Nowadays, when it comes to data management, every business has to make one critical decision: whether to use a Data Mesh or a Data Warehouse. Both are strong data management architectures, but they are designed to support different needs and various organizational structures. Selecting the right one can make or break how efficiently you manage and analyze data.
This blog will discuss Data Mesh vs Data Warehouse and compare their key differences. We shall also give insight into each technology’s limitations. By the end of this blog, you will know much better which one best suits your needs.
Table of Contents
What is Data Mesh?
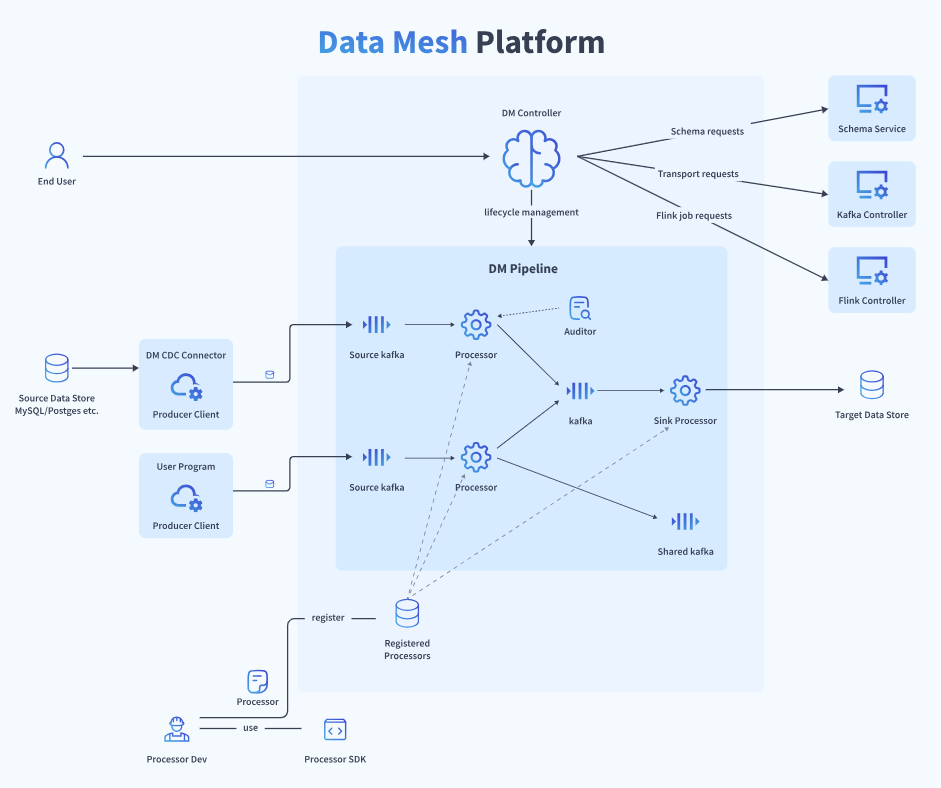
Data Mesh is a bit of a newer concept, it is based on a decentralized approach concerning data ownership. It means having just one central data team substituted by shared responsibilities across different domains or business units of an organization.
Are you looking for the best ETL tools to connect your data sources? Rest assured, Hevo’s no-code platform helps streamline your ETL process. Try Hevo and equip your team to:
- Integrate data from 150+ sources(60+ free sources).
- Utilize drag-and-drop and custom Python script features to transform your data.
- Risk management and security framework for cloud-based systems with SOC2 Compliance.
Key Features of Data Mesh
- Domain-oriented ownership: Teams are responsible for the data relevant to their domain.
- Data as a product: Each team manages their data like a product, ensuring it’s valuable and accessible.
- Self-serve data infrastructure: Teams have the autonomy to manage and use data tools.
- Federated governance: While governance is decentralized, there are still overarching guidelines to ensure security and quality.
What is a Data Warehouse?
