Migrating data from MongoDB to Snowflake usually involves exporting the data, staging the files and finally connecting to Snowflake. This process can be simplifies using Hevo’s no-code automated ETL platform.
Breakdown of Methods:
Method 1: Using Hevo Data
Step 1: Configure MongoDB as a Source
Step 2: Configure Snowflake as a Destination
Method 2: Writing Custom Scripts
Step 1: Extracting data from MongoDB Collections
Step 2: Optional Data Type conversions and Data Formatting
Step 3: Staging Data Files
Step 4: Copying Staged Files to Snowflake Table
Step 5: Migrating to Snowflake
Organizations often need to integrate data from multiple sources into a single destination to gain valuable insights. One common scenario involves transferring data from MongoDB to Snowflake for advanced analytics and business intelligence.
However, this process can be challenging, especially for those new to data engineering. In this blog, we have explored three seamless methods to migrate data from MongoDB to Snowflake, ensuring a smooth and efficient data integration process.
Mongodb’s real-time replication to Snowflake ensures that data is consistently synchronized between MongoDB and Snowflake databases. Since MongoDB operates in a schemaless manner, moving the data to a warehouse, like Snowflake, for meaningful analysis becomes vital.
Table of Contents
What is MongoDB?
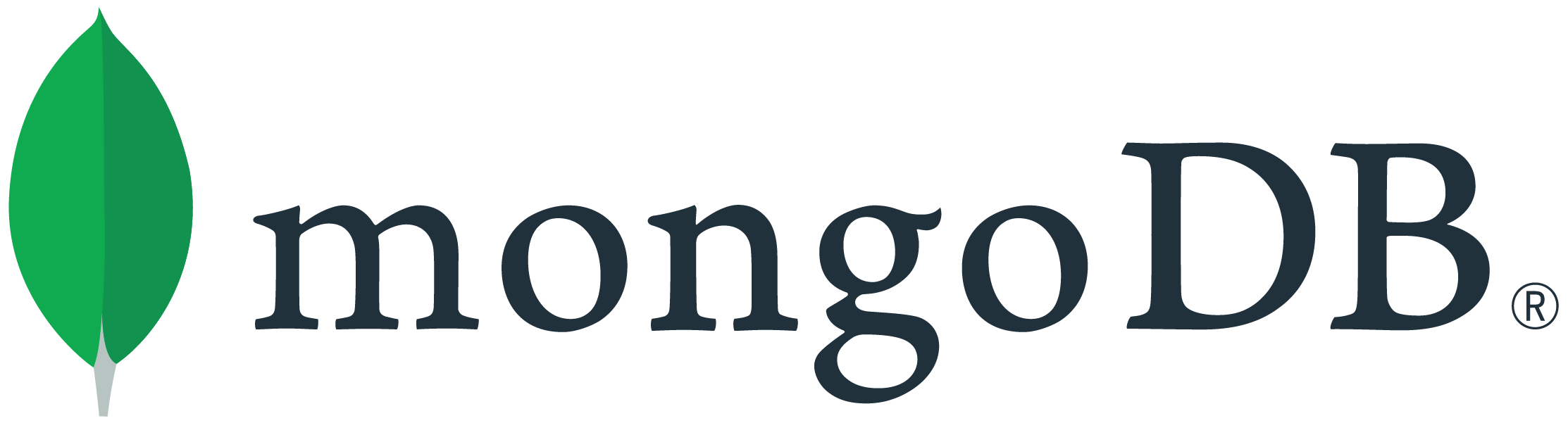
MongoDB is a popular NoSQL database management system designed for flexibility, scalability, and performance in handling unstructured or semi-structured data. This document-oriented database presents a view wherein data is stored as flexible JSON-like documents rather than the traditional table-based relational databases. Data in MongoDB is stored in collections, which contain documents.
Features of MongoDB
- Document-Oriented Storage: Stores data in flexible, JSON-like documents (BSON format), allowing for a dynamic schema and easy representation of complex data structures.
