If you’re reading this, you already know how important data quality can be in today’s fast-moving world for making critical business decisions. Now, be honest; you want to get high-quality data all the time—right? Use data quality tools.
This blog will discuss the top 10 data quality tools available today to ensure your data stays in line and your business stays on track. This includes real-time monitoring tools that alert you to problems, open-source solutions that are flexible and cost-effective, and much more. Ready to dive in? Let’s get started!
Table of Contents
What are Data Quality Tools?
Data quality tools are software solutions designed to ensure that an organization’s data is accurate, consistent, and reliable. These tools are of maximum importance in ensuring data integrity across various systems and processes.
Benefits of using Data Quality Tools
- Time and effort: Data quality tools automate time-consuming tasks, including data profiling, cleansing, and monitoring. This means less time is spent manually sifting through data in search of errors while more time is spent making strategic decisions.
- Better Decision Making: These tools help users make better decisions by ensuring the data is accurate, complete, and consistent—great data results in great insights, which drive business outcomes.
- Lower Costs: Investing in a data quality tool is more cost-effective than manually cleaning data. It’s also significantly cheaper to ensure data accuracy from the start rather than dealing with the mess and expense of fixing problems later.
Struggling with data quality? Hevo’s ETL platform ensures clean, consistent data flows from your sources to your destination.
Why Choose Hevo?
- Real-Time Data Sync: Ensure your analytics are always current.
- User-Friendly Interface: Easily manage and oversee your integrations.
- Security: Adheres to GDPR, SOC II, and HIPAA standards.
- Data Transformation: Refine, alter, and enrich your data with ease.
- Schema Management: Automatically map incoming data to your destination schema.
Top 10 Data Quality Tools
Let us explore the top 10 data quality tools now. I’ve listed a mix of monitoring tools, open-source options, and end-to-end management solutions so that there is something for everyone herAtaccama ONE
1. Attacama ONE
Gartner Rating: 4.5(67)
Ataccama ONE, a data quality monitoring tool, is your one-stop destination for data quality, governance, and management. It’s just one platform that is doing it all.
Key Features:
- Real-time data quality monitoring.
- AI-driven anomaly detection.
- Customizable dashboards for monitoring relevant metrics.
Use Case:
- Ideal for domains with strict data governance requirements (e.g., finance, healthcare).
- Suitable for large organizations needing comprehensive data governance.
- Effective for organizations requiring centralized data quality management.
2. Datafold
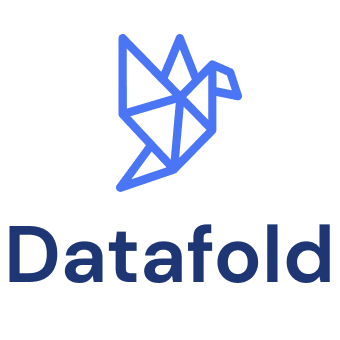
G2 Rating: 4.5(24)
Datafold is another data quality monitoring tool. If you are a data engineer, this tool will help you automate data quality monitoring like a dream.
Key Features:
- Real-time alerts for data quality issues.
- Automated regression testing for continuous data validation.
- Easy integration with data pipelines and ETL processes.
Use Cases:
- Perfect for data engineers working with complex data pipelines.
- Useful for continuous integration environments.
- Time-saving for teams managing multiple ETL processes.
3. Great Expectations
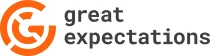
G2 Rating: 4.5(11)
Great Expectations is an open-source data quality tool. It helps validate data, document it, and create profiles. It allows users to define and validate data expectations through a user-friendly interface, enabling automated checks and reports on data quality.
Key Features:
- Open-source and customizable with community-driven plugins.
- Automated testing and validation of data.
- Documentation and data profiling capabilities.
Use Cases:
- It is ideal for data engineering or analytics teams needing flexible validation.
- Suitable for projects requiring custom data quality checks.
- It is beneficial for teams looking to build a robust data validation framework.
4. Apache Griffin
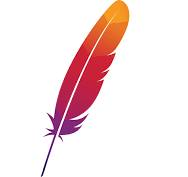
G2 Rating: NA
Apache Griffin is another open-source data quality tool focusing on large-scale data processing environments.
Key Features:
- Focus on data profiling, validation, and monitoring.
- Supports both batch and streaming data processing.
- Scalable for large data environments.
Use Cases:
- Best for big data environments.