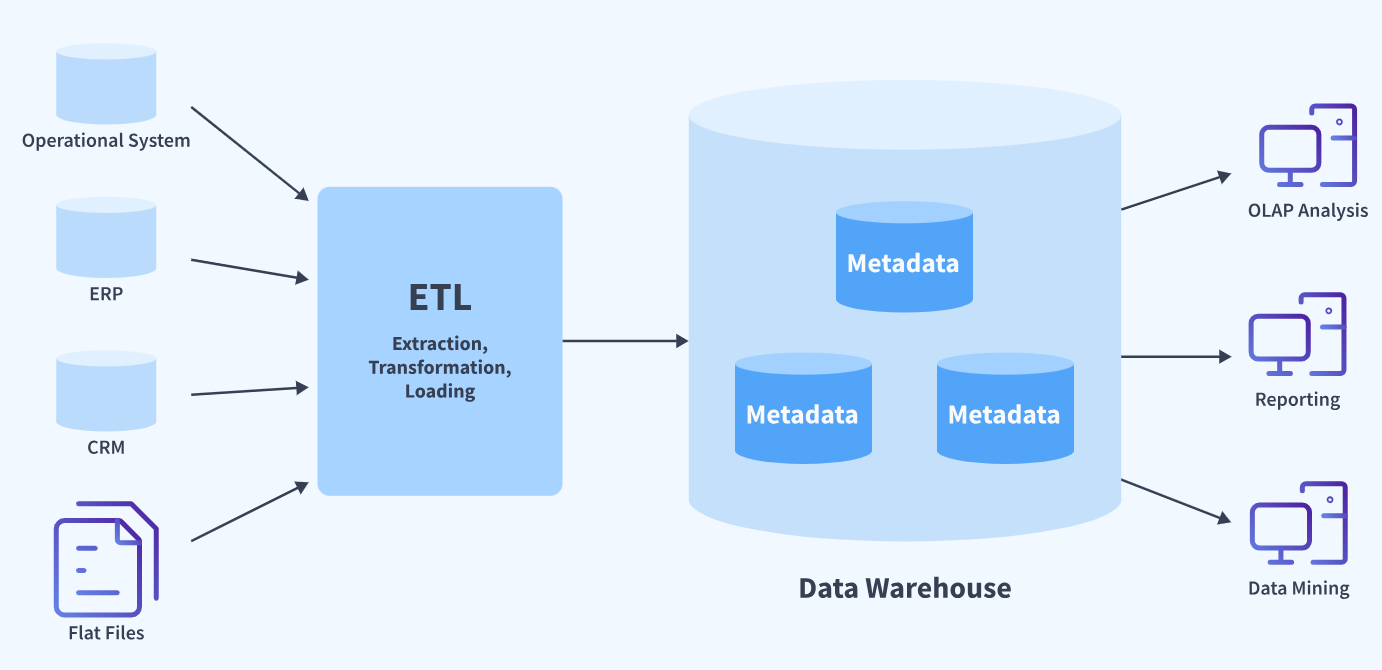
A data warehouse is a storage repository that contains volumes of data fed in from multiple sources. Conventionally, data warehouses have been ways through which companies retain, manage, and analyze structured data. Data is cleaned, transformed, and stored in a single location within a data warehouse, making access and reporting easy for teams.
Key Features of Data Warehouse
- Subject-Oriented: Data is organized around specific subjects or themes (e.g., sales, customers, products) to facilitate analysis.
- Integrated Data: It brings together data from various sources into a cohesive, unified view, ensuring consistency and accuracy.
- Time-Variant: Data warehouses store historical data to track changes over time, supporting trend analysis and long-term reporting.
- Non-Volatile: Once data is entered into the warehouse, it remains stable and is not modified, ensuring data integrity for reporting and analysis.
Criteria Comparison: Data Mesh vs Data Warehouse
Criteria | Data Mesh | Data Warehouse |
Architecture | Decentralized, domain-driven data management | Centralized, monolithic architecture |
Data Ownership | Distributed across domains, each team manages its data | Centralized ownership, managed by a single team |
Data Processing | Supports both batch and real-time streaming | Primarily batch processing |
Scalability | Scales with domain autonomy and distributed teams | Limited scalability due to centralized infrastructure |
Data Governance | Federated governance across domains, shared responsibility | Centralized governance, strict control over data quality |
Infrastructure | Self-serve data infrastructure per domain | Centralized Infrastructure requiring extensive management. |
Use Case Fit | Best for complex organizations with distributed data teams | Ideal for reporting, analytics, and historical data storage |
Data Access | Decentralized, domain-specific data access | Centralized, unified data access for all teams |
Cost | It can be expensive due to the decentralized infrastructure | Cost-effective for centralized, controlled environments |
Implementation Complexity | High requires a culture shift and advanced tooling. | Moderate, well-understood traditional data management. |
Detailed Comparison: Data Mesh vs Data Warehouse
Architecture
- Data Mesh
- Decentralized, domain-oriented architecture where data ownership lies with domain-specific teams.
- Promotes a distributed model where different teams manage their data as a product.
- Data Warehouse
- Centralized architecture where all data is aggregated and stored in a single repository.
- Uses a uniform, organization-wide structure for managing data.
Data Ownership
- Data Mesh
- Domain teams are responsible for data quality and management, following a “data as a product” approach.
- It shifts responsibility to domain experts to ensure the accuracy and relevance of their data.