- Scalability: Supports horizontal scaling through sharding, distributing data across multiple servers to handle large volumes of data and high traffic.
- Indexing: Provides various indexing options, including single field, compound, geospatial, and text indexes, to optimize query performance.
- High Availability: Features replica sets for automatic failover and data redundancy, ensuring high availability and reliability.
What is Snowflake?
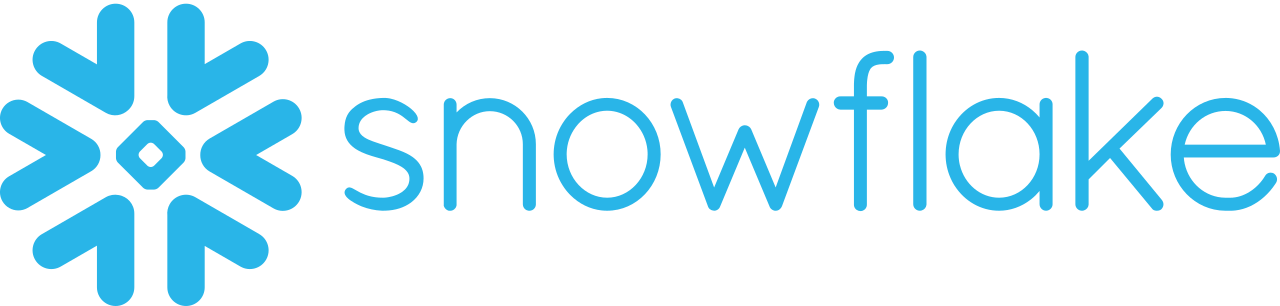
Snowflake is a fully managed service that provides customers with near-infinite scalability of concurrent workloads to easily integrate, load, analyze, and securely share their data. Its common applications include data lakes, data engineering, data application development, data science, and secure consumption of shared data. Snowflake’s unique architecture natively integrates computing and storage. Snowflake also provides various data types for you to export data in any format seamlessly.
Features of Snowflake
- Multi-cluster shared data architecture: It allows point-to-point scaling of computing resources independent of storage.
- Separate Storage and Compute: Optimizes cost performance by allowing storage and compute to scale independently.
- Seamless SQL: Snowflake SQL is straightforward to execute due to its built-in query platform, which enables you to run complex queries.
- Data Security with Sharing: Enables real-time data sharing without compromising privacy and security.
- Data Governance and Compliance:Advanced security features incorporate end-to-end encryption, complying with regulations.
Simplify your data migration from MongoDB to Snowflake using Hevo’s no-code platform. Hevo enables seamless, real-time data integration with automated workflows and reliable data sync, ensuring accurate insights across all your platforms.
Why Hevo is the Best:
- Minimal Learning Curve: Hevo’s simple, interactive UI makes it easy for new users to get started and perform operations.
- Connectors: With over 150 connectors, Hevo allows you to integrate various data sources into your preferred destination seamlessly.
- Schema Management: Hevo eliminates the tedious task of schema management by automatically detecting and mapping incoming data to the destination schema.
- Cost-Effective Pricing: Transparent pricing with no hidden fees, helping you budget effectively while scaling your data integration needs.
Trusted by 2000+ data professionals at companies like Postman and ThoughtSpot. Rated 4.4/5 on G2. Try Hevo and make your MongoDB to BigQuery migration seamless!.
Get Started with Hevo for FreeWhat are the Methods to Connect MongoDB to Snowflake
Here are the 3 easiest methods you can use to move data from MongoDB to Snowflake:
Method 1: Using Hevo’s No-Code Platform
Step 1: Configure MongoDB as a Source
For this method, you will be required to use an ETL tool. To explain this method, we will be using Hevo’s no-code data pipeline as our ETL tool.
- Step 1.1: Select MongoDB as the source.
- Step 1.2: Provide Credentials to MongoDB – You will need to provide details such as hostname, Password, Database Name, and Port number, enabling Hevo to access your data from the database.
Step 2: Configure Snowflake as a Destination
- Step 2.1: Select Snowflake as the Destination.
