Data is the new fuel. Each and every industry is currently dependent on data for its sustenance. And it is very important the data that is being utilized should be clean, precise, and without any errors. Because any error in the data pipeline can lead to gaps in decisions leading to heavy losses in revenues. This is where the concept of Observability comes into the picture.
It is the process of diagnosing the health of the entire data value chain by viewing its outputs in order to proactively find and mitigate the issues.
You will gain a holistic understanding of its importance, the five pillars of this approach, and the comparison between Data Observability and Data Monitoring. You will also learn about the top 6 data observation tools and their key features.
Table of Contents
What is Data Observability?
With the rise of data downtime and the increasing complexity of the data stack, observability has emerged as a critical concern for data teams. But what exactly is it?
“Data Observability” refers to the ability to comprehend the health and state of data in your system. It is an umbrella term that includes a consolidated set of workflows and technologies that enable you to discover, debug, and fix data issues in near real-time. It not only detects inaccuracies in your data but also assists you in determining the primary cause of the problems and suggests proactive measures to make your systems efficient and reliable.
Observability pipelines are highly available for engineers since they encompass a wide range of activities. Unlike the data quality standards and technologies associated with the concept of a data warehouse, observability goes much beyond simply defining the problem. It offers enough background for the engineers to devise remedial measures for mitigating the issues and initiate dialogues to avoid making the same mistake again.
With Hevo’s robust data pipeline solutions, organizations can achieve high data quality standards effortlessly. Try Hevo and equip your team to:
- Integrate data from 150+ sources(60+ free sources).
- Utilize drag-and-drop and custom Python script features to transform your data.
- Risk management and security framework for cloud-based systems with SOC2 Compliance.
Try Hevo and discover why 2000+ customers have chosen Hevo to upgrade to a modern data stack.
Get Started with Hevo for FreeWhy is Data Observability Important?
Data observability extends beyond monitoring and alerting to provide businesses with a complete understanding of their data systems, allowing them to repair data problems in more complex data situations or even prevent them from occurring in the first place. See how it compares and contrasts with data quality.
1) Enhances trust in data
Although data insights and machine learning algorithms can be extremely beneficial, erroneous and unmanaged data can have disastrous repercussions.
Here is where Data Observability comes into play. It enables enterprises to become more confident when making data-driven choices by quickly monitoring and tracking situations.
2) Timely delivery of quality data for business workloads
Every company must ensure that data is easily accessible and in the proper format. Data engineers, data scientists, and data analysts all rely on excellent data to carry out their business operations. A lack of precise quality data might lead to a costly breakdown in business processes.
Data observability maintains the quality, dependability, and consistency of data in the data pipeline by providing companies with a 360-degree perspective of their data ecosystem, allowing them to drill down and rectify issues that might lead to a breakdown in your data pipeline.
3) Discover and resolve data issues before they affect the business
Data observability recognizes circumstances you aren’t aware of or wouldn’t think to search for, allowing you to avert problems before they have a severe impact on your organization. This can give context and relevant information for root cause analysis and remediation by tracking linkages to particular issues.
What are the 5 Pillars of Data Observability?
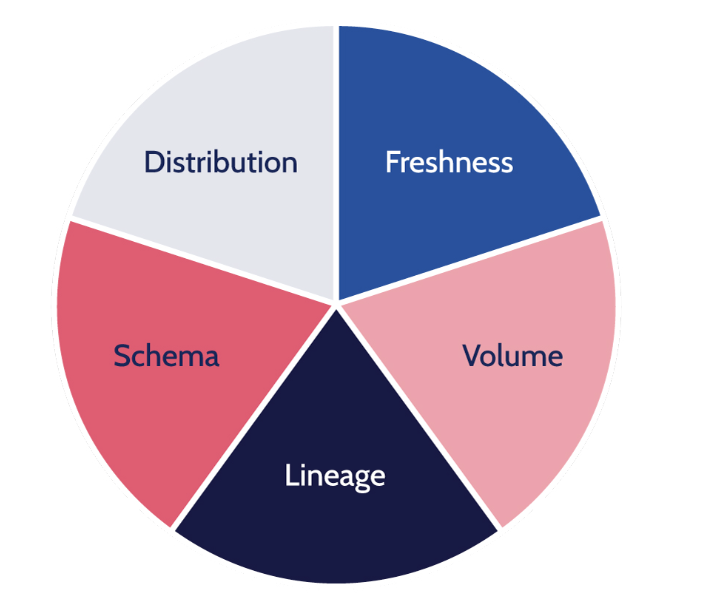
There are five key pillars of Data Observability that represent the health of data. Those are as follows:
1) Freshness
Freshness basically revolves around whether the data is up-to-date, i.e., whether the data has accommodated all the changes with data without any gaps in time. If there is a freshness issue, then this can break data pipelines, resulting in multiple errors and gaps in the data. For example, in case of a data downtime incident, suppose there is a view of a table that gets updated on a regular basis, but in between, there is a large gap of time when it is not getting updated then this is the case of a freshness issue.
