Snowflake provides support for standard SQL, including a subset of ANSI SQL:1999 and the SQL:2003 Analytic extensions. It also supports common variations for numerous commands where those variations do not conflict with each other. This guide will take you through the basic steps of configuring and using Snowflake SQL. So, read along to understand more about Snowflake SQL and how you can work with it.
Table of Contents
What is SQL?
Structured Query Language (SQL) is a standardized format used for querying and database management. Snowflake is a data platform and Data Warehouse that supports ANSI SQL, which is the most widely used standardized version of SQL. This implies that Snowflake SQL supports all of the most frequently used operations. Snowflake SQL also supports all of the operations that are required for Data Warehousing, such as creating, updating, and inserting.
Let’s understand the various DDL, DML, and Query syntax supported by Snowflake SQL.
Migrating to Snowflake is effortless with Hevo Data. Our platform supports Snowflake as a destination, allowing you to leverage Snowflake SQL for powerful data transformations. Simplify your migration process and take full advantage of Snowflake’s features with our user-friendly, no-code solution.
Check out why Hevo is the Best:
- Live Monitoring: Hevo allows you to monitor the data flow and check where your data is at a particular time.
- Schema Management: Hevo takes away the tedious task of schema management & automatically detects the schema of incoming data and maps it to the destination schema.
- Incremental Data Load: Hevo allows the transfer of data that has been modified in real-time. This ensures efficient utilization of bandwidth on both ends.
- Live Support: The Hevo team is available round the clock to extend exceptional customer support through chat, E-Mail, and support calls.
Snowflake SQL: DDL Commands
Snowflake objects including users, virtual warehouses, databases, schemas, tables, views, columns, functions, and stored procedures are created, manipulated, and modified using Data Definition Language (DDL) commands. They’re also used to do things like set parameters, initialize variables, and start transactions at the account and session levels.
All DDL commands are built on the foundation of the following commands:
- ALTER
- COMMENT
- CREATE
- DESCRIBE
- DROP
- SHOW
- USE
Snowflake SQL: DML Commands
DML (Data Manipulation Language) commands are used to modify and update data. You can use DML commands to insert, delete, update, and merge data in Snowflake tables using the following commands:
- INSERT
- INSERT (multi-table)
- MERGE
- UPDATE
- DELETE
- TRUNCATE TABLE
Snowflake SQL: Query Syntax
The most typical application of SQL queries is to discover particular data by filtering against predefined parameters. Data management operations can also be automated using them. For robust relational database querying, Snowflake’s Data Cloud platform has a Data Warehouse workload that supports the most prevalent standardized version of SQL (ANSI).
It can also combine semi-structured data, such as JSON, with structured data stored in SQL. Snowflake SQL makes JSON data more accessible and enables users to mix it with structured data. Users may access JSON data using SQL queries and easily link it to traditional tabular data using Snowflake.
Querying in Snowflake can be done using conventional SELECT statements and the following basic syntax:
[ WITH ... ]
SELECT
[ TOP <n> ]
...
[ FROM ...
[ AT | BEFORE ... ]
[ CHANGES ... ]
[ CONNECT BY ... ]
[ JOIN ... ]
[ MATCH_RECOGNIZE ... ]
[ PIVOT | UNPIVOT ... ]
[ VALUES ... ]
[ SAMPLE ... ] ]
[ WHERE ... ]
[ GROUP BY ...
[ HAVING ... ] ]
[ QUALIFY ... ]
[ ORDER BY ... ]
[ LIMIT ... ]
What is Snowflake SQL – SnowSQL?
- SnowSQL is the Snowflake SQL command-line client that allows you to connect to Snowflake and execute SQL queries. It also lets you perform all DDL and DML operations, including loading and unloading data from database tables.
- SnowSQL (snowsql executable) can run as an interactive shell or in batch mode. This can be done by either using stdin or using the -f option.
- SnowSQL was developed using the Snowflake Connector for Python. However, this connector is not required for installing SnowSQL. All the required software for installing SnowSQL is already bundled in the installers. SnowSQL is supported on all popular operating systems including Windows, macOS, and some distributions of Linux.
Prerequisites
- Install and configure SnowSQL.
- Your Snowflake user must have the necessary permissions to create different Snowflake objects.
How Do You Log into SnowSQL?
- Open a terminal window.
- Start SnowSQL at the command prompt using the following command:
$ snowsql -a <accountName> -u <userName>`
Here:
<accountName> is the name that has been assigned to your account by Snowflake.
<userName> is the login name assigned to your Snowflake user.
3. When you receive a prompt by SnowSQL, enter the password corresponding to your Snowflake user.
For more details, see Connecting Through SnowSQL.
How Do You Create Snowflake Objects?
- In Snowflake, it is necessary to have a database and table before you can load your data. This article loads data into a table in a database called demo.
- Additionally, loading and querying of data require a virtual warehouse. This provides the required compute resources to perform these tasks.