- Step 2.2: Enter Snowflake Configuration Details – You can enter the Snowflake Account URL you obtained.
Once you have successfully established a connection between your source and your destination, data will begin to flow automatically. That’s how easy Hevo makes it for you. With this, you have successfully set up MongoDB to Snowflake Integration using Hevo Data.
Method 2: Writing Custom Scripts to Move Data
Here is a brief overview of the broad framework for moving data.
The steps are:
- Step 1: Extracting data from MongoDB Collections
- Step 2: Optional Data Type conversions and Data Formatting
- Step 3: Staging Data Files
- Step 4: Copying Staged Files to Snowflake Table
- Step 5: Migrating to Snowflake
Let’s take a detailed look at all the required steps for MongoDB to Snowflake Integration:
Step 1: Extracting data from MongoDB Collections
MongoDB’s mongoexport utility can be used to create a JSON or CSV export of the data stored in any MongoDB collection.
The following points should be noted while using mongoexport :
Mongoexport
should be running directly in the system command line, not from the Mongo shell (the Mongo shell is the command-line tool used to interact with MongoDB)- The connecting user should have at least the read role on the target database. Otherwise, a permission error will be thrown.
mongoexport
, by default, uses primary read (direct read operations to the primary member in a replica set) as the read preference when connected to Mongos or a replica set.- Additionally, note that the default read preference, which is “primary read,” can be overridden using the –readPreference option
Below is an example on how to export data from the collection named contact_coln to a CSV file in the location /opt/exports/csv/col_cnts.csv
mongoexport --db users --collection contact_coln --type=csv --fields empl_name,empl_address --out /opt/exports/csv/empl_contacts.csv
- To export in CSV format, specify the column names in the collection to be exported. The above example specifies the empl_name and empl_address fields to export.
The output would look like this:
empl_name, empl_address
Prasad, 12 B street, Mumbai
Rose, 34544 Mysore
- You can also specify the fields to be exported in a file as a line-separated list of fields to export, with one field per line. For example, you can specify the emplyee_name and employee_address fields in a file empl_contact_fields.txt :
empl_name,
empl_address
Then, applying the --fieldFile
option, define the fields to export with the file:
mongoexport --db users --collection contact_coln --type=csv --fieldFile empl_contact_fields.txt --out /opt/backups/emplyee_contacts.csv
- Exported CSV files will have field names as a header by default. If you don’t want a header in the output file,–noHeaderLine option can be used.
- As in the above example –fields can be used to specify fields to be exported. It can also be used to specify nested fields. Suppose you have post_code filed with employee_address filed, it can be specified as employee_address.post_code
Incremental Data Extract From MongoDB to Snowflake
- So far, we have discussed extracting an entire MongoDB collection. It is also possible to filter the data while removing it from the collection by passing a query to filter the data. This can be used for incremental data extraction. –query or -q is used to pass the query.
- For example, let’s consider the contacts collection discussed above. Suppose the ‘updated_time’ field in each document stores the last updated or inserted Unix timestamp for that document.
mongoexport -d users -c contact_coln -q '{ updated_time: { $gte: 154856788 } }' --type=csv --fieldFile employee_contact_fields.txt --out exportdir/emplyee_contacts.csv
- The above command will extract all records from the collection with updated_time greater than the specified value,154856788. You should keep track of the last pulled updated_time separately and use that value while fetching data from MongoDB each time.
Step 2: Optional Data Type conversions and Data Formatting
Along with application-specific logic to be applied during data transfer, the following considerations have to be addressed when migrating data from MongoDB to Snowflake.
- Snowflake supports many character sets, including UTF-8. Read the complete list of supported encodings.
- If you have worked with cloud-based data warehousing solutions before, you might have noticed that most lack support and standard SQL constraints like
UNIQUE, PRIMARY KEY, FOREIGN KEY, NOT NULL
. However, keep in mind that Snowflake supports most SQL constraints. - Snowflake data types encompass all basic and semi-structured types, including arrays. It also offers built-in functions for handling semi-structured data. The list below displays Snowflake data types that are compatible with various MongoDB data types..
