Easily move your data from MariaDB to BigQuery to enhance your analytics capabilities. With Hevo’s intuitive pipeline setup, data flows in real-time—check out our 1-minute demo below to see the seamless integration in action!
The volume of data that businesses collect has grown exponentially over the past few years. This could be data related to things aspects such as how people are interacting with their product or service, what people think of their offerings, how well is the performance of their marketing efforts, etc. Businesses can then use this data to make data-driven decisions and plan their future strategies accordingly.
One of the most widely used Relational Database Management Systems (RDBMS) is MariaDB. This article will introduce you to MariaDB and Google BigQuery. It will also help you understand how you can set up MariaDB to BigQuery Migration to analyze your MariaDB data.
Table of Contents
Introduction to MariaDB
MariaDB is a popular Open-source Relational Database Management System (RDBMS). It was developed as a software fork of another popular Open-source database, MySQL, by the developers who played key roles in developing the original database. MariaDB was devised in 2009 when MySQL was acquired by Oracle. It was designed to ensure ease of use, speed, and reliability for all its users.
Like all other Relational Database Management Systems (RDBMS), MariaDB houses support for ACID-Compliant Data Processing. Along with that, it also supports parallel Data Replication, JSON APIs, and multiple storage engines, including InnoDB, Spider, MyRocks, Aria, Cassandra, TokuDB, and MariaDB ColumnStore.
More information on MariaDB can be found here.
Understanding the Key Features of MariaDB
The key features of MariaDB are as follows:
- Robust Transactional Support: Implementation of ACID (Atomicity, Consistency, Isolation, Durability) properties ensures no data loss or inconsistency.
- Ease of Use: Considering that it makes use of SQL for querying data, anyone with basic knowledge of SQL can perform the required tasks with ease.
- Security: It implements a complex data security layer that ensures that only authorized users can access sensitive data.
- Scalable: MariaDB is considered to be highly scalable due to its support for multi-threading.
- Roll-back Support: MariaDB houses support for roll-backs, commits, and crash recovery for all transactions.
- High Performance: MariaDB houses various fast load utilities along with Table Index Partitioning and Distinct Memory Caches that can help ensure high performance.
Method 1: Manual MariaDB to BigQuery Migration
This method involves manually extracting data from MariaDB as CSV and then loading it into Google BigQuery to set up manual MariaDB to BigQuery Migration. While effective, this method can overload your database in case of larger datasets, and can slow the performance of the database.
Method 2: MariaDB to BigQuery Migration Using Hevo Data
You can use an automated solution that Hevo provides to prevent your database from getting overloaded. Perform complex migrations from 150+ sources like MariaDB to BigQuery or any other destination of your choice in minutes.
Sign up here for a 14-day Free Trial!Introduction to Google BigQuery
BigQuery is Google’s cloud enterprise data warehouse that primarily serves business agility in running complex SQL queries and performing analysis on huge datasets efficiently. It is based on Google technology called Dremel, using columnar storage and tree architecture to support high-speed scanning of data for querying efficiency.
BigQuery is serverless, highly scalable, and enlists Google’s infrastructure in the cloud for management. It uses access patterns to maintain efficiency in storage by dynamically optimising data structures for the handling of data in a changing environment for optimal performance.
Understanding the Key Features of Google BigQuery
- Fully Managed by Google: All databases or Data Warehouses built on Google BigQuery are deployed, maintained, and managed directly by Google.
- Integrations: Google BigQuery, being a part of the Google Cloud Platform (GCP) supports seamless integration with all Google products and services. Google also provides a wide variety of integrations with numerous third-party services along with functionality to integrate with the APIs of applications that are not directly supported by Google.
- High Data Processing Speed: Google BigQuery was designed to enable users to perform real-time analysis of their data.
- Serverless: In Google BigQuery’s Serverless model, the processing is automatically distributed over a vast number of machines that are working in parallel. Hence, any business making use of Google BigQuery can focus on gaining insights from data rather than on setting up and maintaining the infrastructure.
- Google BigQuery ML: Google BigQuery houses a functionality called BigQuery Machine Learning (BQML) that gives users the ability to create and execute Machine Learning models using standard SQL queries.