- Suitable for data lakes and cloud-based ecosystems.
- Ideal for organizations needing real-time data quality monitoring.
5. Informatica Data Quality
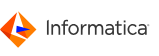
G2 Rating: 4.5(12)
Informatica is a data quality management tool and one of the big names in data quality. It is a comprehensive data management solution that ensures your data’s accuracy, consistency, and reliability across your organization.
Key Features:
- Comprehensive data profiling, cleansing, and enrichment.
- AI-driven recommendations for data quality improvements.
- Robust data governance capabilities.
Use Cases:
- Suitable for company-wide data quality programs.
- Perfect for large enterprises with complex data management needs.
- Ideal for industries where data quality is mission-critical.
6. SAS Data Management
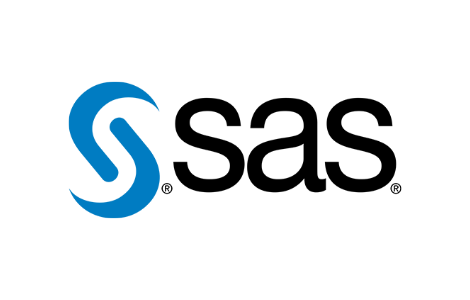
G2 Rating: 4.2(17)
SAS Data Management is a data quality management tool. It is a comprehensive data management powerhouse, offering a robust suite of tools for integrating, profiling, cleansing, and enriching data.
Key Features:
- Comprehensive data integration, profiling, and cleansing.
- Strong analytical capabilities for data enrichment.
- Advanced matching and deduplication features.
Use Cases:
- Ideal for financial services or healthcare sectors with complex data landscapes.
- Suitable for organizations requiring end-to-end data management solutions.
- Effective for companies needing robust data integration and quality.
7. Talend Data Quality
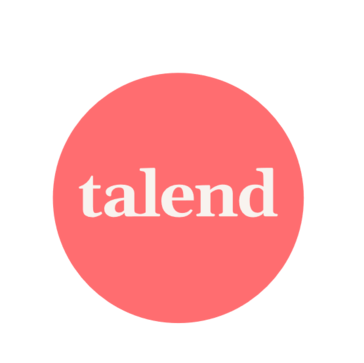
G2 Rating: 3.8(4)
Talend Data Quality is part of the Talend data integration platform and concentrates on keeping your data clean and compliant.
Key Features:
- Seamless integration with Talend’s broader data integration platform.
- Comprehensive data profiling and cleansing.
- Data deduplication and enrichment capabilities.
Use Cases:
- Best for medium to large enterprises needing integrated data solutions.
- Suitable for organizations focused on compliance and data cleanliness.
- Ideal for companies already using Talend’s data integration tools.
8. Precisely (formerly Syncsort)
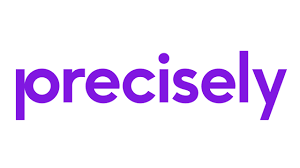
G2 Rating: 4.2(22)
Precisely offers data quality solutions for mainframes and large-scale data environments, thus becoming the go-to for legacy systems.
Key Features:
- Specialized in data governance and quality monitoring for mainframes.
- Real-time data enrichment tailored for large-scale environments.
- Robust support for legacy systems.
Use Cases:
- Ideal for organizations relying on legacy systems or mainframes.
- Suitable for large-scale data environments with specialized needs.
- Effective for industries requiring high levels of data governance.
9. Oracle Enterprise Data Quality
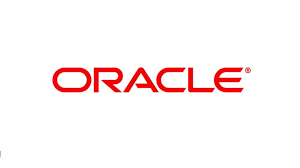
G2 Rating: 4.1(63)
Oracle Enterprise Data Quality (EDQ) is a comprehensive data management solution that improves data accuracy and consistency across an organization. It offers advanced data profiling, cleansing, matching, and enrichment capabilities, leveraging Oracle’s robust infrastructure to handle large-scale data environments.
Key Features:
- Enterprise-grade data profiling, cleansing, and matching.
- Integrated monitoring within Oracle’s ecosystem.
- Seamless integration with Oracle’s broader data management tools.
Use Cases:
- Best for organizations using Oracle’s data management stack.
- Suitable for enterprises needing comprehensive data quality management.
- Ideal for maintaining high data quality standards across large datasets.
