MongoDB is commonly used in modern web applications, data analytics, real-time processing, and other scenarios where flexibility and scalability are essential. However, MongoDB doesn’t have a proper join; getting data from other systems to MongoDB will be difficult, and it also has no native support for SQL. MongoDB’s aggregation framework makes it difficult to draft complex analytics logic as in SQL. Therefore, in the blog, we have provided you with 3 step-by-step methods that can help you integrate your data from MongoDB to BigQuery.
Also, take a look at the best real-world use cases of MongoDB to get a deeper understanding of how you can efficiently work with your data.
Table of Contents
MongoDB to BigQuery: Benefits & Use Cases
- Scalability and Speed: With BigQuery’s serverless, highly scalable architecture, you can manage and analyze large datasets more efficiently, without worrying about infrastructure limitations.
- Enhanced Analytics: BigQuery provides powerful, real-time analytics capabilities that can handle large-scale data with ease, enabling deeper insights and faster decision-making than MongoDB alone.
- Seamless Integration: BigQuery integrates smoothly with Google’s data ecosystem, allowing you to connect with other tools like Google Data Studio, Google Sheets, and Looker for more advanced data visualization and reporting.
Struggling with custom scripts to sync MongoDB and BigQuery? Hevo simplifies the process with a fully managed, no-code data pipeline that gets your data where it needs to be fast and reliably.
With Hevo:
- Connect MongoDB to BigQuery in just a few clicks.
- Handle semi-structured data effortlessly with built-in transformations.
- Automate schema mapping and keep your data analysis-ready.
Trusted by 2000+ data professionals at companies like Postman and ThoughtSpot. Rated 4.4/5 on G2. Try Hevo and make your MongoDB to BigQuery migration seamless!
Get Started with Hevo for FreeMethods to Integrate Your Data from MongoDB to BigQuery
- Method 1: Using Hevo Data to Automatic Stream Data from MongoDB to BigQuery
- Method 2: Using Confluent Connectors
- Method 3: Using Google Cloud Dataflow
Prerequisites
- MongoDB Source & BigQuery Sink connectors
- GCP service account key with BigQuery permissions
- MongoDB Atlas cluster with data
- BigQuery dataset in your GCP project
- Google Cloud Platform account with BigQuery access
- A Hevo account (free or paid)
- Access to Confluent Cloud/Platform
Method 1: Using Hevo Data to Automatic Stream Data from MongoDB to BigQuery
Step 1: Configure MongoDB as your Source
Step 2: Configure BigQuery as your Destination
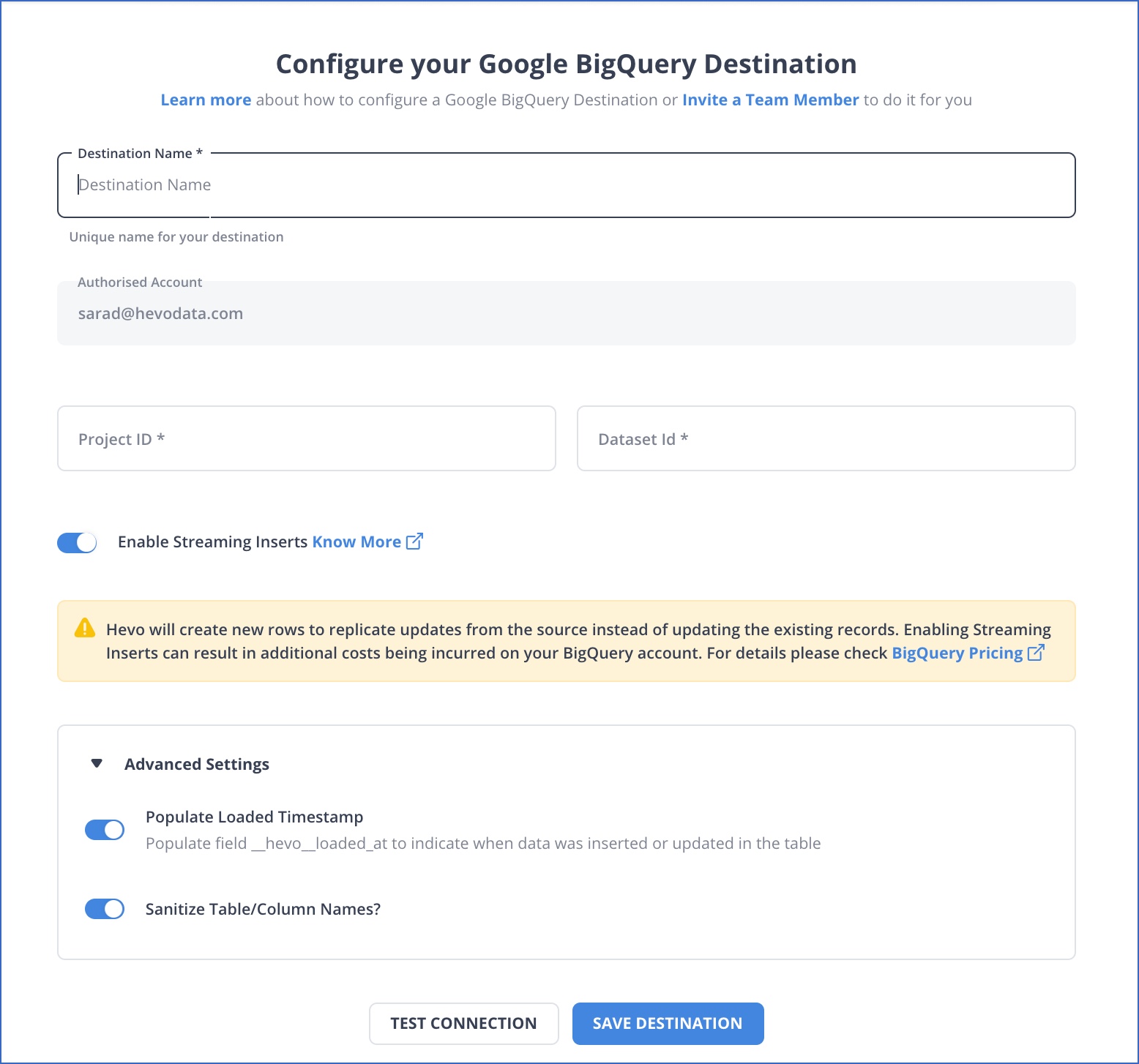
By following the above-mentioned steps, you will have successfully completed MongoDB BigQuery replication.
