Is your data in Google Sheets becoming too large for on-demand analytics? Are you struggling to combine data from multiple Google Sheets into a single source of truth for reports and analytics? If that’s the case, then your business may be ready for a move to a mature data platform like Snowflake.
This post covers three approaches for migrating your data from Google Sheets to Snowflake. Snowflake Google Sheets integration facilitates data accessibility and collaboration by allowing information to be transferred and analyzed across the two platforms with ease. The following are the methods you can use to connect Google Sheets to Snowflake in a seamless fashion:
Table of Contents
Need for Migration From Google Sheets to Snowflake
This section will give you a better idea of why you need to migrate your data from Google Sheets to Snowflake.
- Snowflake deals very easily with huge volumes of data, while Google Sheets slows down performance when the size of the data is large. Therefore, if you are dealing with larger datasets, migrating them to Snowflake will be a better option.
- Snowflake has very strong analytics capabilities for running complex queries and deriving deeper data insights than Google Sheets can.
- Security capabilities, versioning control, and collaboration are enhanced within Snowflake’s cloud-based platform compared to Google Sheets, which offers more limited options for sharing and security.
Manually exporting spreadsheets and uploading them to Snowflake? There’s a better way. Hevo’s no-code platform lets you automate the entire flow from Google Sheets to Snowflake quickly and reliably.
With Hevo:
- Connect Google Sheets to Snowflake with just a few clicks
- Schedule automatic syncs to keep your warehouse up to date
- Handle schema changes and data formatting with ease
Trusted by 2000+ data professionals at companies like Playtomic and Harmoney. Say goodbye to manual uploads and start syncing your Google Sheets to Snowflake with Hevo today!
Get Started with Hevo for FreeMethods to Connect Google Sheets to Snowflake
Here, I have demonstrated three methods by which you can successfully connect Google Sheets to Snowflake.
Method 1: Using Hevo Data to Connect Google Sheets to Snowflake
Hevo provides an easy-to-use data integration platform that works by building an automated pipeline in just two interactive steps:
Step 1: Configure Google Sheets as a source by entering the details as shown in the image.
Step 2: Create and Configure your Snowflake Warehouse
It just took us 2 weeks to completely transform from spreadsheets to a modern data stack. Thanks to Hevo that helped us make this transition so smooth and quick. Now all the stakeholders of our management, sales, and marketing team can easily build and access their reports in just a few clicks.
– Matthew Larner, Managing Director, ClickSend
Method 2: Using Migration Scripts to Connect Google Sheets to Snowflake
To migrate your data from Google Sheets to Snowflake, you may opt for a custom-built data migration script to get the job done. We will demonstrate this process in the next paragraphs. To proceed, you will need the following requirements.
Step 1: Setting Up Google Sheets API Access for Google Sheets
As a first step, you would need to set up Google Sheets API access for the affected Google Sheets. Start by doing the following:
1. Log in to the Google account that owns the Google Sheets
2. Point your browser to the Google Developer Console (copy and paste the following in your browser: console.developers.google.com)
3. After the console loads create a project by clicking the “Projects” dropdown and then clicking “New Project“
4. Give your project a name and click “Create“
5. After that, click “Enable APIs and Services“
6. Search for “Google Sheets API” in the search bar that appears and select it
7. Click “Enable” to enable the Google Sheets API
8. Click on the “Credentials” option on the left navbar in the view that appears, then click “Create Credentials“, and finally select “Service Account“
9. Provide a name for your service account. You will notice it generates an email format for the Service Account ID. In my example in the screenshot below, it is “gsheets-migration@migrate-268012.iam.gserviceaccount.com”. Take note of this value. The token “migrate-268012” is the name of the project I created while “gsheets-migration” is the name of my service account. In your case, these would be your own supplied values.
10. Click “Create” and fill out the remaining optional fields. Then click “Continue“
11. In the view that appears, click “Create Key“, select the “JSON” option and click “Create” to download your key file (credentials). Please store it in a safe place. We will use this later when setting up our migration environment.
12. Finally, click “Done“.
At this point, all that remains for the Google Sheets setup is the sharing of all the Google Sheets you wish to migrate with the email-format Service Account ID mentioned in step 9 above.
Note: You can copy your Service Account ID from the “client-email” field of the credential file you downloaded.
For this demonstration, I will be migrating a sheet called “data-uplink-logs” shown in the screenshot below. I will now share it with my Service Account ID:
Click “Share” on the Google sheet, paste in your Service Account ID, and click “Send“. Repeat this process for all sheets you want to migrate. Ignore any “mail delivery subsystem failure” notifications you receive while sharing the sheets, as your Service Account ID is not designed to operate as a normal email address.
