Moving data from BigQuery to Snowflake isn’t always as easy as it sounds. Many teams run into problems like differences in how the two platforms store and organize data or errors that come up when trying to match table formats and column types. It can also be tough to move large amounts of data without slowing things down or spending more than expected. Plus, the SQL used in BigQuery doesn’t always work the same way in Snowflake, which means extra time spent rewriting queries. All of this makes the migration process confusing and time-consuming if you don’t have the right tools or plan in place.
But you don’t have to do it all manually. In this blog post, we’ll walk you through two ways to move your data:
- Using Hevo’s Automated Data Pipeline Platform for a fast, no-code setup, and
- A more hands-on approach with manual SQL scripts if you prefer to build the pipeline yourself.
Let’s dive into both options so you can choose the one that best fits your needs.
Table of Contents
What is BigQuery?
BigQuery is Google Cloud’s fully-managed, serverless data warehouse built for high-speed analytics on massive datasets. It shines when it comes to running ad hoc queries without worrying about infrastructure or performance tuning. Since it follows a pay-as-you-go pricing model based on the amount of data processed, it’s ideal for teams that want simplicity and are already using Google Cloud services.
Compared to Snowflake, BigQuery is more plug-and-play, making it great for quick analysis and rapid experimentation. However, it offers less flexibility when it comes to fine-tuning performance or isolating workloads across teams.
What is Snowflake?
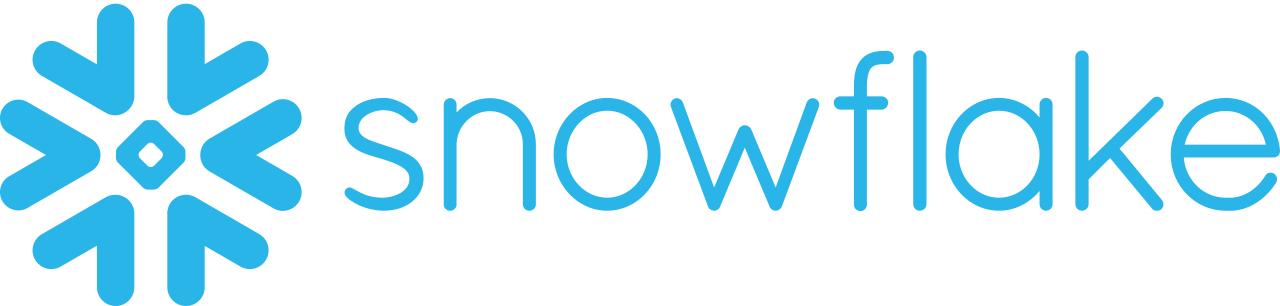
Snowflake is a modern cloud data warehouse available on AWS, Azure, and Google Cloud. It separates storage and compute, allowing you to scale each independently, and supports features like virtual warehouses, time travel, and semi-structured data out of the box. Snowflake’s pricing is based on compute and storage usage, which gives teams more control over costs and performance.
Unlike BigQuery, Snowflake gives users more hands-on control, whether it’s isolating workloads for different teams or tuning performance for specific jobs. It’s especially useful for organizations that need flexibility, multi-cloud deployment, or more granular resource management.
Prerequisites
- Snowflake account
- Billing enabled BigQuery account
- The bucket and dataset that need to be moved are present in BigQuery. For help loading data into BigQuery, refer to Hevo’s blog.
Why Replicate data from BigQuery to Snowflake?
Replicating data from BigQuery to Snowflake can be due to several business or technical requirements. Some of the requirements can be:
- Performance Optimization: Because Snowflake is architected differently, many workloads may execute far more efficiently on Snowflake. Replicated data can benefit from those performance optimisations Snowflake sends for specific scenarios.
- Cost Management: BigQuery charges both for storage and query processing; its costs rapidly get prohibitively costly for some query types. On cost management, Snowflake’s independent scaling of storage and computing could, in the future, provide lower cost choices for individual use cases.
- Disaster Recovery: Having data on both platforms creates redundancy and backup options, guaranteeing business continuity if one of the platforms experiences an outage or data corruption.
- Data Integration and Sharing: Snowflake has robust data-sharing features that make it easier to share data with other partners, customers, or even various departments of the company. Replicating data from BigQuery to Snowflake can make data sharing easier and more secure.
