About TextExpander
TextExpander is a SaaS product enabling efficient and consistent communication for teams through the automation of repetitive tasks.



TextExpander is a SaaS product enabling efficient and consistent communication for teams through the automation of repetitive tasks. The organization mainly focuses on selling to businesses in the North American market.
Just over a year ago, Jeff joined as the first data person within the team, and since then, has set up the entire data ecosystem at TextExpander.
When going through the process of setting up the data stack at TextExpander, Jeff was very strategic about the way that he approached it. As a lean team, it was important that others in the organization would be empowered to manage the data for their functions. He wished to create data champions within each function of the organization who form an interconnected network of data management. Every tool in the data stack would need to enable this vision.
The requirement: A small team with big data goals
Jeff is a veteran of data engineering and analytics with significant experience in building data infrastructure in startups. He knew that building the data stack correctly from Day 1 can avert a lot of issues down the line. In the beginning, analytics was run directly on TextExpander’s production database, MongoDB. It was not a conducive environment for analytics due to the nature of the NoSQL database. Jeff did not want to manage a data platform; he wanted to use a data platform without worrying about breakages or maintenance.
He had four main principles in mind for the tools he wished to onboard:
- It had to be fully cloud-based. It should not require any management on TextExpander's part- if any issues arose, they should be resolved without any intervention from Jeff's side.
- It had to be very scalable. As TextExpander grows, the tool should also have the ability to scale along with their growth.
- It had to provide a great onboarding and support experience. Many tools come with a lot of features but can be complicated to actually onboard, especially without adequate training and support.
- Jeff wanted to create a partnership with the company that he brought on; it should be a very symbiotic, personal relationship. If the platform was able to solve all of Jeff's needs completely, he wished to be able to become a strong advocate for the product and put his word behind the product with complete trust.
Out of all the tools that Jeff evaluated, including Informatica, Fivetran, Matillion, etc., Hevo was the clear winner for data ingestion when it came to all these categories, and Snowflake was the best data warehouse.
Within an hour after setting up Hevo and connecting it to Snowflake, all production data was available to query and analyze. There was a very clear line of sight when it came to scalability in the future.
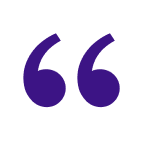
Hevo really showed me how it scales with our growth and created the onboarding experience I wanted. In two calls within one week, I had loaded all the data into Snowflake. I really felt that partnership, and over the years, that connection has not faded. This really feels like a shared partnership between us and Hevo.
The solution: A mature data infrastructure for analytics
Hevo Data has been a well-rounded boon to Jeff’s data stack. The support is not only helpful to resolve issues, but is also collaborative. They even help Jeff improve his knowledge on different aspects of data management at times. In an organization with a data team of one, an engaging and collaborative support like this is highly beneficial.
Hevo is easy-to-use, which means that data champions from the marketing and customer success teams have been granted access to Hevo Data and have been able to handle the data requirements for their functions on their own, despite limited technical knowledge. This reduces the burden on the data team, allowing Jeff to focus on advanced analytics projects instead of mere pipeline setup and maintenance. Most data requests can be solved within 15 minutes, and this ease-of-us is precisely what enables the data champions within TextExpander to empower themselves when it comes to data.
Lastly, Hevo Data is always reliable. TextExpander has never faced any issues of data loss or data mismatch, and the data is always up-to-date and accurate in Snowflake. Even if rows are deleted, Hevo marks them as deleted in the destination warehouse, allowing for traceability of even deleted rows. Jeff finds Hevo to be much more reliable than other tools he has used for data integration in the past. When things don’t go according to plan, it’s very easy to hit the reset button and have the complete and accurate data back in a working environment within minutes.
Using Hevo, TextExpander has built a very mature data architecture. Hevo is used to manage the entire data ingestion from the sources to Snowflake, model the data within the staging database using Hevo models, and then stored in the analytics database. All the raw application data from MongoDB as well as data from SaaS sources like Google Sheets, SurveyMonkey, HelpScout, etc. goes into ODS- operational data store- within Snowflake. From the ODS, the data is processed within a staging database. The final output, the modeled data for analytics, is stored in a database where downstream apps (Mode, Mixpanel, Hubspot CRM, etc) and all users have access to.
This structure ensures that the data is traceable, clean, and easy to manage at all stages of the process.
The Impact: Critical data projects enabled
Many critical projects have been enabled due to the introduction of Hevo Data and Snowflake within TextExpander. The use of a tool like Hevo ensures that Jeff saves critical bandwidth, and even new folks who enter the organization can do 95% of their own data analysis using the existing setup.
One major project has been the credit tracking system, which is important for TextExpander as they are a credit-based company. That credit management system, financial tracking, and calculation of MRR was built using the combination of Hevo + Snowflake, which allows TextExpander to calculate their active usage, daily MRR snapshots and overall product usage insights.
The marketing funnel is also enabled using the combination of Hevo and Snowflake. Questions like who is signing up, who is using various product features, and who is churning can be answered easily. This has allowed the marketing team to make data-driven decisions, allowing them to reduce their CPL on paid campaigns and focus their marketing activities. Jeff was previously spending about 20-25 hours per week on marketing data, but now that marketing has been empowered to manage all their data needs on their own, Jeff is able to save 100% of that time. Data is all available in an analytics-ready format within Snowflake, and the marketing team creates required views on top of that data. Since implementing Hevo, the marketing team has seen a sharp increase in trials, impressions, site visits, and most other business metrics.
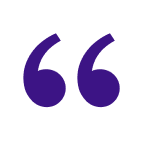
I was helping an individual who was spending 2 hours a day getting data to create a churn report based on that data. Ingesting and modeling that data into Snowflake using Hevo means that he spends only 10 minutes getting the data he needs, and can now spend that extra time diving into the reports. He’s also bringing product recommendations to improve retention to the leadership team using that data, which was facilitated by the data he was able to easily access.
All in all, Hevo feels proud to have enabled Jeff to single-handedly set up a robust, mature and sound data stack within TextExpander, and to have gained an outspoken advocate in the process. It’s a great feeling to enable people like Jeff and organizations like TextExpander derive value from their data.
Excited to see Hevo in action and understand how a modern data stack can help your business grow? Sign up for our 14-day free trial or register for a personalized demo with our product expert.