Step 2: Configuring Target Database in Snowflake
We’re now ready to get started on the Snowflake side of the configuration process, which is simpler.
To begin, create a Snowflake account. Creating an account furnishes you with all the credentials you will need to access Snowflake from your migration script.
Specifically:
- After creating your account, you will be redirected to your Cloud Console which will open up in your browser
- During the account creation process, you would have specified your chosen username and password. You would have also selected your preferred AWS region, which will be part of your account.
- Your Snowflake account is of the form <Your Account ID>.<AWS Region> and your Snowflake cloud console URL will be of the form https://<Your Account ID>.<AWS Region>.snowflakecomputing.com/
- Prepare and store a JSON file with these credentials. It will have the following layout:
{ “user”: “<Your Username>”, “account”: “<Your Account ID>.<AWS Region>”, “password”: “<Your Password>” }
After storing the JSON file, take some time to create your target environment on Snowflake using the intuitive User Interface.
You are initially assigned a Data Warehouse called COMPUTE_WH so you can go ahead and create a Database and tables in it.
After providing a valid name for your database and clicking “Finish“, click the “Grant Privileges” button which will show the form in the screenshot below.
Select the “Modify” privilege and assign it to your schema name (which is “PUBLIC” by default). Click “Grant“. Click “Cancel” if necessary, and then return to the main view.
The next step is to add a table to your newly created database. You do this by clicking the database name on the left display and then clicking on the “Create Table” button. This will pop up the form below for you to design your table:
After designing your table, click “Finish” and then click on your table name to verify that your table was created as desired:
Finally, open up a Worksheet pane, which will allow you to run queries on your table. Do this by clicking on the “Worksheets” icon, and then clicking on the “+” tab.
You can now select your database from the left pane to start running queries.
We will run queries from this view to verify that our data migration process is correctly writing our data from the Google sheet to this table.
We are now ready to move on to the next step.
Step 3: Preparing a Migration Environment on Linux Server
In this step, we will configure a migration environment on our Linux server.
- SSH into your Linux instance. I am using a remote AWS EC2 instance running Ubuntu, so my SSH command is of the form
ssh -i <keyfile>.pem ubuntu@<server_public_IP>
- Once in your instance, run sudo apt-get update to update the environment
- Next, create a folder for the migration project and enter it
sudo mkdir migration-test; cd migration-test
- It’s now time to clone the migration script we created for this post:
sudo git clone https://github.com/cmdimkpa/Google-Sheets-to-Snowflake-Data-Migration.git
- Enter the project directory and view contents with the command:
cd Google-Sheets-to-Snowflake-Data-Migration; ls
This reveals the following files:
- googlesheets.json: copy your saved Google Sheets API credentials into this file.
- snowflake.json: likewise, copy your saved Snowflake credentials into this file.
- migrate.py: this is the migration script.
Using the Migration Script
Before using the migration script (a Python script), we must ensure the required libraries for both Google Sheets and Snowflake are available in the migration environment. Python itself should already be installed – this is usually the case for Linux servers, but check and ensure it is installed before proceeding.
To install the required packages, run the following commands:
sudo apt-get install -y libssl-dev libffi-dev
pip install --upgrade snowflake-connector-python
pip install gspread oauth2client PyOpenSSL
At this point, we are ready to run the migration script.
The required command is of the form:
sudo python migrate.py <Source Google Sheet Name>
<Comma-separated list of columns in the Google Sheet to Copy>
<Number of rows to copy each run> <Snowflake target Data Warehouse>
<Snowflake target Database> <Snowflake target Table> <Snowflake target table Schema>
<Comma-separated list of Snowflake target table fields> <Snowflake account role>
For our example process, the command becomes:
sudo python migrate.py data-uplink-logs A,B,C,D 24
COMPUTE_WH TEST_GSHEETS_MIGRATION GSHEETS_MIGRATION PUBLIC CLIENT_ID,NETWORK_TYPE,BYTES,UNIX_TIMESTAMP SYSADMIN
To migrate 24 rows of incremental data (each run) from our test Google Sheet data-uplink-logs to our target Snowflake environment, we simply run the command above. The following is a screenshot of what follows:
The reason we migrate only 24 rows at a time is to beat the rate limit for the free tier of the Google Sheets API. Depending on your plan, you may not have this restriction.
Step 4: Testing the Migration Process
To test that the migration ran successfully, we simply go to our Snowflake Worksheet which we opened earlier, and run the following SQL query:
SELECT * FROM TEST_GSHEETS_MIGRATION.PUBLIC.GSHEETS_MIGRATION
Indeed, the data is there. So the data migration effort was successful.