Understanding the Need for MariaDB to BigQuery Migration
Businesses feel the need to set up MariaDB to BigQuery Migration because of the following reasons:
- Powerful Analytics: There are numerous analytics workloads that businesses can run on a Data Warehouse. Since Google BigQuery houses an SQL engine, businesses can use Business Intelligence tools like Google Data Studio, Looker, and Tableau to create descriptive data visualizations and reports.
- Machine Learning Capabilities: Google BigQuery goes beyond conventional Data Warehousing. It can be used to create robust Machine Learning models to carry out batch predictions without having to export data out of Google BigQuery.
- Simplified Workflows: Google BigQuery by design is meant to encourage customers to focus on gathering insights as opposed to managing infrastructure. This approach allows teams to innovate faster with fewer resources. DBAs are not needed to provision and maintain servers, and this enables them to work with a lean team of Data Analysts.
- Scale-out Architecture: From an architectural point of view, the only limit on speed and scale in Google BigQuery is the amount of hardware in the Availability Zone (AZ). Queries are automatically scaled to thousands of machines and executed in parallel. This is the same infrastructure used on other Google products like AdWords, YouTube, Gmail, G-Suite, Google Search, etc.
- Rich Product Ecosystem: Google BigQuery is part of the GCP ecosystem, and it integrates tightly with Cloud AI, Cloud DLP, AutoML, Data Studio, Cloud Scheduler, Cloud Functions, Cloud Composer, etc.
Methods to Set up MariaDB to BigQuery Migration
Users can set up MariaDB to BigQuery Migration by implementing one of the following methods:
Method 1: Manual MariaDB to BigQuery Migration
Users can set up manual MariaDB to BigQuery Migration by implementing the following steps:
- Step 1: Export your MariaDB data into CSV format by running the following query:
mysql --host=[INSTANCE_IP] --user=[USER_NAME] --password [DATABASE]
-e " SELECT * FROM mydataset.mytable INTO OUTFILE 'myfile.csv' CHARACTER SET 'utf8mb4'
FIELDS TERMINATED BY ',' OPTIONALLY ENCLOSED BY '"' ESCAPED BY '"' "
When executed, the output of this command will be a CSV file with the data from the specified table.
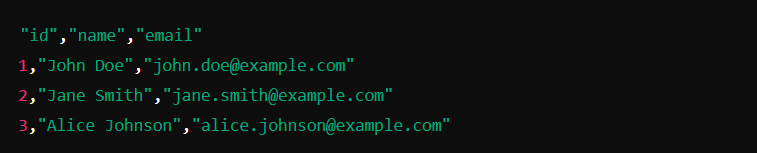
- Step 2: Once the data has been exported from MariaDB as CSV, it has to be imported into Google BigQuery. Users can easily perform a batch-load job in Python by running the following code:
from google.cloud import bigquery
# Construct a BigQuery client object.
client = bigquery.Client()
# TODO(developer): Set table_id to the ID of the table to create.
# table_id = "your-project.your_dataset.your_table_name"
job_config = bigquery.LoadJobConfig(
source_format=bigquery.SourceFormat.CSV, skip_leading_rows=1, autodetect=True,
)
with open(file_path, "rb") as source_file:
job = client.load_table_from_file(source_file, table_id, job_config=job_config)
job.result() # Waits for the job to complete.
table = client.get_table(table_id) # Make an API request.
print(
"Loaded {} rows and {} columns to {}".format(
table.num_rows, len(table.schema), table_id
)
)

In this example, I have loaded 1000 rows and 5 columns from the CSV file generated to the BigQuery table.
Limitations of Manual MariaDB to BigQuery Migration
The limitations associated with manual MariaDB to BigQuery Migration are as follows:
- As Google BigQuery does not support real-time data synchronisation, it becomes really hard to provide support for live updates coming from MariaDB. You can consider CDC implementation in the case of real-time needs, but it might be inefficient.
- BigQuery supports datasets of huge sizes, with CSV file sizes of up to 5 TB and a maximum load job size of 15 TB. However, it has a 100 MB row and cell size limit, which can be difficult to manage.
- BigQuery does not guarantee data consistency in case of live data updates. The results can be unpredictable if the base data changes during the execution of a query. Additional coding is needed for data cleaning and transformation before loading.
