Migrating from an API to BigQuery using custom code involves extracting data from the API, transforming it into a structured format, and then loading it into BigQuery. However, using no-code tools like Hevo Data can automate the entire process in just two simple steps.
Detailed Methods:
- Step 1: Getting your data out of your application using API
- Step 2: Preparing the data that was extracted from the Application
- Step 3: Loading data into Google BigQuery
- Step 1: Configure REST API as your Source
- Step 2: Configure BigQuery as your Destination
Many businesses use cloud-based applications like Salesforce, HubSpot, Mailchimp, and Zendesk for daily operations. We need to combine data from these sources to measure key metrics and drive growth.
These applications, run by third-party vendors, provide APIs for data extraction into data warehouses like Google BigQuery. In this blog, we’ll walk you through the process of moving data from an API to BigQuery, discuss potential challenges, and share workarounds. Let’s dive in!
Note: When you perform this integration, consider factors like data format, update frequency, and API rate limits to design a stable integration.
Overview of BigQuery
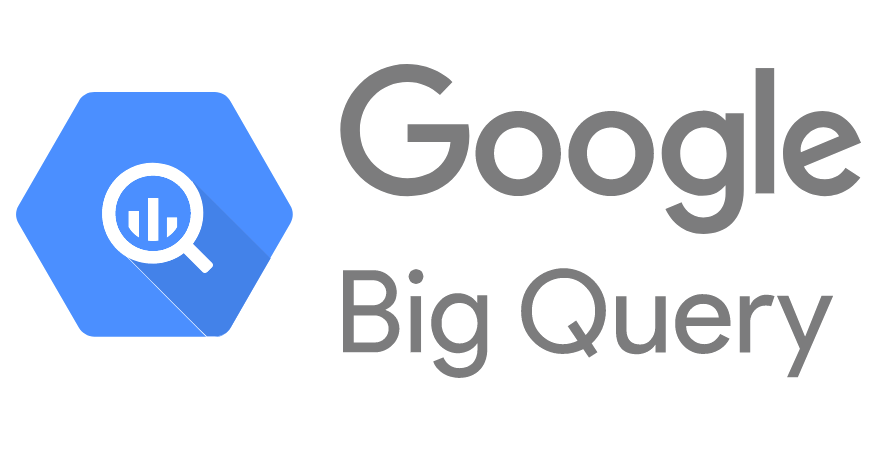
Google BigQuery is a cloud data warehouse service provider and a part of the Google Cloud Platform. It helps companies store and analyze their business data at a secure data warehouse. Google allows users to leverage other Google Cloud Platform features, such as engines, APIs, etc, on their data directly from the Google BigQuery data warehouse.
Google BigQuery can manage terabytes of data using SQL language. Also, it enables companies to analyze their data stored in Data Warehouse using SQL queries. Google BigQuery has a columnar storage structure that helps deliver faster query processing and file compression. Moreover, BigQuery ML allows users to train and run machine learning models in BigQuery using only SQL syntax.
Unleash the full potential of your API data with Hevo’s no-code platform. Skip the coding and dive straight into real-time BigQuery insights as Hevo effortlessly handles data transfer, schema mapping, and error handling—all while you focus on what matters most: your analysis.
Check out what makes Hevo amazing:
- Schema Management: Hevo can automatically detect the schema of the incoming data and map it to the destination schema.
- Scalable Infrastructure: Hevo has in-built integrations for 150+ data sources (with 60+ free sources) that can help you scale your data infrastructure as required.
- Live Support: Hevo team is available round the clock to extend exceptional support to its customers through chat, email, and support calls.
Try to see why customers like Voiceflow and ScratchPay have upgraded to a powerful data and analytics stack by incorporating Hevo!
Get Started with Hevo for Free!Why Connect APIs to BigQuery?
Integrating APIs with BigQuery offers several advantages:
- Centralized Analytics: Consolidate data from multiple APIs for unified reporting.
- Real-Time Insights: Analyze streaming data for timely decision-making.
- Scalability: Handle large datasets without worrying about infrastructure.
- Automation: Minimize manual effort and ensure consistent data pipelines.
Use Cases of API and BigQuery Integration
- Social Media Analytics: Fetch data from Twitter or Instagram to analyze engagement trends.
- IoT Data Aggregation: Collect sensor data to monitor equipment performance in real-time.
- E-Commerce Tracking: Combine data from APIs like Shopify or Stripe for sales and inventory analysis.
- Advanced Analytics: BigQuery has powerful data processing capabilities that enable you to perform complex queries and data analysis on your API data. This way, you can extract insights that would not be possible within API alone.
- Data Consolidation: If you’re using multiple sources along with API, syncing them to BigQuery can help you centralize your data. This provides a holistic view of your operations, and you can set up a change data capture process to avoid discrepancies in your data.
- Historical Data Analysis: API has limits on historical data. However, syncing your data to BigQuery allows you to retain and analyze historical trends.