Planning to move your workloads from BigQuery to Snowflake? Hevo’s no-code data pipeline makes the transition seamless, with no scripts and no manual effort.
With Hevo:
✅ Move data from source to destination without writing a single line of code
✅ Handle large-scale migrations with built-in scalability and fault tolerance
✅ Auto-map schemas and maintain data integrity throughout the pipeline
Trusted by 2000+ data professionals, including teams at Playtomic and Cleeng. Make your Snowflake migrations hassle-free with Hevo today!
Start your Migration with Hevo Today!Method 1: Replicate Data from BigQuery to Snowflake using Hevo
Step 1: Configure BigQuery as a Source
Authenticate and configure your BigQuery source.
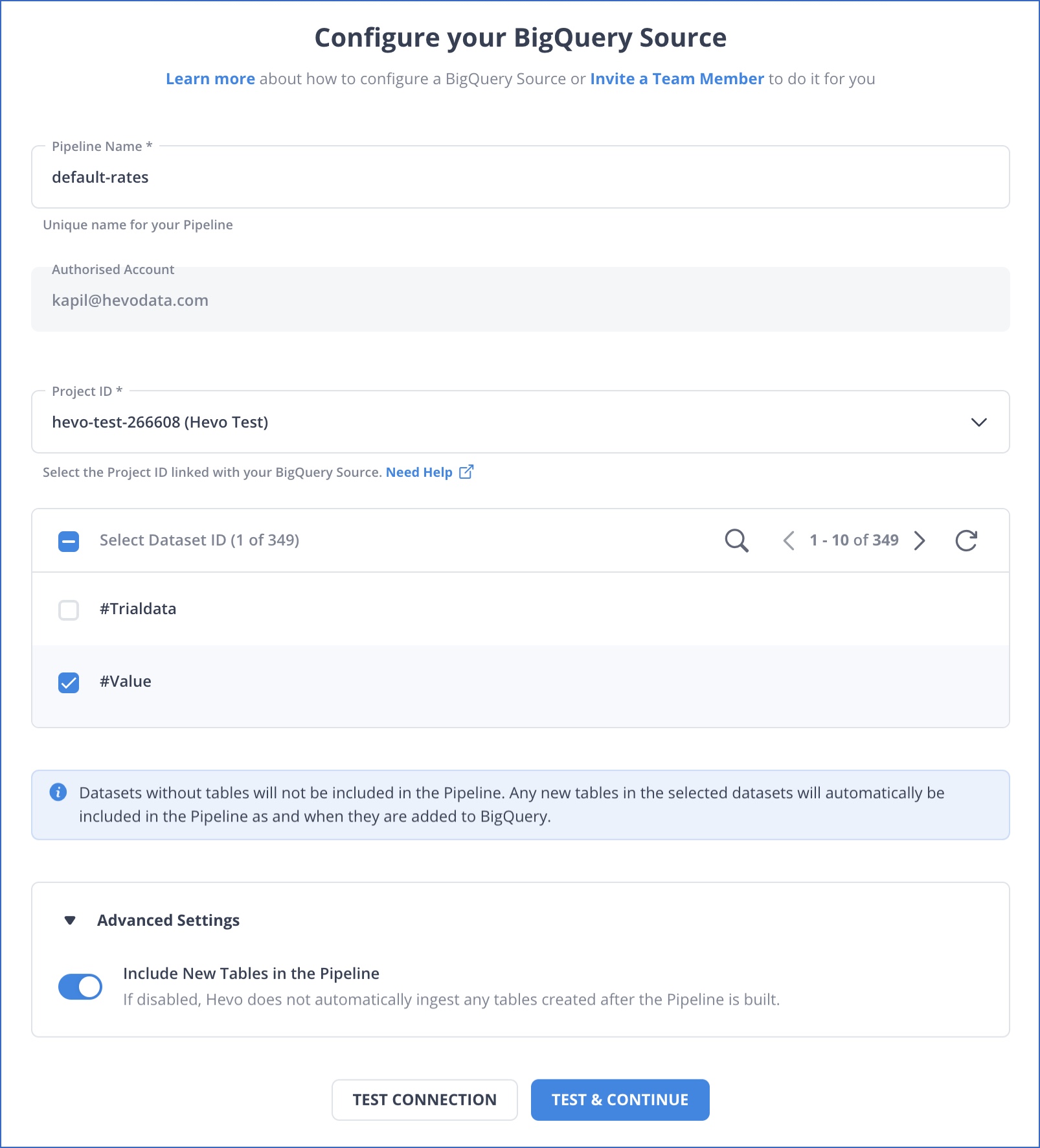
Step 2: Configure Snowflake as a Destination
Now, we will configure Snowflake as the destination.