Method 2: MariaDB to BigQuery Migration Using Hevo Data
Using Hevo Data, you can automatically migrate your data from MariaDB to BigQuery in just two easy steps. I have shown a step-by-step implementation of this method below for your reference.
Step 1: Configure MariaDB as the Souce.
You can choose MariaDB as the source for your data migration.
Step 1.1: Select MariaDB as the source.
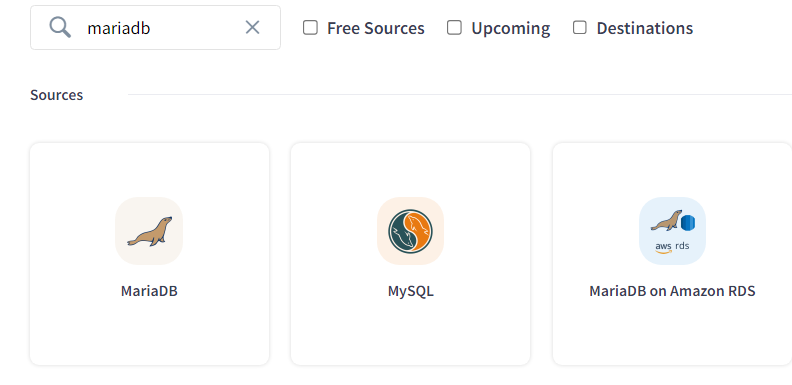
Step 1.2: You need to provide access to your MariaDB database by giving the credentials to it.
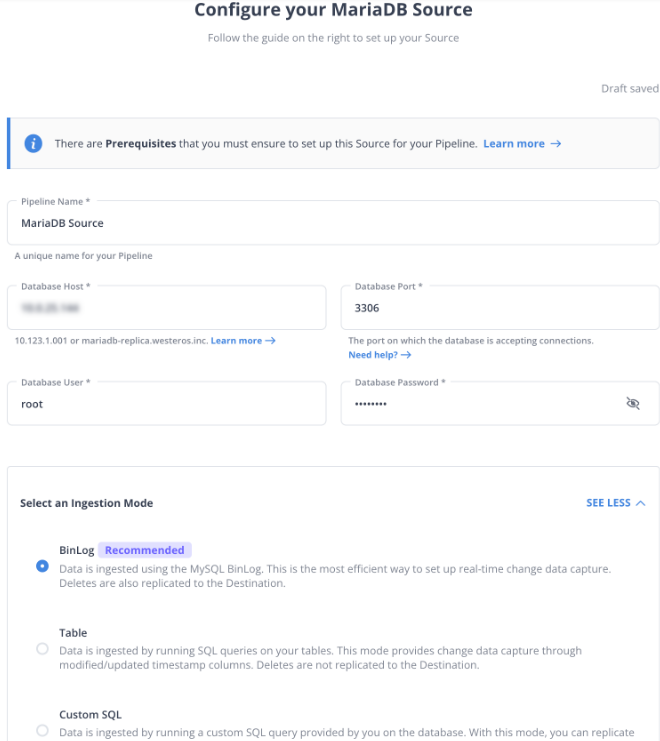
Note: You can also select the ingestion method using which you want to migrate your data. This function is exclusively available only for database systems.
Step 1.3: Click on Test and Run.
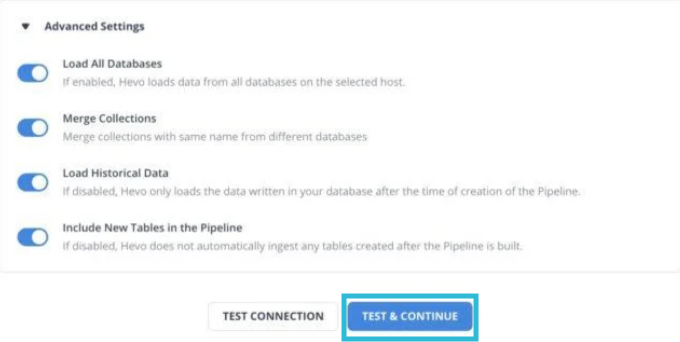
Step 2: Configure BigQuery as your Destination
You can select BigQuery as your destination by providing credentials to your Google account.