- Machine Learning: You can apply machine learning models to your data for predictive analytics, customer segmentation, and more by having API data in BigQuery
Method 1: Loading Data Using Hevo
Step 1: Configure REST API as your source
Step 2: Configure BigQuery as your Destination
Yes, that is all. Hevo will do all the heavy lifting to ensure that your analysis-ready data is moved to BigQuery securely, efficiently, and reliably.
To learn more about configuring REST API as your source, refer to Hevo Documentation.
Method 2: Using Custom Code
The BigQuery Data Transfer Service allows scheduling and managing transfers from REST API data sources to Bigquery for supported applications.
One advantage of the REST API to Google BigQuery is the ability to perform actions (like inserting data or creating tables) that might not be directly supported by the web-based BigQuery interface. The steps involved in migrating API data manually to BigQuery are as follows:
- Getting your data out of your application using API
- Preparing the data that was extracted from the Application
- Loading data into Google BigQuery
Step 1: Getting data out of your application using API
Below are the steps to extract data from the application using API.
Get the API URL from where you need to extract the data. In this article, you will learn how to use Python to extract data from Exchangerate-api.com, a free service for current and historical foreign exchange rates published by the European Central Bank. The same method should broadly work for any API you want.
API URL = https://v6.exchangerate-api.com/v6/[Access-Key]/latest/USD
If you click on the above URL, you will get the following result format:
Note: Replace [Access-Key] with your actual access token.
{
"result": "success",
"documentation": "https://www.exchangerate-api.com/docs",
"terms_of_use": "https://www.exchangerate-api.com/terms",
"time_last_update_unix":1722556802,
"time_last_update_utc":"Fri, 02 Aug 2024 00:00:02 +0000",
"time_next_update_unix":1722643202,
"time_next_update_utc":"Sat, 03 Aug 2024 00:00:02 +0000",
"base_code": "USD",
"conversion_rates": {
"USD": 1,
"AUD": 1.4817,
"BGN": 1.7741,
"CAD": 1.3168,
"CHF": 0.9774,
"CNY": 6.9454,
"EGP": 15.7361,
"EUR": 0.9013,
"GBP": 0.7679,
"...": 7.8536,
"...": 1.3127,
"...": 7.4722, etc. etc.
}
}
Reading and Parsing API response in Python:
a. To handle API response, will need two important libraries
import requests
import json
b. Connect to the URL and get the response
url = 'https://v6.exchangerate-api.com/v6/YOUR-API-KEY/latest/USD'
response = requests.get(url)
c. Convert string to JSON format
data = response.json()
d. Extract data and print
parsed = json.loads(data)
eur_rate = parsed["conversion_rates"]["EUR"]
usd_rate = parsed["conversion_rates"]["USD"]
Here is the complete code:
import requests
import json
url = "https://v6.exchangerate-api.com/v6/YOUR-API-KEY/latest/USD"
response = requests.get(url)
data = response.json()
parsed = json.loads(data)
eur_rate = data["conversion_rates"]["EUR"]
usd_rate = parsed["conversion_rates"]["USD"]
print(str(usd_rate) + "USD equals " + str(eur_rate) + " EUR")
Step 2: Preparing data received from API
There are two ways to load data to BigQuery.
- You can save the received JSON formatted data on a JSON file and then load it into BigQuery.
- You can parse the JSON object, convert JSON to a dictionary object, and load it into BigQuery.
To convert JSON data into a CSV or JSON Lines format compatible with BigQuery, run the following code:
import csv
with open('data.csv', 'w', newline='') as file:
writer = csv.writer(file)
writer.writerow(["id", "name", "value"])
for item in data:
writer.writerow([item['id'], item['name'], item['value']])
Step 2.3: Loading data into Google BigQuery
We can load data into BigQuery directly using an API call or create a CSV file and then load it into a BigQuery table.
- Create a Python script to extract data from the API URL and load (UPSERT mode) into the BigQuery table. Here, UPSERT is nothing but Update and Insert operations. If the target table has matching keys, then update the data; otherwise, insert a new record.
import requests
import json
from google.cloud import bigquery
url = "https://v6.exchangerate-api.com/v6/YOUR-API-KEY/latest/USD"
response = requests.get(url)
data = response.text
parsed = json.loads(data)
base = parsed["base"]
date = parsed["date"]
client = bigquery.Client()
dataset_id = 'my_dataset'
table_id = 'currency_details'
table_ref = client.dataset(dataset_id).table(table_id)
table = client.get_table(table_ref)
for key, value in parsed.items():
if type(value) is dict:
for currency, rate in value.items():
QUERY = ('SELECT target_currency FROM my_dataset.currency_details where currency=%', currency)
query_job = client.query(QUERY)
if query_job == 0:
QUERY = ('update my_dataset.currency_details set rate = % where currency=%',rate, currency)
query_job = client.query(QUERY)
else:
rows_to_insert = [(base, currency, 1, rate)]
errors = client.insert_rows(table, rows_to_insert)
assert errors == []
- Load JSON file to BigQuery. You need to save the received data in a JSON file and load the JSON file into the BigQuery table.
