific operational needs and compliance requirements. Integrating a Medication alternatives tool within the suite empowers providers to identify cost-effective and clinically appropriate treatment options, enhancing patient care outcomes. Some of them are as follows:
1. Disease Control
- Data Analytics can be used in Healthcare in the control of disease in different forms (hereditary, contagious, etc) by recognizing potential issues in patients beforehand.
- Genetic information and historical patterns can be analyzed to draw insights and identify the problems whenever they occur.
- Data Analytics can be used to develop smart devices to analyze patient behaviors at an early stage and predict possible outcomes based on the data.
2. Tracking Individual Performances
- The feedback from patients using an organization’s Healthcare Service can serve as data. This data(e.g experience with their practitioners) can determine whether their practitioners need more training to ensure patient’s safety and improve their service overall.
- All these would be tedious without Data Analytics in place to bridge the gap between trials and errors and effective analysis.
3. Track the Health of a Population
- Health Data Analytics can be used to identify and predict trends of a certain illness. The data collected can predict future outbreaks and help hospitals, schools, offices, etc prepare adequately.
- Health Data Analytics is used to avoid the abuse of certain medications and drugs. For example, The Center for Disease Control( CDC) stated “On average, 130 Americans die daily from opioid overdose”. Data Analytics can be used to analyze potential risk factors causing the deaths and predict if a person is at risk or not.
4. Diagnosis and Treatment
- Research has shown that patient cycles of Diagnosis and Treatment often differ significantly from standardized clinical pathways. The analysis of these deviations could lead to a further improvement in the quality of care, the promotion of patient safety, an increase in patient satisfaction, and optimization of the application.
- Understanding the routing behavior and deviations are made possible by the increased availability of reliable data from hospital information systems.
4 Key Tools Used in Health Data Analytics
There are several domains of technology that are involved in Health Data Analytics. Some of them are mentioned below:
1. Cloud Computing
- Healthcare systems handle a lot of data. The storage and processing of these data efficiently depend on the IT infrastructure it sits on.
- Cloud Computing eliminates the cost of investing in On-Premise infrastructure and deployments. Leading healthcare software development services can facilitate seamless cloud integration while ensuring compliance with industry regulations and data security standards. Although On-Premise databases offer relatively more control, Healthcare organizations are constantly looking for scalable alternatives.
- Ensuring compliance with regulations like the LEIE list is crucial when transitioning to cloud-based solutions.
- Examples of Cloud Computing tools are Data Warehouses. Data Warehouses allow you to get data from different sources and perform real-time analysis on them. Examples include Amazon Athena, Redshift, Snowflake, Google BigQuery, etc.
2. Data Visualization
There are different Data Visualization tools available with different pricing plans and capabilities. They include:
3. Machine Learning
- In recent years, Health Data Analytics has become one of the most promising areas of research.
- Healthcare includes data of various types, such as Clinical data, Omics data, and Sensor data. Clinical data includes electronic medical records that store the records of patients collected during treatment.
- Omics data is one of the high-level data that includes genome, transcriptome, and proteome data types. Sensor data is collected from various portable and wireless sensor devices used in the Healthcare Industry.
- Manipulating this raw data is very difficult and this is where Machine Learning proves to be a significant tool.
- Machine learning uses various statistical techniques and advanced algorithms to predict health data outcomes with greater precision.
- Machine learning uses various algorithms such as Supervised Learning, Unsupervised Learning, Decision Tree algorithm, and many more for analysis.
4. Database Management System
- With the exponential involvement of technology in the Healthcare industry, there is the generation of a large number of data points, therefore it becomes equally important to store the data in such a manner that the retrieval of information becomes easier and this is where Database Management System comes into the picture.
- A well-designed Database Management System stores the data and makes it ready to be used for Data Analysis.
Conclusion
- Data Analytics in Healthcare is improving both Healthcare as an ecosystem and people’s lives. It is another field where data plays the ultimate role.
- In this article, you learned about the importance of Data Analytics in the Healthcare Industry. You also learned about the applications and the tools used in Health Data Analytics
- Extracting complex data from a diverse set of data sources to carry out an insightful analysis can be a challenging task and this is where Hevo saves the day!
Share your experience of learning about Health Data Analytics. Let us know in the comments section below!
FAQs
1. What is health data analytics?
Health data analytics involves collecting, processing, and analyzing healthcare data to uncover patterns, trends, and insights. It helps improve patient care, streamline operations, and support decision-making in areas like diagnosis, treatment planning, and public health initiatives.
2. What are the four types of data analytics in healthcare?
Healthcare data analytics can be categorized into four types. Descriptive analytics provides insights into past trends and events, such as patient admission rates. Diagnostic analytics focuses on identifying the causes of health issues by analyzing data patterns. Predictive analytics uses data to forecast future outcomes, like predicting disease outbreaks or patient readmission risks. Finally, prescriptive analytics recommends actionable steps to improve outcomes, such as optimizing treatment plans or resource allocation. Together, these analytics types empower better decision-making in healthcare.
3. How do I get into healthcare data analysis?
To get into healthcare data analysis, start by building a strong foundation in data analytics tools like Excel, SQL, Python, or R, and learn about healthcare systems and standards such as HL7 and HIPAA. Gaining a relevant degree or certification in health informatics or data analytics, along with practical experience through internships or projects, can help you break into the field.