2) Distribution
Distribution focuses on determining the field-level health of your data i.e if the data is within an accepted range. It checks whether there is a gap between the expected value of data and the actual value of data. There will be a distribution issue when there will be an abnormal representation of values of fields or the null rate percentage will be significantly different for any particular field.
3) Volume
Volume simply refers to the amount of data in a file or database. This indicates whether the amount of data being fed i.e, the data intake meets the expected thresholds. It helps in providing insights into the health of your data sources. Suppose, you observe a significant change in the volume of data coming from a source on two different dates, there is some anomaly in the data source.
4) Schema
Schema refers to the structure of the data supported by a database management system. The schema of the tables in a database should always be according to the way the DBMS complies to. However, sometimes due to changes in the data, modifications, removal, or if the data isn’t fed properly then errors might occur in the schema of the database. This can result in severe data downtime. Regular auditing of your schema ensures the good health of your data.
5) Lineage
Data lineage indicates which upstream sources and downstream consumers were affected when the pipeline broke, as well as whose teams generated the data and who accessed it. Good lineage also gathers data-related information, i.e., metadata that describes the governance, business, and technical principles connected with specific data tables, acting as a single source of truth for all users.
Data Observability vs Monitoring
Data Monitoring and observability have long been used interchangeably, but if we look deep into them then we will come to know that these are 2 concepts that complement each other. Explore the guide on observability for the modern data stack to understand how it enhances data management and monitoring.
Feature | Monitoring | Observability |
Purpose | Collects and captures data about system behavior. | Interprets, analyzes, and assesses system health using collected data. |
Scope | Focuses on gathering metrics, logs, and traces. | Goes beyond data collection, involving analysis and discovering patterns or anomalies in the data. |
Function | Tells you what is wrong. | Helps you understand why something is wrong and suggests potential remedies. |
Depth of Insight | Alerts when something goes wrong (e.g., application performance). | Provides detailed insights into why issues occur, such as identifying problematic microservices. |
Actionability | Provides notifications for problems. | Offers deeper analysis, guiding corrective actions and solutions. |
Data Correlation | Limited to individual metrics and logs. | Correlates data from different sources to identify root causes and significant patterns. |
Role in System Health | Basic understanding of issues. | Comprehensive understanding of system performance, aiding in reliability planning and mitigation. |
What are the Top 6 Data Observability Tools?
In data pipelines, observability matters because pipelines are now highly complicated with many independent and concurrent systems. Complexities can create dangerous dependencies, and you need an opportunity to avoid them. That’s where data observability tools come into play.
Some of the best tools are as follows:
1) Monte Carlo
Monte Carlo provides an end-to-end Data Observability platform that helps prevent broken data pipelines. This is an excellent tool for data engineers to ensure reliability and avoid potentially costly data downtime.
- This tool is a data reliability platform that helps you keep your data clean and credible and helps your team build trust in your data by decreasing data downtime.
- It uses Machine Learning algorithms to understand what your data looks likes and compares it to how good data should look.
- This tool helps in discovering bad data, alerts about potential data downtime, and gauges its impact so that the right set of people can fix the issue.
2) DataBuck
Powered by Machine Learning, DataBuck is an advanced observability tool. It automatically figures out what data is expected, when, and where. The right stakeholders get alerted when there are deviations.
Benefits of DataBuck:
- Ensures data is reliable
- as soon as it lands in the lake
- as it cascades down the pipeline
- and also in the data warehouse
- The only solution that leverages AI/ML to identify hard-to-detect errors
- Plug and play for Data Engineers – no rules writing
- Self-service for business stakeholders
- Auditable
3) Databand
Databand is an AI-powered platform that provides tools for data engineering teams to smoothen their operations and obtain unified insight into their data flows.