MongoDB Data Type | Snowflake Data Type |
DOUBLE | DOUBLE,
DOUBLE PRECISION |
STRING | STRING, TEXT |
BINARY DATA | BINARY |
OBJECTID | STRING, TEXT |
BOOLEAN | BOOLEAN |
DATE | DATE |
NULL | NULL |
32-BIT INTEGER | INTEGER |
TIMESTAMP | TIMESTAMP |
64-BIT INTEGER | INT64 |
DECIMAL128 | DECIMAL |
- As you can see from the table comparing MongoDB and Snowflake data types, Snowflake allows almost all date/time formats when inserting data. You can explicitly specify the format when loading data using the File Format Option. We will discuss this in detail later. The full list of supported date and time formats can be found here.
Step 3: Staging Data Files
Inputting data into a Snowflake table requires the data to be uploaded first to a cloud storage service, such as S3. This step is referred to as staging. Snowflake typically offers two kinds of stages: internal and external.
A. Internal Stage
For every user and table, Snowflake will create and allocate a staging location that is used by default for staging activities, and those stages will be named using some conventions mentioned below.
Note: It is also possible to create named internal stages.
- The user stage is named ‘@~’
- The name of the table stage is the name of the table.
- The user or table stages can’t be altered or dropped.
- It is not possible to set file format options in the default user or table stages.
Named internal stages can be created explicitly using SQL statements. While creating named internal stages, file format, and other options can be set, which makes loading data into the table very easy with minimal command options. SnowSQL features a lightweight CLI client that allows users to run commands such as DDLs or data loads. This provided in for Linux, macOS, and Windows.
Below are some example commands to create a stage:
create or replace stage my_mongodb_stage
copy_options = (on_error='skip_file')
file_format = (type = 'CSV' field_delimiter = '|' skip_header = 2);
The PUT
command is used to stage data files to an internal stage. The syntax is straightforward – you only need to specify the file path and stage name :
PUT file://path_to_file/filename internal_stage_name
E.g.:
Upload a file named emplyee_contacts.csv in the /tmp/mongodb_data/data/ directory to an internal stage named mongodb_stage
put file:////tmp/mongodb_data/data/emplyee_contacts.csv @mongodb_stage;
Several configurations can be used to maximize data load spread during file upload, such as the number of parallel threads and automatic data file compression.
B. External Stage
AWS and Azure are the industry leaders in the public cloud market. It is not surprising that Snowflake supports both Amazon S3 and Microsoft Azure for external staging locations. If the data is in S3 or Azure, all you need to do is create an external stage to point to it, and the data can be loaded into the table.
IAM credentials are to be specified to create an external stage on S3. If the data in S3 is encrypted, encryption keys should also be given.
create or replace stage mongod_ext_stage url='s3://snowflake/data/mongo/load/files/'
credentials=(aws_key_id='181a233bmnm3c' aws_secret_key='a00bchjd4kkjx5y6z');
encryption=(master_key = 'e00jhjh0jzYfIjka98koiojamtNDwOaO8=');
Data for the external stage can be uploaded using the respective cloud web interfaces, provided SDKs, or third-party tools.
Step 4: Copying Staged Files to Snowflake Table
COPY INTO
is the command used to load data from the stage area into the Snowflake table. Compute resources needed to load the data are supplied by virtual warehouses, and the data loading time will depend on the size of the virtual warehouses
E.g.:
A. To load from a named internal stage
copy into mongodb_internal_table
from @mngodb_stage;
B. To load from the external stage :(Here, only one file is specified)
copy into mongodb_external_stage_table
from @mongodb_ext_stage/tutorials/dataloading/employee_contacts_ext.csv;
C. To copy directly from an external location without creating a stage:
copy into mongodb_table
from s3://mybucket/snow/mongodb/data/files
credentials=(aws_key_id='$AWS_ACCESS_KEY_ID' aws_secret_key='$AWS_SECRET_ACCESS_KEY')
encryption=(master_key = 'eSxX0jzYfIdsdsdsamtnBKOSgPH5r4BDDwOaO8=')
file_format = (format_name = csv_format);
D. The subset of files can be specified using patterns
copy into mongodb_table
from @mongodb_stage
file_format = (type = 'CSV')
pattern='.*/.*/.*[.]csv[.]gz';
Some standard format options used in the COPY command for CSV format :
- COMPRESSION – Compression used for the input data files.