Step 5: Run CRON Jobs
As a final step, run cron jobs as required to have the migrations occur on a schedule. We cannot cover the creation of cron jobs here, as it is beyond the scope of this post.
This concludes the first approach! I hope you were as excited reading that as I was, writing it. It’s been an interesting journey, now let’s review the drawbacks of this approach.
Limitations of using Migration Scripts to Connect Google Sheets to Snowflake
The migration script approach to connect google sheets to Snowflake works well, but has the following drawbacks:
- This approach would need to pull out a few engineers to set up and test this infrastructure. Once built, you would also need to have a dedicated engineering team that can constantly monitor the infra and provide immediate support if and when something breaks.
- Aside from the setup process which can be intricate depending on experience, this approach creates new requirements such as:
- The need to monitor the logs and ensure the uptime of the migration processes.
- Fine-tuning of the cron jobs to ensure optimal data transmission with respect to the data inflow rates of the different Google sheets, any Google Sheet API rate limits, and the latency requirements of the reporting or analytics processes running on Snowflake or elsewhere.
Method 3: Connect Google Sheets to Snowflake Using Python
In this method, you will use Python to load data from Google Sheets to Snowflake. To do this, you will have to enable public access to your Google Sheets. You can do this by going to File>> Share >> Publish to web.
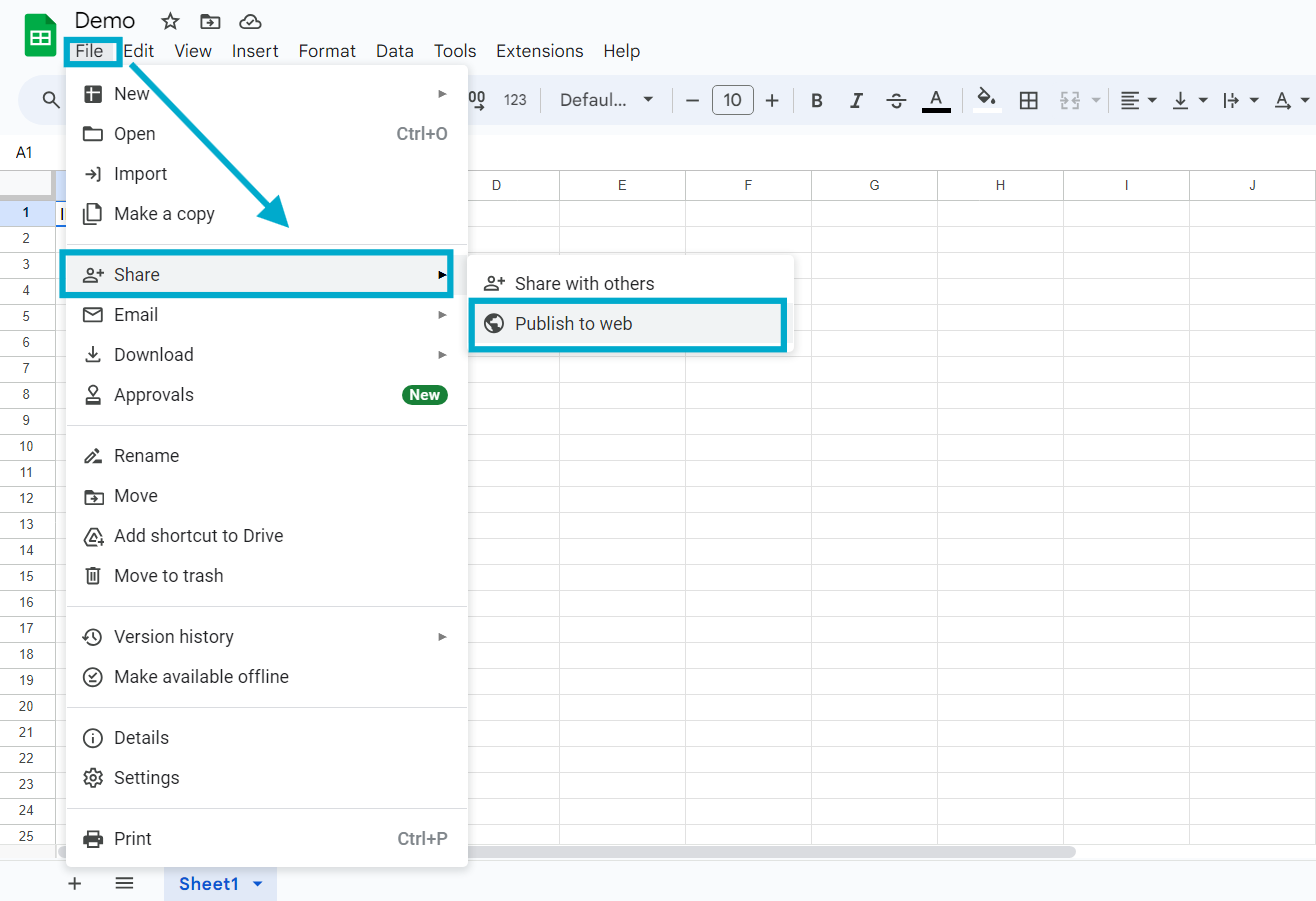
After publishing to web, you will see a link in the format of
https://docs.google.com/spreadsheets/d/{your_google_sheets_id}/edit#gid=0
You would need to install certain libraries in order to read this data, transform it into a dataframe, and write to Snowflake. Snowflake.connector and Pyarrow are the other two, while Pandas is the first.