- This method ensures that pipelines are fully completed while keeping an eye on resource consumption and expenses.
- Its goal is to enable more effective data engineering in today’s complicated digital infrastructure.
- Databand’s aim is to explore and figure out the exact reason and location of the failure of the data pipelines before any bad data gets through. In the modern data stack, the platform also incorporates cloud-native technologies such as Apache Airflow and Snowflake.
4) Honeycomb
The observability tool in Honeycomb gives developers the visibility they need to fix issues in distributed systems. According to the firm, “Honeycomb makes complicated interactions within your dispersed services easier to comprehend and debug.”
- Its full-stack cloud-based observability technology supports events, logs, and traces, as well as automatically instrumented code via its agent.
- Honeycomb also supports OpenTelemetry in the process of generating instrumentation data.
5) Acceldata
Acceldata offers data pipeline monitoring, data reliability, and data observability products.
- The tools are designed to help data engineering teams acquire extensive and cross-sectional views of complicated data pipelines.
- Acceldata’s technologies synthesize signals across many layers and workloads to let multiple teams collaborate to resolve data issues.
- Furthermore, Acceldata Pulse assists in performance monitoring and observation to ensure data dependability at scale.
- The tool is intended for use in the financial and payments industries.
6) Datafold
Datafold’s solution assists data teams in evaluating data quality by utilizing diffs, anomaly detection, and profiling.
- Its features allow teams to do data Quality Assurance by profiling data, comparing tables across databases or inside a database, and generating smart notifications from any SQL query with a single click.
- Furthermore, data teams may track ETL code changes as they occur during data transfers. You can then connect them to their CI/CD to review the code rapidly.
Key Features of Data Observability Tools
Some of the key features of these tools are as follows:
- These tools can easily help you connect to your existing stack in a very fast and seamless manner. You don’t even need to modify your data pipelines or write any piece of code in any programming language. As a result, this enables you to maximize your testing coverage.
- These tools monitor your data while it is at rest, and you don’t need to extract the data from where it is currently stored. This makes them highly performant and cost-effective.
- These tools ensure that the users must meet the most stringent security and compliance standards.
- These tools prevent problems from occurring in the first place by revealing detailed information about data assets and reasons for gaps in data. This allows for responsible and proactive alterations and revisions thus increasing efficiency and saving time.
- You don’t need to do any prior mapping of what should be monitored and in what way. These tools assist you in identifying important resources, key dependencies, and key invariants, allowing you to achieve enhanced data observability with minimal effort.
- These tools offer comprehensive context for speedy evaluation and troubleshooting, as well as effective communication with stakeholders affected by data reliability concerns.
- These tools reduce false positives by considering not just specific measurements but a comprehensive perspective of your data and the possible implications of any single issue.
Conclusion
In this article, you have learned about the concept of data observability. The article also covered its importance and the five pillars of the approach and compared it to data monitoring. Additionally, you explored the top five tools for data observation and their key features.
Hevo Data, a No-code Data Pipeline, provides you with a consistent and reliable solution to manage data transfer between a variety of sources and a wide variety of Desired Destinations with a few clicks.
Hevo Data, with its strong integration with 150+ Data Sources (including 40+ Free Sources), allows you to not only export data from your desired data sources & load it to the destination of your choice but also transform & enrich your data to make it analysis-ready.
Want to give Hevo a try? Sign Up for a 14-day free trial and experience the feature-rich Hevo suite firsthand. You may also have a look at the amazing price, which will assist you in selecting the best plan for your requirements.
Share your experience of understanding Data Observability in the comment section below!
FAQs
1. What is KPI in observability?
A KPI (Key Performance Indicator) in observability is a measurable value that tracks system health, performance, or reliability, helping assess how well a system meets goals like uptime or response time.
2. What is the hierarchy of data observability?
The hierarchy includes data freshness, data distribution, data volume, schema changes, and lineage, which help monitor, understand, and trust data across its lifecycle.
3. What are the 4 signals of observability?
The 4 key signals of observability are metrics, logs, traces, and events, providing insights into system behavior, performance issues, and root cause identification.