Bigquery is a widely used data warehouse that is able to process and store raw data efficiently. It provides various function to help in performing the tasks efficiently. Windows functions are used to process the data and perform analytical tasks on the same.
This article gives a guide on BigQuery Dense_rank and rank functions in detail.
Table of Contents
What is BigQuery?
Google BigQuery is the product offered by Google Cloud Platform, which is serverless, cost-effective, highly scalable data warehouse capabilities along with built-in Machine Learning features. Google Bigquery supports ANSI SQL, allowing users to execute SQL queries on massive datasets to manage business transactions, perform data analytics, and many more.
BigQuery is getting popular nowadays, and many companies like Twitter use BigQuery to forecast the exact volume of packages for its various offerings.
BigQuery also supports Streaming data sources along with batch data.
Key Features of BigQuery:
- Scalable Architecture: BigQuery has a scalable architecture and offers a petabyte scalable system that users can scale up and down as per load.
- Faster Processing: Being a scalable architecture, BigQuery executes petabytes of data within the stipulated time and is more rapid than many conventional systems. BigQuery allows users to run analysis over millions of rows without worrying about scalability.
- Fully Managed: BigQuery is a product of Google Cloud Platform, and thus it offers fully managed and serverless systems.
- Security: BigQuery has the utmost security level that protects the data at rest and in flight.
- Real-time data ingestion: BigQuery can perform real-time data analysis, thereby making it famous across all the IoT and Transaction platforms.
- Fault Tolerance: BigQuery offers replication that replicates data across multiple zones or regions. It ensures consistent data availability when the region/zones go down.
Hevo is a fully managed, no-code data pipeline platform that effortlessly integrates data from more than 150 sources into a data warehouse such as BigQuery. With its minimal learning curve, Hevo can be set up in just a few minutes, allowing users to load data without having to compromise performance. Its features include:
- Connectors: Hevo supports 150+ integrations to SaaS platforms, files, Databases, analytics, and BI tools. It supports various destinations, including Google BigQuery, Amazon Redshift, and Snowflake.
- Transformations: A simple Python-based drag-and-drop data transformation technique that allows you to transform your data for analysis.
- Schema Management: Hevo eliminates the tedious task of schema management. It automatically detects the schema of incoming data and maps it to the destination schema.
- Real-Time Data Transfer: Hevo provides real-time data migration, so you can always have analysis-ready data.
- 24/7 Live Support: The Hevo team is available 24/7 to provide exceptional support through chat, email, and support calls.
Try Hevo today to experience seamless data transformation and migration.
Get Started with Hevo for FreeWhat is Window Function?
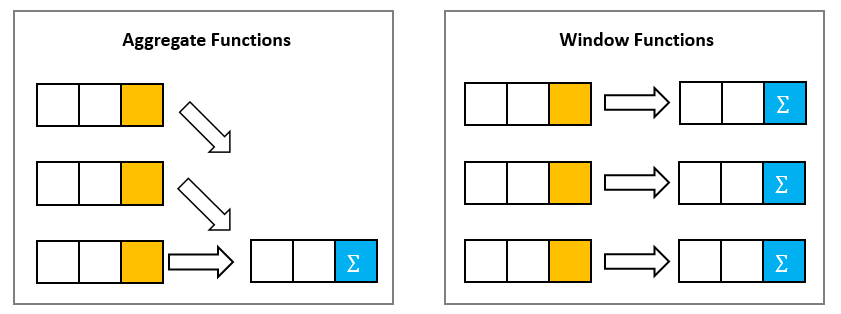
Window functions are analytical functions that enable users to create and execute analytical queries efficiently. The window function operates on a partition or window of defined limit and returns the result for each window. For example, if the user wants to sum the salary of all the employees based on department, the window function will first all the rows for the same department and then perform the sum against the department.
The aggregations are calculated based on the rows present in that particular window, and it is based on three main concepts –
- Partition clause that defines the partition value to create a window
- Over clause to perform ordering of the rows within each window/partition.
- Window frames are defined relative to each row to further restrict the rows’ set.
What is the Dense_Rank function?
The Bigquery Dense_Rank function is similar to other window functions except that it doesn’t skip any ranking number if there is a tie in the preceding rankings. For example – if there are two records with the Dense_Rank assigned as 1, then the next increment for the third record will start from 2 (Not ‘3’, as with the Rank function).
How to use the Google BigQuery DENSE_RANK Function?
The implementation of Bigquery Dense_Rank in Google BigQuery is simple as it has an implementation in SQL.
Consider the following table in BigQuery –
Orders
order_id | order_date | prodcut_id | order_qty |
O1 | 2022-01-02 01:12:23 | P01 | 1 |
O1 | 2022-01-02 01:12:23 | P02 | 1 |
O2 | 2022-01-04 02:12:23 | P01 | 2 |
O3 | 2022-01-06 06:12:23 | P03 | 2 |
Syntax
The syntax for the Bigquery Dense_Rank function is
DENSE_RANK () OVER
(
PARTITION BY <col name>
ORDER BY <col name>
)
Example
Consider the above Orders table. Let us try to implement the Bigquery Dense_Rank function in the Orders table and see the outcome –
order_id | order_date | prodcut_id | order_qty | dense_rank |
O1 | 2022-01-02 01:12:23 | P01 | 1 | 1 |
O1 | 2022-01-02 01:12:23 | P02 | 1 | 1 |
O2 | 2022-01-04 02:12:23 | P01 | 2 | 2 |
O3 | 2022-01-06 06:12:23 | P03 | 2 | 3 |
The above table shows ties between two rows, the next counter value assigned to the third row 2.
BigQuery DENSE_Rank vs RANK Function: What is the Difference and similarities?
The differences and similarities between BigQuery Dense_Rank and Rank functions are –
- BigQuery Dense_rank and Rank are part of the window function, and both provide ranking to the rows based on the input parameters that define the window of implementation.
- BigQuery Dense_Rank doesn’t skip the rows when there are ties in between rows; however, in the case of the Rank function, it will skip the counter value assigns the next available counter. For example, in the above example, for the third row, the rank function will assign the number 3.
Let us understand the above two features with an example applied on the Orders table –
order_id | order_date | prodcut_id | order_qty | dense_rank | rnk |
O1 | 2022-01-02 01:12:23 | P01 | 1 | 1 | 1 |
O1 | 2022-01-02 01:12:23 | P02 | 1 | 1 | 1 |
O2 | 2022-01-04 02:12:23 | P01 | 2 | 2 | 3 |
O3 | 2022-01-06 06:12:23 | P03 | 2 | 3 | 4 |
Conclusion
This blog post discussed what BigQuery Dense_Rank is and how to use it effectively with BigQuery. We have also discussed the similarities and Differences between Dense_Rank and Rank function and understood an example.
BigQuery is a trusted data warehouse that a lot of companies use and store data as it provides many benefits but transferring data into it is a hectic task. The Automated data pipeline helps in solving this issue and this is where Hevo comes into the picture. Hevo Data is a No-code Data Pipeline and has awesome 150+ pre-built Integrations that you can choose from.Sign up for Hevo’s 14-day free trial and experience seamless data migration.
Frequently Asked Questions
1. What does rank do in BigQuery?
In BigQuery, the RANK function is used to assign a unique rank to each row within a partition of a result set.
2. Why use dense rank?
The DENSE_RANK function is similar to RANK but without gaps in the ranking sequence when there are ties.
3. What is dense ranking method?
The Dense Ranking Method ensures that there are no gaps in the sequence of ranks when there are ties.