- RECORD_DELIMITER – The character used as records or line separator
- FIELD_DELIMITER -Character used for separating fields in the input file.
- SKIP_HEADER – Number of header lines to skip while loading data.
- DATE_FORMAT – Used to specify the date format
- TIME_FORMAT – Used to specify the time format
Step 5: Migrating to Snowflake
While discussing data extraction from MongoDB to Snowflake, both full and incremental methods are considered. Here, we will look at how to migrate that data from MongoDB to Snowflake effectively. Snowflake’s unique architecture helps to overcome many shortcomings of existing big data systems. Support for row-level updates is one such feature. Out-of-the-box support for row-level updates makes the delta data load to the Snowflake table simple. We can extract the data incrementally, load it into a temporary table, and modify records in the final table as per the data in the temporary table.
There are three popular methods to update the final table with new data after new data is loaded into the intermediate table.
- Update the rows in the final table with values from a temporary table, and insert new rows from the temporary table into the final table.
UPDATE final_mongodb_table t
SET t.value = s.value
FROM intermed_mongdb_table in
WHERE t.id = in.id;
INSERT INTO final_mongodb_table (id, value)
SELECT id, value FROM intermed_mongodb_table WHERE NOT id IN (SELECT id FROM final_mongodb_table);
2. Delete all rows from the final table that are also present in the temporary table. Then insert all rows from the intermediate table to the final table.
DELETE .final_mogodb_table f
WHERE f.id IN (SELECT id from intermed_mongodb_table);
INSERT final_mongodb_table (id, value)
SELECT id, value FROM intermed_mongodb_table;
3. MERGE statement – Both inserts and updates can be carried out simultaneously using a single MERGE
statement. We can use this option to apply changes to the temporary table.
MERGE into final_mongodb_table t1 using tmp_mongodb_table t2 on t1.key = t2.key WHEN matched then update set value = t2.value WHEN not matched then INSERT (key, value) values (t2.key, t2.value);
What are the Limitations of using Custom Scripts?
Although the manual method can complete your work, you may face some difficulties. Below, we have listed some limitations that might hinder your data migration from MongoDB to Snowflake:
- This approach works well if you want to migrate data in batches. However, this approach becomes highly tedious and time-consuming if you are looking for real-time data availability.
- With this method, you can only move data from one place to another, but not transform the data when in transit.
- When you create code to extract specific data, those scripts frequently fail due to changes or developments in the source schema, leading to potential data loss.
- Choosing between data platforms is crucial, especially when integrating MongoDB with Snowflake or Databricks to enhance your data architecture.
The method mentioned above has a high scope of errors. This may impact Snowflake’s availability and data accuracy.
Method 3: Using Native Cloud Tools and Snowpipe
Snowpipe, provided by Snowflake, enables a shift from the traditional scheduled batch-loading jobs to a more dynamic approach. It supersedes the conventional SQL COPY command, facilitating near real-time data availability. Snowpipe imports data into a staging area in smaller increments, working in tandem with your cloud provider’s native services, such as AWS or Azure.
For example, let’s explore these situations for each cloud provider, highlighting how your platform’s infrastructure integrates and how data is transferred from MongoDB to a Snowflake warehouse:
AWS: Utilize a Kinesis delivery stream to deposit MongoDB data into an S3 bucket. With an active SNS system, the associated successful run ID can be leveraged to import data into Snowflake using Snowpipe.
Azure: Activate Snowpipe with an Event Grid message corresponding to Blob storage events. Your MongoDB data is initially placed into an external Azure stage. Upon creating a blob storage event message, Snowpipe is alerted via Event Grid when the data is primed for Snowflake insertion. Subsequently, Snowpipe transfers the queued files into a pre-established table in Snowflake. For comprehensive guidance, Snowflake offers a detailed manual on the setup.