10. Collibra Data Quality & Observability
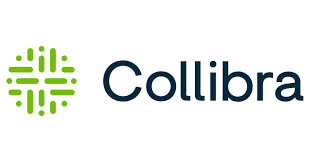
G2 Rating: 4.2(76)
Collibra’s Data Quality & Observability tool is a module in its greater data governance platform and can be leveraged to ensure high data standards.
Key Features:
- Automated data quality assessments.
- Lineage tracking and observability tools.
- Integration with Collibra’s data governance platform.
Use Cases:
- Ideal for organizations with stringent data governance and compliance needs.
- Suitable for companies requiring continuous data observability.
- Effective for ensuring high data standards across enterprise systems.
Comparison of Data Quality Tools
Tool | Type | Cost | Scalability | Usability | Integration Capabilities | Support and Community |
Informatica Data Quality | Data Quality Management | High | Highly scalable for enterprise environments | User-friendly interface, extensive features | Strong integration with various data sources and platforms | Premium support with a large, active community |
Ataccama ONE | Data Quality & Governance | Moderate to High | Scalable, suitable for large organizations | Intuitive with AI-driven features | Integrates well with multiple systems | Robust support with a growing user community |
Talend Data Quality | Data Quality & Integration | Moderate | Scalable, ideal for medium to large enterprises | Easy to use, with drag-and-drop interfaces | Seamless integration with Talend’s data management suite | Comprehensive support, active open-source community |
Datafold | Data Quality Monitoring | Moderate | Scalable, designed for modern data pipelines | Developer-friendly, specialized for engineers | Integrates well with modern data pipelines | Focused support, smaller but active community |
Great Expectations | Open-Source Data Quality | Accessible to Low (depends on customization) | Scalable for various environments | It is highly customizable but requires technical expertise | Flexible integration supports various data platforms | Strong open-source community with active development |
Apache Griffin | Open-Source Data Quality | Free | Scalable for big data environments | Requires technical expertise, less user-friendly | Integrates well with big data platforms | Growing open-source community, active development |
SAS Data Management | Data Quality & Management | High | Highly scalable for complex data landscapes | Feature-rich but has a learning curve | Extensive integration with various data systems | Premium support with extensive documentation |
Precisely (formerly Syncsort) | Data Quality & Governance | High | Scalable, especially for legacy systems | Focused on specialized environments | Integrates well with legacy and mainframe systems | Premium support with industry-specific resources |
Oracle Enterprise Data Quality | Enterprise Data Quality Management | High | Scalable for large enterprises | Feature-rich with a steeper learning curve | Deep integration with the Oracle ecosystem | Comprehensive support with dedicated Oracle resources |
Collibra Data Quality & Observability | Data Quality & Governance | High | Scalable for governance-focused organizations | User-friendly with robust governance features | Integrates well with Collibra’s governance platform | Firm support with an active enterprise community |
Criteria for selecting Data Quality Tools
Here are a few considerations to remember while choosing the right data quality tool that best fits your needs:
- Usability and interface: Is it easy to use? This needs to be evaluated, and it varies from person to person.
- Scalability and Performance: Will it work for your data size and complexity?
- Integration capabilities: Will it integrate well with your existing systems and data sources?
- Community and Support: Does the tool have a proper, responsive vendor support system or an active community behind it?
- Cost and ROI: Is it right-priced against your budget, and will it deliver good value for the money spent?
Conclusion
High data quality is essential when data is driving business success. Efficient data quality tools provide critical data cleansing, validation, and monitoring functionalities that give an organization confidence in its accurate and consistent data. By choosing the right tool, companies can do much more than ensure the integrity of their data; they can also make their data management easier.
A data quality tool is an investment in protecting the value of your data assets. These technologies provide a foothold for making informed decisions and strategic growth in the intricacies of digital transformation. Today’s priority to data quality will pay dividends for data-driven initiatives tomorrow.
FAQ on Data Quality Tools
1. What is a data quality tool?
A data quality tool is software that helps ensure data accuracy, consistency, completeness, and reliability within an organization. These tools automate the processes of data profiling, cleansing, standardization, validation, and monitoring.
2. What are five quality tools?
Here are five commonly used data quality tools:
1. Informatica Data Quality
2. Ataccama ONE
3. Talend Data Quality
4. Datafold
5. Great Expectations
3. What are the four categories of data quality?
Data quality can generally be categorized into four key dimensions:
1. Accuracy
2. Completeness
3. Consistency
4. Timeliness