With continuous real-time data movement, Hevo allows you to combine MongoDB data with your other data sources and seamlessly load it to BigQuery with a no-code, easy-to-setup interface.
Method 2: Using Confluent Connectors
Step 1: Set Up MongoDB Atlas
- Create a cluster & load data
- Whitelist IP & get connection string
Step 2: Set Up Confluent
- Create Kafka cluster (with Connect & Schema Registry)
- Install MongoDB Source & BigQuery Sink connectors
Step 3: Deploy MongoDB Source Connector
jsonCopyEdit{
"connector.class": "com.mongodb.kafka.connect.MongoSourceConnector",
"connection.uri": "mongodb+srv://<username>:<password>@...",
"database": "sample_mflix",
"collection": "movies",
"output.format.value": "json",
"topic.prefix": "mongo."
}
Step 4: Create BigQuery Dataset
- In GCP Console, create a dataset
- Set up service account & download JSON key
Step 5: Deploy BigQuery Sink Connector
jsonCopyEdit{<br> "connector.class": "com.wepay.kafka.connect.bigquery.BigQuerySinkConnector",<br> "topics": "mongo.sample_mflix.movies",<br> "project": "<gcp-project-id>",<br> "datasets": "default=<dataset-name>",<br> "autoCreateTables": "true"<br>}
Step 6: Test the Pipeline
Insert docs in MongoDB → View in BigQuery
Method 3: Using Google Cloud Dataflow
Google Cloud Dataflow’s batch template is a reliable option for moving data from MongoDB to BigQuery, especially for large or scheduled migrations. The process involves providing MongoDB and BigQuery connection details, selecting the desired data format (flattened, JSON, or raw), and allowing Dataflow to manage the data transfer. For a detailed step-by-step guide, this blog provides a thorough walkthrough to connect MongoDB to BigQuery using Google Cloud Dataflow.
However, there are some common challenges to be aware of. Handling MongoDB’s ObjectId
fields or deeply nested documents can be complicated, as they don’t always map cleanly to BigQuery’s schema. Using User-Defined Functions (UDFs) to transform or extract these values is often necessary, but misconfigurations, such as incorrect function names, can cause the pipeline to fail. Additionally, choosing the “flatten” option may lead to errors or data loss if the documents are highly nested or complex.
For scenarios requiring real-time synchronization between MongoDB and BigQuery, the Change Data Capture (CDC) method using MongoDB Change Streams with Dataflow is recommended.
Conclusion
In this blog, we have provided you with 3 step-by-step methods to make migrating from MongoDB to BigQuery an easy everyday task for you! The methods discussed in this blog can be applied so that business data in MongoDB and BigQuery can be integrated without any hassle through a smooth transition, with no data loss or inconsistencies.
Sign up for a 14-day free trial with Hevo Data to streamline your migration process and leverage multiple connectors, such as MongoDB and BigQuery, for real-time analysis!
FAQ on MongoDB To BigQuery
1. What is the difference between BigQuery and MongoDB?
BigQuery is a fully managed data warehouse for large-scale data analytics using SQL. MongoDB is a NoSQL database optimized for storing unstructured data with high flexibility and scalability.
2. How do I transfer data to BigQuery?
Use tools like Google Cloud Dataflow, BigQuery Data Transfer Service, or third-party ETL tools like Hevo Data for a hassle-free process.
3. Is BigQuery SQL or NoSQL?
BigQuery is an SQL database designed to run fast, complex analytical queries on large datasets.
4. What is the difference between MongoDB and Oracle DB?
MongoDB is a NoSQL database optimized for unstructured data and flexibility. Oracle DB is a relational database (RDBMS) designed for structured data, complex transactions, and strong consistency.