Step 2.1: Select BigQuery as your Destination.
Step 2.2: Provide credentials to your BigQuery account.
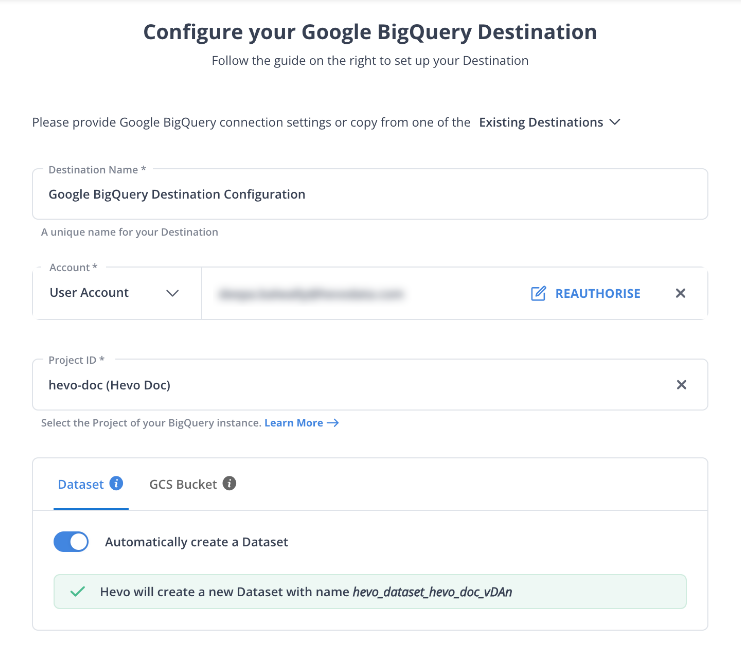
Step 2.3: Save and Continue to run the pipeline.
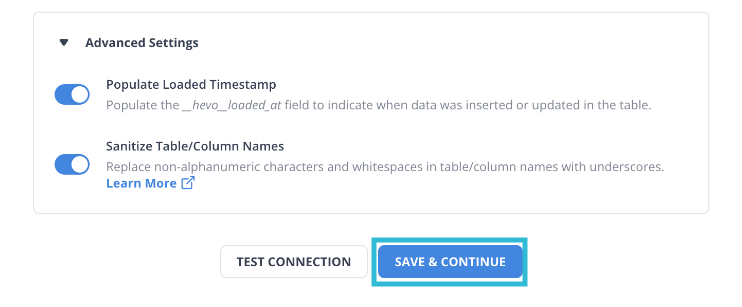
In just a few minutes, your data will automatically migrate from MariaDB to BigQuery without requiring any human intervention.
Learn More:
Conclusion
This article provided you with a step-by-step guide on how you can set up MariaDB to BigQuery Migration manually or using Hevo. However, there are certain limitations associated with the manual method. If those limitations are not a concern to your operations, then using it is the best option but if it is, then you should consider using automated Data Integration platforms like Hevo.
Hevo helps you directly transfer data from a source of your choice to a Data Warehouse, Business Intelligence, or desired destination in a fully automated and secure manner without having to write the code. It will make your life easier and make data migration hassle-free. It is User-Friendly, Reliable, and Secure.
Want to take Hevo for a spin? Sign Up for a 14-day free trial and experience the feature-rich Hevo suite first hand.
Share your experience of setting up MariaDB to BigQuery Integration in the comments section below!
FAQs to load data from MariaDB to BigQuery
1. How do we migrate data from MariaDB to Oracle?
To migrate data from MariaDB to Oracle, you can use data export tools like mysqldump to export MariaDB data to CSV or SQL files and then use Oracle SQL*Loader or Oracle Data Pump to import the data into Oracle. Alternatively, ETL tools like Hevo can automate and streamline this process.
2. How to migrate data from MariaDB to SQL Server?
To migrate data from MariaDB to SQL Server, use tools like mysqldump to export MariaDB data to CSV or SQL format and then utilize SQL Server’s Import and Export Wizard to import the data.
3. Is Google BigQuery expensive?
Google BigQuery’s cost depends on usage, including data storage and query processing. While it offers a pay-as-you-go model with flexible pricing, costs can accumulate with large datasets and frequent queries.