Installing pandas may be done with pip install pandas. The command pip install snowflake-connector-python may also be used to install Snowflake connector. The command pip install pyarrow may be used to install Pyarrow.
You may use the following code to read the data from your Google Sheets.
import pandas as pd
data=pd.read_csv(f'https://docs.google.com/spreadsheets/d/{your_google_sheets_id}/pub?output=csv')
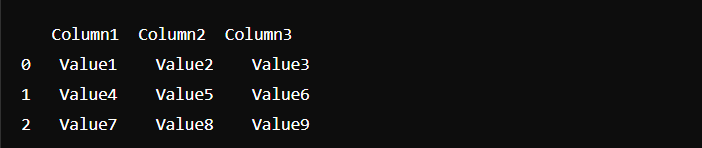
In the code above, you will replace {your_google_sheets_id} with the id from your spreadsheet. You can preview the data by running the command data.head()
# Error 1: HTTPError or URLError if the URL is incorrect or if there’s an issue with internet connectivity.
# Error 2: ParserError if the CSV data is not formatted correctly.
You can also check out the number of columns and records by running data.shape
Setting up Snowflake login credentials
You will need to set up a data warehouse, database, schema, and table on your Snowflake account.
Data loading in Snowflake
You would need to utilize the Snowflake connection that was previously installed in Python in order to import the data into Snowflake.
When you run write_to_snowflake(data), you will ingest all the data into your Snowflake data warehouse.
Disadvantages Of Using ETL Scripts
There are a variety of challenges and drawbacks when integrating data from sources like Google Sheets to Snowflake using ETL (Extract, Transform, Load) procedures, especially for businesses with little funding or experience.
- Price is the primary factor to be considered. Implementation and upkeep of the ETL technique can be expensive. It demands investments in personnel with the necessary skills to efficiently design, develop, and oversee these processes in addition to technology.
- Complexity is an additional problem. ETL processes may be intricate and challenging to configure properly. Companies without the necessary expertise may find it difficult to properly manage data conversions and interfaces. This is where businesses often choose to hire JavaScript developers to streamline the integration process and handle complex data transformations effectively.
- ETL processes can have limitations on scalability and flexibility. They might not be able to handle unstructured data well or provide real-time data streams, which makes them inappropriate.
Learn More About:
How to Connect Google Search Console to Snowflake
Conclusion
In this blog, we explored three key methods to migrate data from Google Sheets to BigQuery: using migration scripts, Python, and Hevo. While migration scripts offer flexibility, they demand extensive coding and come with challenges in error handling. Python scripts help automate the process but require strong programming skills and ongoing maintenance.
Hevo stands out as a no-code, fully automated solution that simplifies ETL and ensures real-time data sync without the need for custom scripts or manual intervention. With built-in error handling and seamless connectivity, Hevo makes your data migration efficient, reliable, and hassle-free.
Ready to simplify your Google Sheets to BigQuery data flow? Try Hevo for free and start streaming data in minutes.
FAQs to migrate from Google Sheets to Snowflake
1. Can I connect Google Sheets to Snowflake?
Yes, you can connect Google Sheets to Snowflake using various methods such as data integration tools like Hevo or custom scripts.
2. How do I push data into Snowflake?
To push data into Snowflake, you can use the COPY INTO command to load data from files stored in cloud storage (like AWS S3 or Google Cloud Storage) into Snowflake tables.
3. Is Snowflake just SQL?
No, Snowflake is not just SQL. While it supports SQL for querying and managing data, Snowflake is a cloud-based data warehousing platform designed for scalable data storage, processing, and analysis.