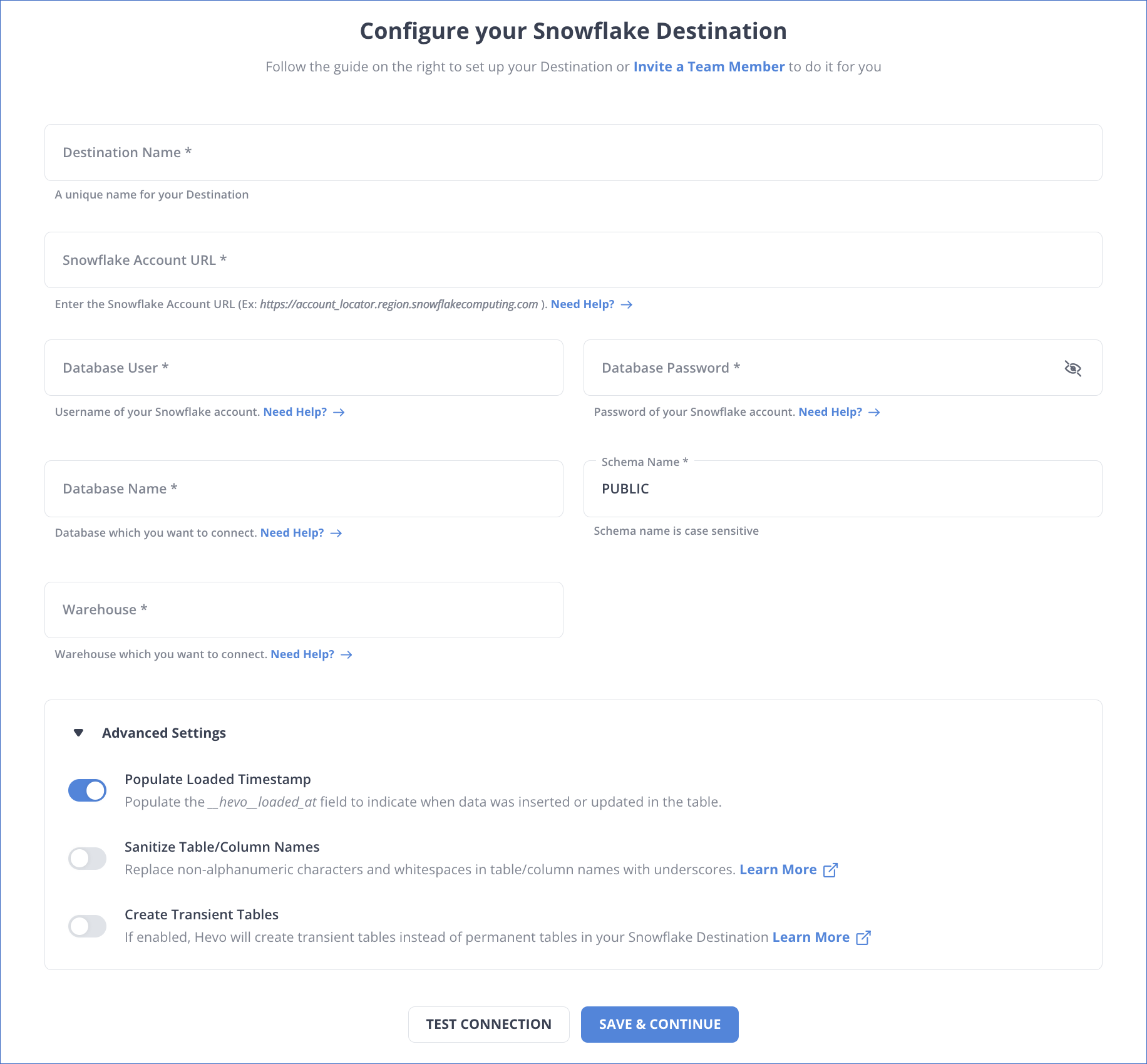
Congratulations! You’ve successfully constructed your data pipeline with BigQuery as your data source and Snowflake as your data destination.