1. Create a Database
The database can be created using the following syntax:
CREATE OR REPLACE DATABASE demo;
Snowflake assigns a default schema named public to every database created and thus, it is not necessary to create a schema on your own. The database and schema that you just created are now being used for your current session. This information is displayed in your SnowSQL command prompt, but you can also view it by using the following command:
SELECT CURRENT_DATABASE(), CURRENT_SCHEMA();
2. Creating a Table
Create a table named emp_details in demo.public using the CREATE TABLE command:
CREATE OR REPLACE TABLE emp_details (
first_name STRING,
last_name STRING,
email STRING,
address STRING,
city STRING,
start_date DATE
);
Note that the number of columns in the table, their positions, and their data types correspond to the fields in the CSV data files that you will be staging in the next step.
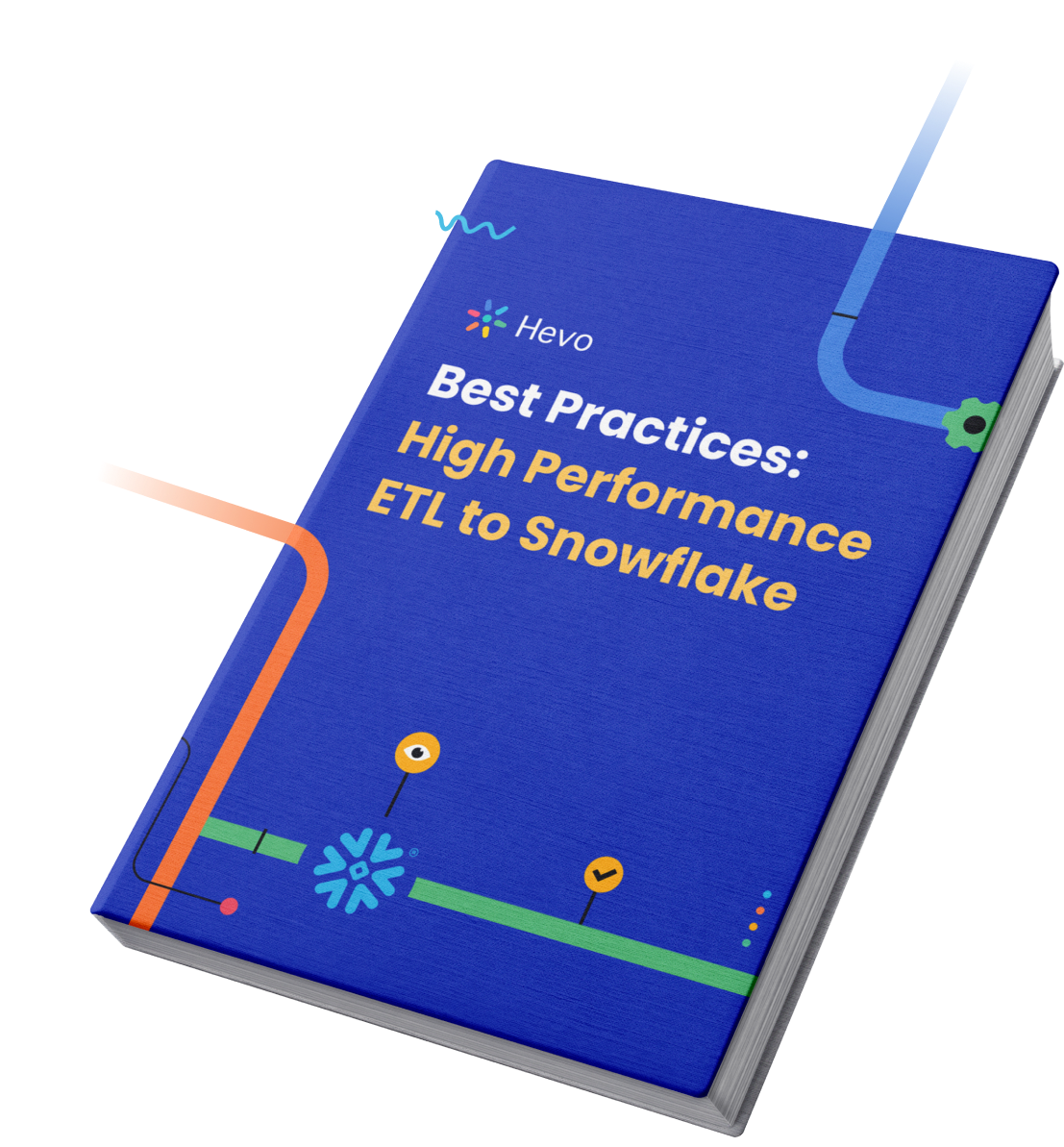
3. Creating a Virtual Warehouse
Create an X-Small warehouse named demo_wh using the following syntax:
CREATE OR REPLACE WAREHOUSE demo_wh WITH
WAREHOUSE_SIZE='X-SMALL'
AUTO_SUSPEND = 180
AUTO_RESUME = TRUE
INITIALLY_SUSPENDED=TRUE;
Here,
WAREHOUSE_SIZE: Specifies the number of servers in each cluster. Learn more about the different sizes available.
AUTO_SUSPEND and AUTO_RESUME: It will suspend the warehouse if it is inactive for the specified period. Auto-resume has enabled automatically when a query is submitted that requires computing resources from the warehouse and the warehouse is in the current session.