import requests
import json
from google.cloud import bigquery
url = "https://v6.exchangerate-api.com/v6/YOUR-API-KEY/latest/USD"
response = requests.get(url)
data = response.text
parsed = json.loads(data)
for key, value in parsed.items():
if type(value) is dict:
with open('F:Pythondata.json', 'w') as f:
json.dump(value, f)
client = bigquery.Client(project="analytics-and-presentation")
filename = 'F:Pythondata.json'
dataset_id = 'dayaset'
table_id = 'currency_rate_details'
dataset_ref = client.dataset(dataset_id)
table_ref = dataset_ref.table(table_id)
job_config = bigquery.LoadJobConfig()
job_config.source_format = bigquery.SourceFormat.NEWLINE_DELIMITED_JSON
job_config.autodetect = True
with open(filename, "rb") as source_file:
job = client.load_table_from_file(source_file, table_ref, job_config=job_config)
job.result() # Waits for table load to complete.
print("Loaded {} rows into {}:{}.".format(job.output_rows, dataset_id, table_id))
Limitations of writing custom scripts and developing ETL
- The above code is written based on the current source and target destination schema. If either the incoming data or the schema on BigQuery changes, the ETL process will break.
- Modern data integration solutions like News search API demonstrate how robust ETL processes can handle schema changes effectively.
- If you need to clean your data from API, transform time zones, hide personally identifiable information, and so on, the current method does not support it. You will need to build another set of processes to accommodate that. This would also require you to invest extra effort and money.
- You are at a serious risk of data loss if, at any point, your system breaks. This could be anything from the source/destination that is not reachable to script breaks and more. You must invest upfront in building systems and processes that capture all the fail points and consistently move your data to the destination.
- Since Python is an interpreted language, it might cause performance issues when extracting from API and loading data into BigQuery API.
- For many APIs, we need to supply credentials to access API. Passing credentials as plain text in Python script is a very poor practice. You will need to take additional steps to ensure your pipeline is secure.
Challenges in API-to-BigQuery Integration
While connecting APIs to BigQuery unlocks significant value, it comes with its own set of challenges:
- API Rate Limits: Excessive requests may lead to throttling or blocked access.
- Authentication Complexities: Handling OAuth tokens or API keys securely.
- Data Transformation: Converting API data formats to match BigQuery’s schema.
- Error Handling: Managing failed API calls or incomplete data uploads.
Best Practices for API Data Ingestion
- Batch Requests: Avoid rate-limit issues by batching API calls.
- Schema Validation: Ensure API data matches your BigQuery table schema to avoid errors.
- Monitor Pipelines: Set up alerts for failed or delayed data loads.
- Use BigQuery Streaming API: For real-time data, use the streaming API to ensure low-latency ingestion.
- Partition Tables: Organize data by date or other criteria to reduce query costs.
- Avoid Redundant API Calls: Cache responses to minimize unnecessary requests.
Additional Resources
Conclusion
From this blog, you will understand the process you need to follow to load data from API to BigQuery. This blog also highlights various methods and their shortcomings. Connecting APIs to BigQuery can unlock immense value for your business by enabling real-time analytics and centralized data management.
While manual integration offers flexibility, automated solutions like Hevo simplify the process and save time. By following best practices and optimizing your pipelines, you can ensure a seamless and cost-effective data integration experience. Ready to simplify your API-to-BigQuery integration? Try Hevo for Free.
FAQs
1. How to connect API to BigQuery?
1. Extracting data out of your application using API
2. Transform and prepare the data to load it into BigQuery.
3. Load the data into BigQuery using a Python script.
4. Apart from these steps, you can also use automated data pipeline tools to connect your API url to BigQuery.
2. Is BigQuery an API?
BigQuery is a fully managed, serverless data warehouse that allows you to perform SQL queries. It provides an API for programmatic interaction with the BigQuery service.
3. What is the BigQuery data transfer API?
The BigQuery Data Transfer API offers a wide range of support, allowing you to schedule and manage the automated data transfer to BigQuery from many sources. Whether your data comes from YouTube, Google Analytics, Google Ads, or external cloud storage, the BigQuery Data Transfer API has you covered.
4. How to input data into BigQuery?
Data can be inputted into BigQuery via the following methods.
1. Using Google Cloud Console to manually upload CSV, JSON, Avro, Parquet, or ORC files.
2. Using the BigQuery CLI
3. Using client libraries in languages like Python, Java, Node.js, etc., to programmatically load data.
4. Using data pipeline tools like Hevo
5. What is the fastest way to load data into BigQuery?
The fastest way to load data into BigQuery is to use automated Data Pipeline tools, which connect your source to the destination through simple steps. Hevo is one such tool.
AnalogOne says:
Great Information. Thanks for Sharing.