Limitations of Using Native Cloud Tools and Snowpipe
- A deep understanding of NoSQL databases, Snowflake, and cloud services is crucial. Troubleshooting in a complex data pipeline environment necessitates significant domain knowledge, which may be challenging for smaller or less experienced data teams.
- Long-term management and ownership of the approach can be problematic, as teams outside the Data department often control the resources used. This requires careful coordination with other engineering teams to establish clear ownership and ongoing responsibilities.
- The lack of native tools for applying the schema to NoSQL data presents challenges in schematizing the data, which may decrease its value in the data warehouse.
Why You Should Migrate from MongoDB to Snowflake
- Snowflake’s core is a powerful SQL engine that integrates BI and analytics tools seamlessly. Its SQL capabilities extend beyond relational data, enabling access to MongoDB’s JSON data, with its variable schema and nested structures, through SQL. Snowflake’s extensions and the creation of relational views make this JSON data readily usable with SQL-based tools.
- Snowflake’s system supports JSON natively, which is central to MongoDB’s document model. This allows direct loading of JSON data into Snowflake without needing to convert it into a fixed schema, eliminating the need for an ETL pipeline and concerns about evolving data structures.
- Snowflake’s architecture is designed for scalability and elasticity online. It can handle large volumes of data at varying speeds without resource conflicts with analytics, supporting micro-batch loading for immediate data analysis. Scaling upto a destination like a warehouse or data lake can reduce the time required for data loading, reducing the chances of causing downtime or requiring data redistribution.
- Migrating data from MongoDB to Snowflake allows businesses to leverage advanced analytics capabilities, particularly for use cases like customer segmentation and real-time reporting. Learn more about the use cases of connecting to MongoDB.
Additional Resources for MongoDB Integrations and Migrations
- Stream data from mongoDB Atlas to BigQuery
- Move Data from MongoDB to MySQL
- Connect MongoDB to Tableau
- Sync Data from MongoDB to PostgreSQL
- Move Data from MongoDB to Redshift
Conclusion
In this blog, we’ve walked you through three different ways to move your data from MongoDB to Snowflake. The effectiveness and difficulty of the migration can be greatly impacted by the method you select, and each strategy has advantages and disadvantages. Though they may provide flexibility, methods like Snowpipe and bespoke scripts can call for a great deal of human labor, sophisticated technological know-how, and potential problems with data consistency and real-time updates. You can use a no-code platform such as Hevo to automate and speed your data migration, or you can choose the pipeline method to make things simpler.
For using the Native Cloud Tools, you will need a deep understanding of NoSQL databases, Snowflake, and cloud services. Moreover, troubleshooting can also be troublesome in such an environment. On the other hand, leveraging Hevo simplifies and automates the migration process by providing a user-friendly interface and pre-built connectors.
Want to take Hevo for a spin? Sign up for a 14-day free trial with Hevo Data to streamline your migration process and leverage multiple connectors, such as MongoDB and BigQuery, for real-time analysis!
To explore a hassle-free data migration from MongoDB to Snowflake. You can also have a look at the unbeatable pricing that will help you choose the right plan for your business needs.
FAQs
1. Does MongoDB work with Snowflake?
Yes, MongoDB can work with Snowflake through data integration and migration processes.
2. How do I migrate a database to a Snowflake?
To migrate a database to Snowflake:
1. Extract data from the source database using ETL tools or scripts.
2. Load the extracted data into Snowflake using Snowflake’s data loading utilities or ETL tools, ensuring compatibility and data integrity throughout the process.
3. Can Snowflake handle NoSQL?
While Snowflake supports semi-structured data such as JSON, Avro, and Parquet, it is not designed to directly manage NoSQL databases.
4. Which SQL is used in Snowflake?
Snowflake uses ANSI SQL (SQL:2003 standard) for querying and interacting with data.