Method 2: Connecting BigQuery to Snowflake Manually
Step 1: Move Data from BigQuery to Google Cloud Storage
We need to move the data to GCS so that we can load it to Snowflake
- Open BigQuery Console
- Export the Data using the bq tool
- Upload the Dataset into GCS Bucket
Step 2: Configure a Snowflake Storage Integration
To create a new storage integration, run the following command:
create or replace storage integration gcs_int
type = external_stage
storage_provider = 'GCS'
enabled = true
storage_allowed_locations = ('gcs://test_bucket_hevo/');

Step 3: Retrieve the cloud storage service account for our Snowflake account
To get the details of your integration, run the following command;
desc storage integration gcs_int;
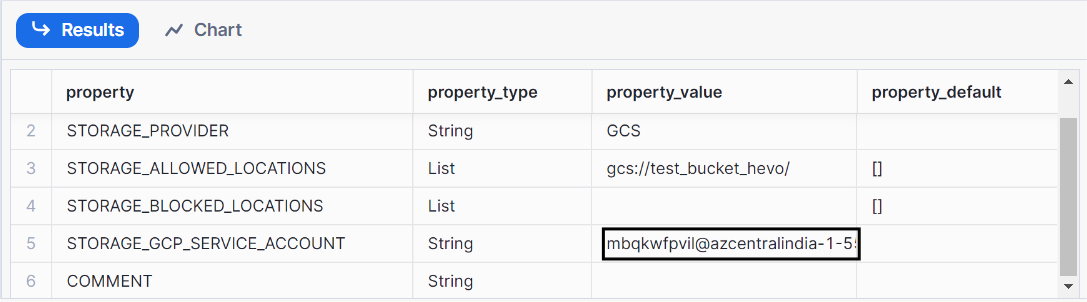
Step 4: Grant the service account permission to access the bucket object
- Go to the Google Cloud Console and navigate to IAM and Admin >> Roles
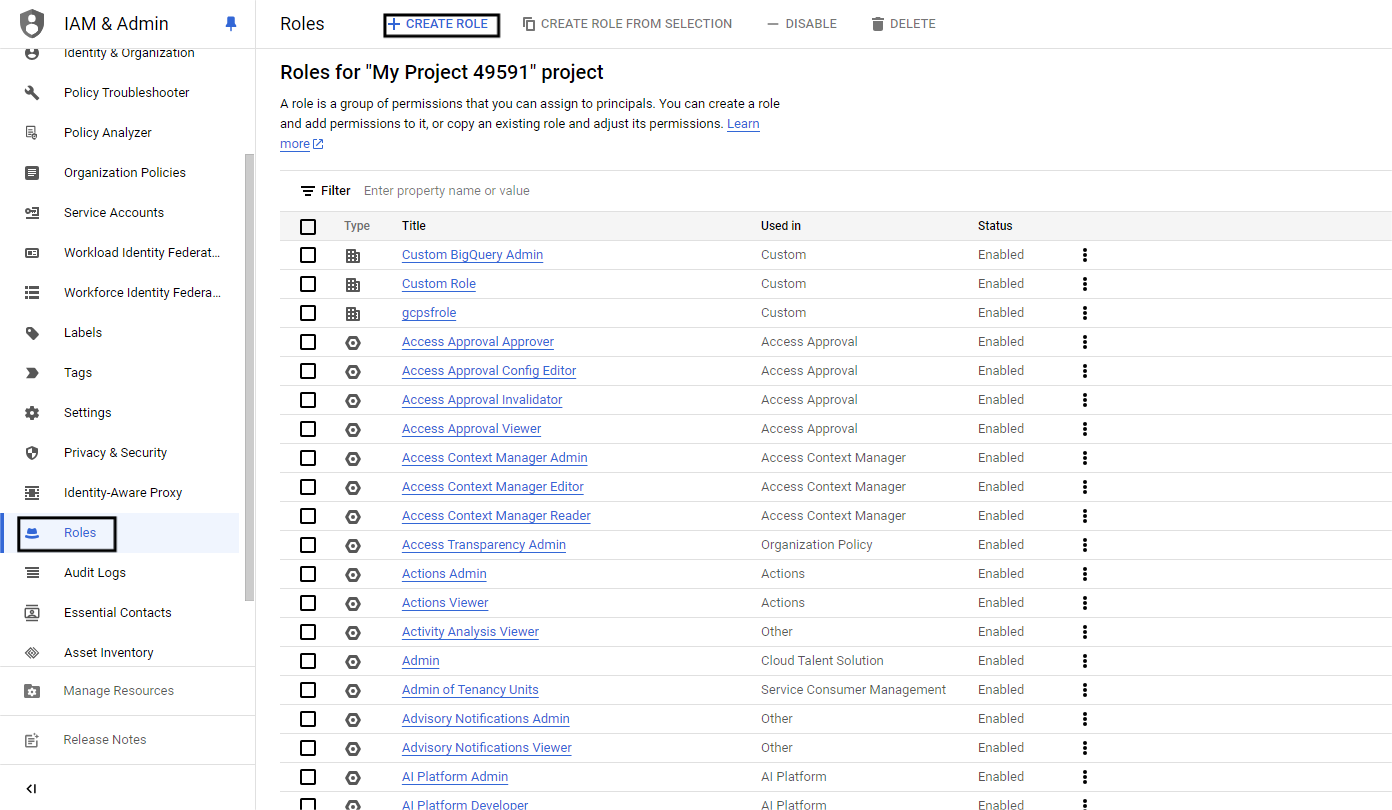
- Create a role