INITIALLY_SUSPENDED = TRUE | FALSE, specifies whether the warehouse is created initially in the ‘Suspended’ state.
It should be noted that the warehouse will not be started initially, but is set to auto-resume, what it means is that, it will automatically start running when you execute your first SQL command that requires its compute resources.
Explore other parameters and allowed values for creating a warehouse.
Also, note that the warehouse you created is now being used for your current session. This information will be displayed in your SnowSQL command prompt but can also be viewed using the following command:
SELECT CURRENT_WAREHOUSE();
How Do You Stage Data Files in Snowflake?
Snowflake lets you load data from files that have been not only staged in an internal (Snowflake) stage but also in an external (Amazon S3, Google Cloud Storage, or Microsoft Azure) stage. Loading from any external stage is very useful when you already have data files stored in these cloud storage services.
The <strong>PUT</strong>
command is used to stage files.
Execute PUT to upload local data files to the table stage provided for the emp_details table you previously created. Note that this command is OS-specific because it references files in your local environment. The path thus needs to be set accordingly.
Linux or macOS:
PUT file:///tmp/employees0*.csv @demo.public.%emp_details;
Windows:
PUT file://C:tempemployees0*.csv @demo.public.%emp_details;
It is important to note that the PUT command compresses files by default using gzip.
You can see the list of the files you successfully staged by executing a <strong>LIST</strong>
command:
LIST @demo.public.%emp_details;
How Do You Copy Data into the Target Table in Snowflake?
You can now easily load your staged data into the target table using the COPY INTO <table> command. Another important thing to keep in mind is that this command requires an active and running warehouse.
COPY INTO emp_details
FROM @%emp_details
FILE_FORMAT = (type = csv field_optionally_enclosed_by='"')
PATTERN = '.*employees0[1-5].csv.gz'
ON_ERROR = 'skip_file';
Let’s look more closely at this command:
- The FROM clause is used to identify the internal stage location.
- FILE_FORMAT is used to specify the type of the file. In this example, it specifies the file type as CSV and the double-quote character (“) as the character used to enclose strings. Snowflake supports a variety of file types and options.
- PATTERN is used for pattern matching to pull data from all files that match the regular expression .*employees0[1-5].csv.gz.
- ON_ERROR specifies what to do when the COPY command incurs any errors in the files. By default, the command stops loading data when the first error is caught. However, we have edited the query to skip any file containing an error and move on to loading the next file.
Moreover, The COPY command helps in validating files before loading. You can go through the COPY INTO <table> topic for additional error checking and validation methods.
How Do You Query the Loaded Data in Snowflake?
The data loaded in the emp_details table can be queried easily using the standard Snowflake SQL statements, supporting functions, and operators.
Moreover, the data can also be manipulated, like updating the loaded data or inserting more data, using standard DML commands.
- To return all rows and columns from the table:
SELECT * FROM emp_details;
- To insert rows directly into a table:
E.g. to insert two additional rows into the table:
INSERT INTO emp_details VALUES
('Clementine','Adamou','cadamou@sf_tuts.com','10510 Sachs Road','Klenak','2017-9-22') ,
('Marlowe','De Anesy','madamouc@sf_tuts.co.uk','36768 Northfield Plaza','Fangshan','2017-1-26');
- For pattern matching, you can use the LIKE function.
E.g. To display records having email addresses with a UK domain name:
SELECT email FROM emp_details WHERE email LIKE '%.uk';
Learn more about the complete reference manual for Snowflake SQL commands supported by Snowflake.
Use Snowflake’s TRUNCATE TABLE command to clear data efficiently without dropping the table. Find out more at TRUNCATE Table in Snowflake.
Conclusion
- You have now learned the basics of using Snowflake SQL. SnowSQL can be used to load, access, and manipulate data.
- You can also transfer data from MySQL Server to Snowflake and perform various ETL operations. Learn how you can transfer data from MySQL Server to Snowflake as well.
- Follow a step-by-step guide to installing Snowflake on Linux for a seamless setup experience. Find more information at Install Snowflake on Linux.
- Not comfortable with using Snowflake SQL queries? Want an easier way to load data? This is where a simpler alternative like Hevo can save your day!
Hevo Data is a No-Code Data Pipeline that offers a faster way to move data from 150+ Data Sources including 60+ Free Sources, into your Data Warehouse to be visualized in a BI tool. Hevo is fully automated and, hence, does not require you to code.
Take Hevo’s 14-day free trial to experience a better way to manage your data pipelines. You can also check out the unbeatable pricing, which will help you choose the right plan for your business needs.
Frequently Asked Questions
1. Is Snowflake SQL different than SQL?
Yes, Snowflake SQL is based on standard SQL but includes some unique features and functions specific to Snowflake’s cloud data platform.
2. Does Snowflake use SQL or MySQL?
Snowflake uses SQL, not MySQL. It utilizes its own variant of SQL tailored for cloud-based data warehousing and analytics.
3. What language is Snowflake SQL?
Snowflake uses its own variant of SQL called SnowSQL, which is based on standard SQL with added Snowflake-specific functionalities.