- Data Warehouse
- The centralized data team manages and maintains all organizational data.
- Relies on data engineers and administrators for data quality and governance.
Scalability
- Data Mesh
- Scales more easily across multiple teams since each domain manages its own data.
- Allows independent scaling of data systems for each domain, reducing bottlenecks.
- Data Warehouse
- Scaling can be resource-intensive, as all data must flow through a central system.
- Larger data volumes may lead to performance issues and require significant infrastructure upgrades.
Data Processing
- Data Mesh
- Each domain manages its own processing and transformation of data.
- Enables real-time and domain-specific processing.
- Data Warehouse
- Data is processed centrally using standardized ETL (Extract, Transform, Load) pipelines.
- Typically, batch processing focuses on historical and structured data.
Cost
- Data Mesh
- Costs can increase as different domains require their own infrastructure and tools.
- It may reduce costs for specific domains but can lead to inefficiencies without proper coordination.
- Data Warehouse
- Centralized systems may have higher initial costs but are easier to manage long-term.
- More predictable and standardized cost management.
Factors to consider while choosing Data Mesh or Data Warehouse
When deciding between data mesh and data warehouse, consider the following factors to make the best choice for your organization:
- Organization Size and Complexity: If your organization is large and complex, with multiple departments that need autonomy over their data, data mesh may be a better fit.
- Data Team Capacity: If you have a small or overburdened central data team, Data Mesh can help distribute the load across different departments.
- Governance Requirements: If you need strict, centralized governance and consistent data quality across all departments, a data warehouse may be a better choice.
Limitations of Data Mesh
- Complexity in Implementation: Implementing a data mesh requires a cultural shift and advanced tooling, which can be a barrier for organizations that are not used to decentralized models.
- Governance Challenges: While federated governance allows for autonomy, maintaining consistent governance across domains can be tricky.
- Initial Cost: Setting up the infrastructure for Data Mesh can be more expensive initially, especially in terms of tooling and training.
Limitations of Data Warehouse
- Scalability Issues: As the amount of data increases, the centralized nature of the data warehouse can lead to bottlenecks and slowdowns.
- Centralized Bottlenecks: All data requests must go through a central team, potentially slowing down access to insights for departments.
- Limited Flexibility: In rapidly changing environments, centralized data models can be slow to adapt to new data requirements or sources.
How Hevo Simplifies Data Migration for Data Warehouses and Data Mesh
Hevo’s versatile data integration platform is instrumental in streamlining data migration, whether you’re leveraging a traditional Data Warehouse or adopting a modern Data Mesh approach.
- Hevo facilitates the smooth integration of diverse data sources into your centralized Data Warehouse. With support for various data formats and systems, it simplifies the ETL process, allowing you to aggregate and consolidate data efficiently.
- Hevo’s real-time data synchronization ensures that your Data Warehouse remains up-to-date with the latest information, enhancing the accuracy of your reports and business intelligence.
- Hevo’s no-code interface empowers individual teams to set up and manage their own data pipelines, facilitating self-serve data infrastructure and adhering to the Data Mesh philosophy of decentralized data management.
To better understand the core principles of data architecture, explore the key components and types in this insightful guide.
Conclusion
Both Data Mesh and Data Warehouse have their merits, and the right choice depends on your organization’s specific needs. Data Mesh is ideal for organizations that need scalability, agility, and decentralized data ownership. It’s particularly effective in large, distributed organizations where data teams need autonomy. On the other hand, Data Warehouse is a proven, reliable solution for organizations that prioritize centralized control, data consistency, and business intelligence.
Ultimately, the best decision comes down to how you want to structure your data management approach. Consider your organization’s size, data processing needs, and governance requirements before making a choice.
FAQ on Data Validation vs Data Warehouse
Is Data Mesh a Data Warehouse?
No, Data Mesh is a decentralized approach to managing data, where each domain or department is responsible for its own data. In contrast, a Data Warehouse is a centralized repository where all data is stored and managed by a central team.
What is the difference between Data Mesh and EDW (Enterprise Data Warehouse)?
The key difference is in the architecture. Data Mesh is decentralized, with each domain owning its data, while EDW (Enterprise Data Warehouse) centralizes all data in a single system managed by a dedicated team. Data Mesh promotes scalability and autonomy, while EDW focuses on centralized data management and governance.
What is Data Mesh vs Lake vs Warehouse?
Data Mesh: Decentralized, domain-based data ownership with federated governance.
Data Lake: A storage solution for unstructured and structured data, often used for big data analytics.
Data Warehouse: A centralized system designed for structured data, typically used for business intelligence and reporting.