- Fill in the required fields and then click on Add permissions
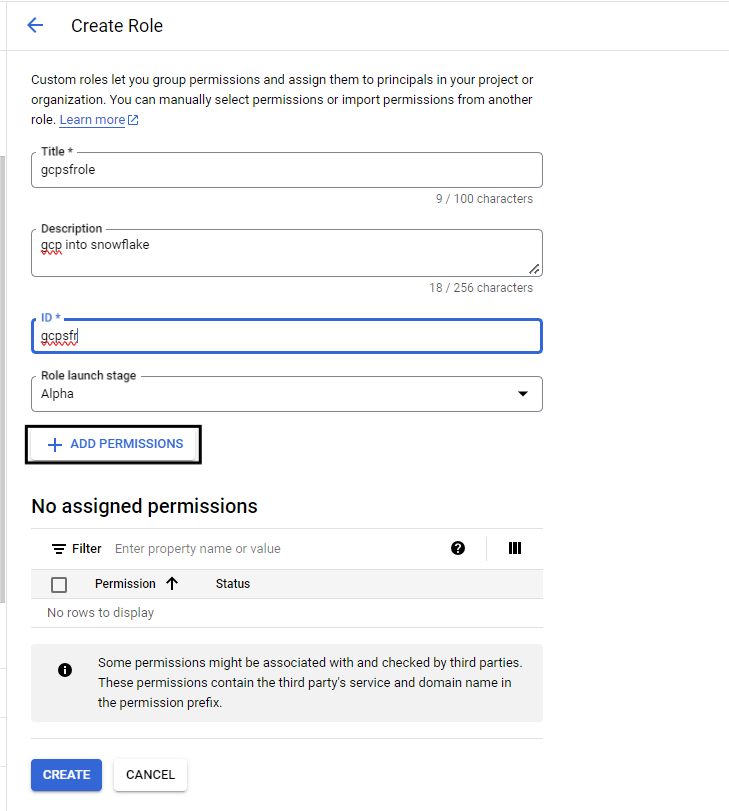
- Add these few permissions and click on Create
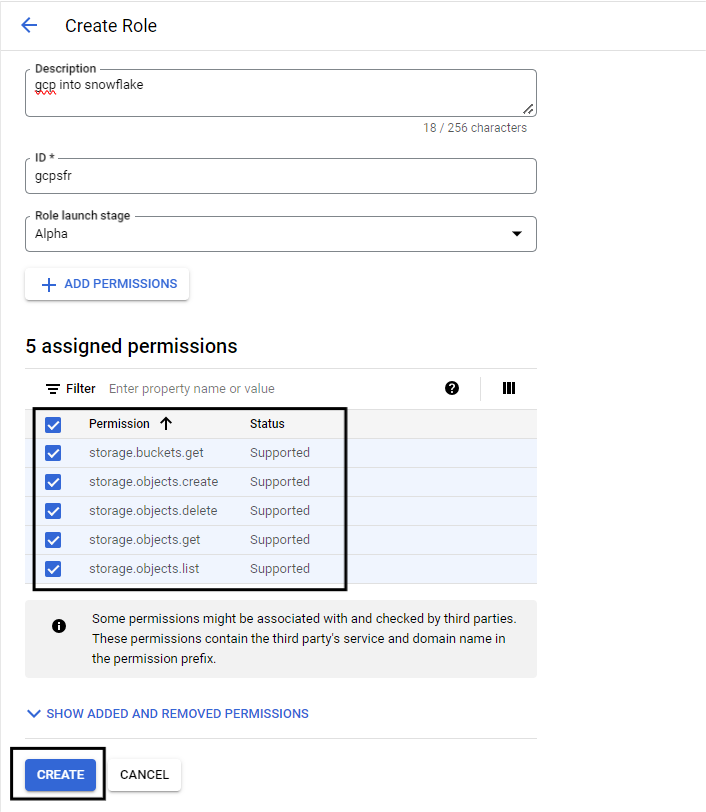
Step 5: Assign the role to the cloud storage service account
- Go back to cloud storage and select the bucket
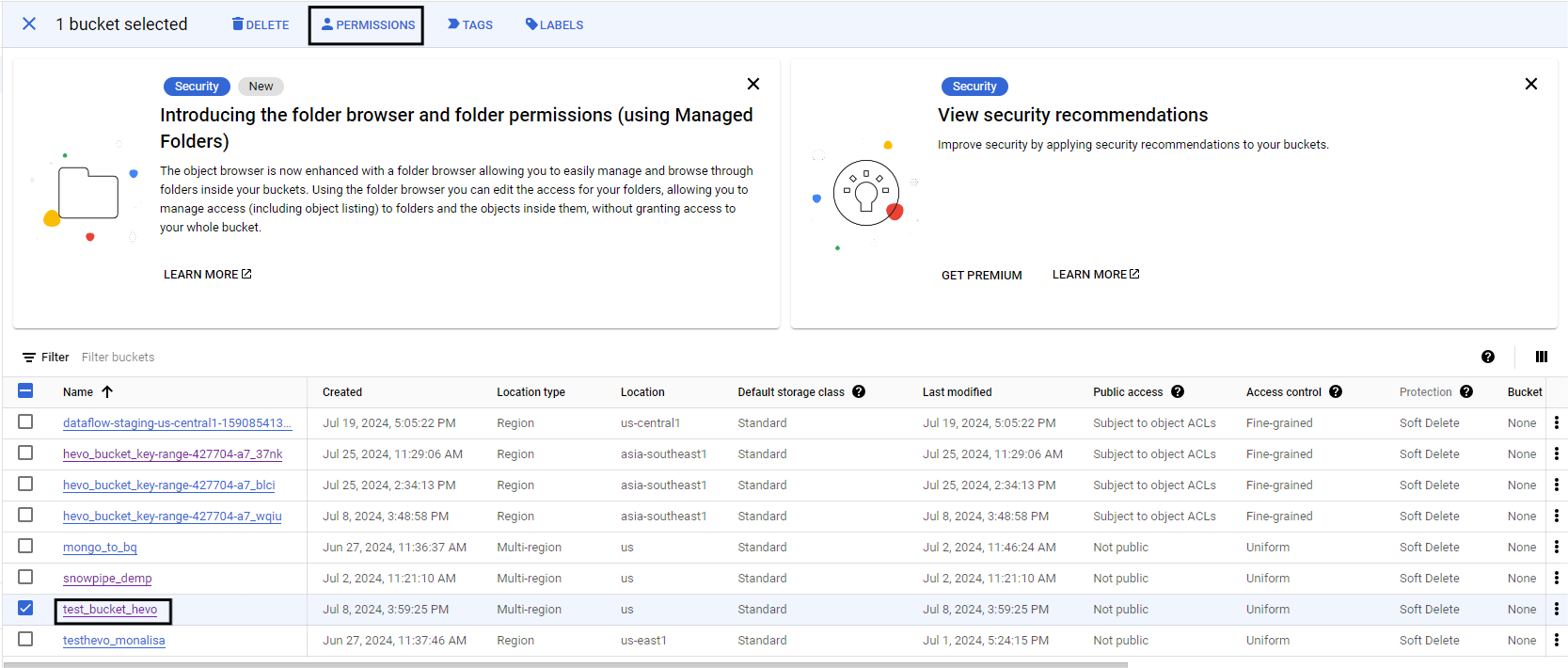
- Click on add permissions
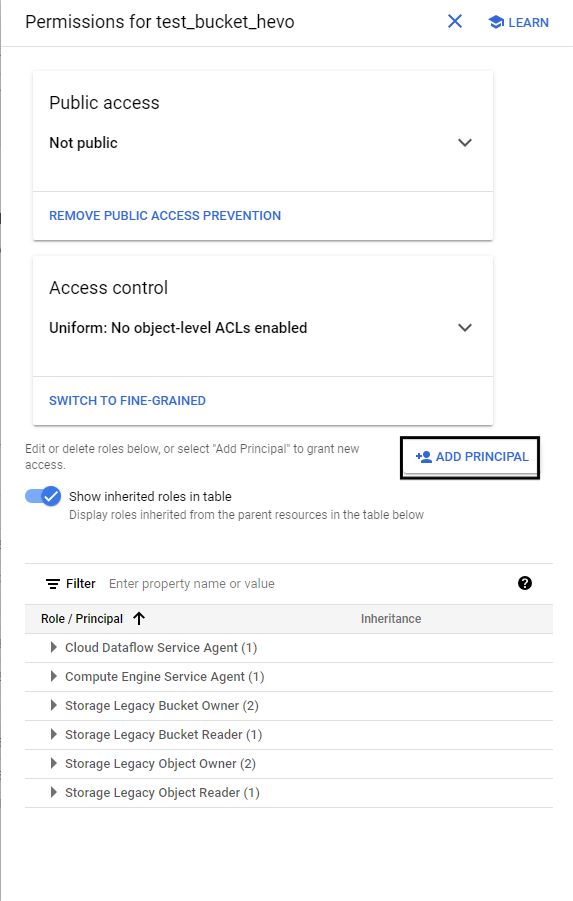
- Click on Add Principal and select the service account that you got in Snowflake
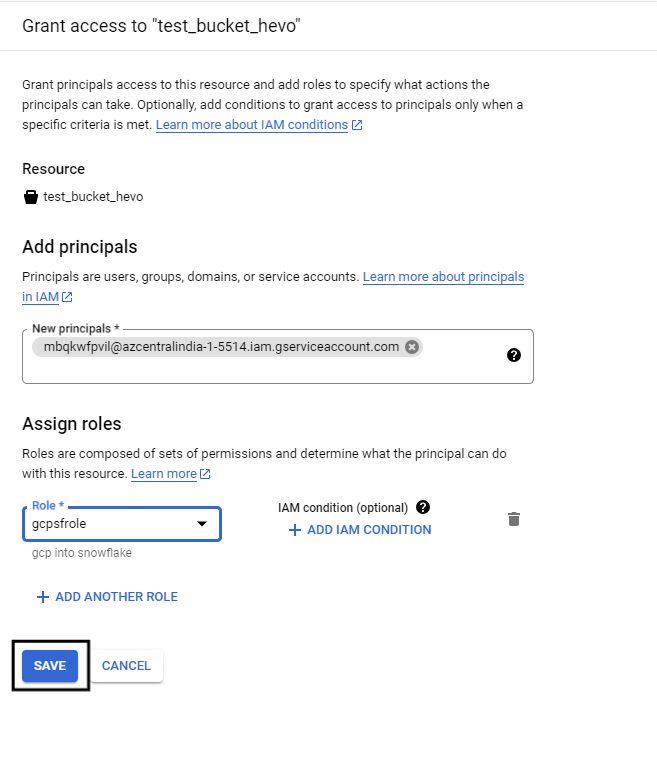
Step 6: Create an external stage
- Go back to the snowflake worksheet and create a role using the command
create role my_role;
- Next, we have to create a stage for this role that uses the command.
grant create a stage on schema public to role my_role;
- Now grant access for the integration to the role using the command.
grant usage on integration gcs_int to role myrole;
Step 7: Create a CSV file format
Use the command below to create a CSV file format to load your data from Google Cloud Storage.
Create or replace file format my_csv_format
type = csv
field_delimiter = ‘,’
skip_header = 1
Error_on_column_count_mismatch = false;
Step 8: Create a stage
Create stage my_gcs_stage
url = ‘’
Storage_integration = gcs_int
file_format = my_csv_format;
Note: U can check the details of your stage using the command
list @my_gcs_stage;
Step 9: Create a table in Snowflake
CREATE TABLE your_schema.your_table_name (
id INT,
timestamp DATETIME,
col3 INT,
col4 INT,
amount FLOAT,
status STRING,
description STRING,
bank STRING
);
Step 10: Copy data from Google Cloud Storage to Snowflake
copy into <table_name>
from @my_gcs_stage
file_format = my_csv_format
on_error = continue;
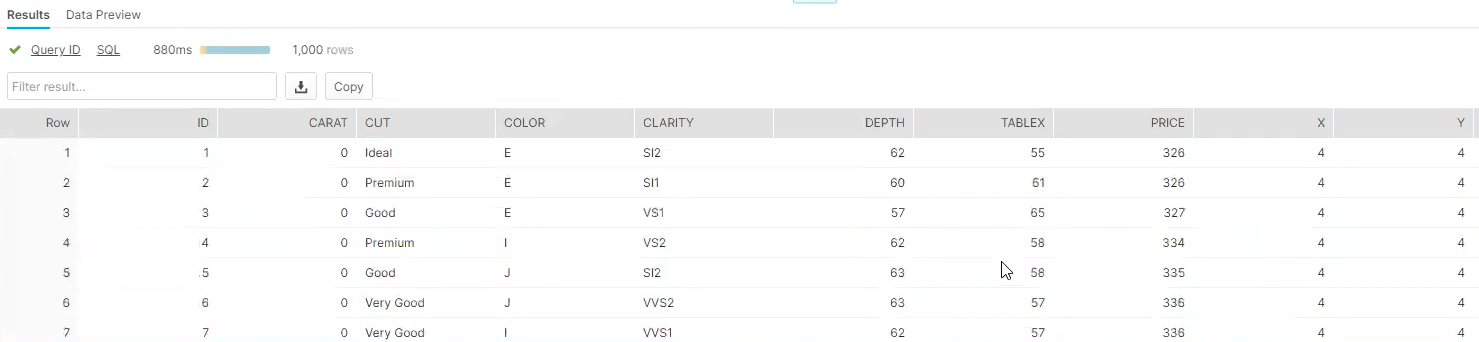
Limitations of Manually Connecting BigQuery and Snowflake
- Complex Setup: Manual connections involve multiple steps like exporting data from BigQuery, formatting it, and importing it into Snowflake, making the process time-consuming and error-prone.
- Limited Automation: Manual processes lack automation, requiring repeated efforts for recurring data transfers, which can delay operations and increase workload.
- Data Consistency Issues: Ensuring data consistency during transfers is challenging, especially for large datasets, leading to potential mismatches or incomplete data.
- Performance Bottlenecks: Exporting and importing large datasets can be slow and resource-intensive, impacting performance and potentially causing delays.
- Lack of Real-Time Sync: Manual methods do not support real-time data syncing, which is critical for time-sensitive insights and decision-making.
- High Error Risk: Manual handling increases the risk of errors like incorrect configurations, data loss, or incomplete data migrations.
- Security Concerns: Exporting and transferring data manually may expose sensitive data to vulnerabilities if not handled securely.
- Scalability Challenges: Scaling manual connections for larger datasets or frequent transfers becomes increasingly difficult and inefficient over time.
Final Thoughts
We hope we’ve helped you add value to your quest. This article explored two methods for replicating data from BigQuery to Snowflake. We used Hevo, an automated data pipeline, to obtain our desired results, making the data replication process much faster and fully automated.
Initiate your journey with Hevo today and enjoy fully automated, hassle-free data replication for 150+ sources. Hevo’s free trial gives you limitless free sources and models to pick from, support for up to 1 million events per month, and a spectacular live chat service supported by an incredible 24/7 support team to help you get started.
Sign up for a 14-day free trial and experience the feature-rich Hevo suite firsthand.
FAQs
How do you import data from BigQuery to Snowflake?
To import data from BigQuery to Snowflake, follow these general steps:
1. Export data from BigQuery
2. Transfer data to Snowflake
3. Create Stage in Snowflake
4. Load data into Snowflake
How do you migrate data from SQL to Snowflake?
To migrate data from an on-premises SQL database (like MySQL, PostgreSQL, SQL Server) to Snowflake, you can use one of the following methods:
1. Export and Load
2. ETL Tools
3. Snowflake Connector
Is Snowflake better than BigQuery?
Snowflake might be better if you need flexibility in resource scaling, cross-cloud compatibility, and robust data-sharing capabilities.
BigQuery might be better if you prefer a fully managed service with no infrastructure to manage and strongly prefer or rely on the Google Cloud ecosystem.