Snowflake’s architecture is defined newly from scratch, not an extension of the existing Big Data framework like Hadoop. It is a hybrid of the traditional shared-disk database and modern shared-nothing database architectures. Snowflake uses a central repository for persisted data that is accessible from all compute nodes in the data warehouse and processes queries using MPP (Massively Parallel Processing) compute clusters where each node in the cluster stores a portion of the data set.
Snowflake processes using “Virtual Warehouses,” which is an MPP compute cluster composed of multiple compute nodes. All components of Snowflake’s service run in a public Cloud-like AWS Redshift. This data warehouse is considered a cost-effective, high-performing analytical solution that is used by many organizations to manage critical workloads. In this post, we will discuss how to move real-time data from Postgres to Snowflake. So, read along and understand the steps to migrate data from Postgres to Snowflake.
Table of Contents
What is Postgres?
Postgres is an open-source Relational Database Management System (RDBMS) developed at the University of California, Berkeley. It is widely known for reliability, feature robustness, and performance and has been in use for over 20 years.
Postgres not only supports object-relational data but also supports complex structures and a wide variety of user-defined data types. This gives Postgres a definitive edge over other open-source SQL databases like MySQL, MariaDB, and Firebird.
Businesses rely on Postgres as their primary data storage/data warehouse for online, mobile, geospatial, and analytics applications. Postgres runs on all major operating systems, including Linux, UNIX (AIX, BSD, HP-UX, SGI IRIX, Mac OS X, Solaris, Tru64), and Windows.
What is Snowflake?
Snowflake is a fully-managed Cloud-based Data Warehouse that helps businesses modernize their analytics strategy. Snowflake can query both structured and unstructured data using standard SQL. It delivers results of user queries spanning Gigabytes and Petabytes of data in seconds.
Snowflake automatically harnesses thousands of CPU cores to quickly execute queries for you. You can even query streaming data from your web, mobile apps, or IoT devices in real-time.
Snowflake comes with a web-based UI, a command-line tool, and APIs with client libraries that make interacting with Snowflake pretty simple. Snowflake is secure and meets the most secure regulatory standards, such as HIPAA, FedRAMP, and PCI DSS. When you store your data in Snowflake, your data is encrypted in transit and at rest by default, and it’s automatically replicated, restored, and backed up to ensure business continuity. Plan smarter with our Snowflake pricing calculator – accurate, fast, and reliable.
Looking for the best ETL tools to connect your data sources? Rest assured, Hevo’s no-code platform helps streamline your ETL process. Try Hevo and equip your team to:
- Integrate data from 150+ sources(60+ free sources).
- Utilize drag-and-drop and custom Python script features to transform your data.
- Risk management and security framework for cloud-based systems with SOC2 Compliance.
Try Hevo and discover why 2000+ customers have chosen Hevo over tools like AWS DMS to upgrade to a modern data stack.
Get Started with Hevo for FreeMethod 1: Use Hevo ETL to Move Data From Postgres to Snowflake With Ease
Using Hevo, the official Snowflake ETL partner, you can easily load data from Postgres to Snowflake with just 3 simple steps: Select your Source, Provide Credentials, and Load to the Destination. Hevo is the only real-time ELT No-code Data Pipeline platform that cost-effectively automates data pipelines that are flexible to your needs.
Step 1: Configure PostgreSQL as Source
Connect your PostgreSQL account to Hevo’s platform. Hevo has an in-built PostgreSQL Integration that connects to your account within minutes.
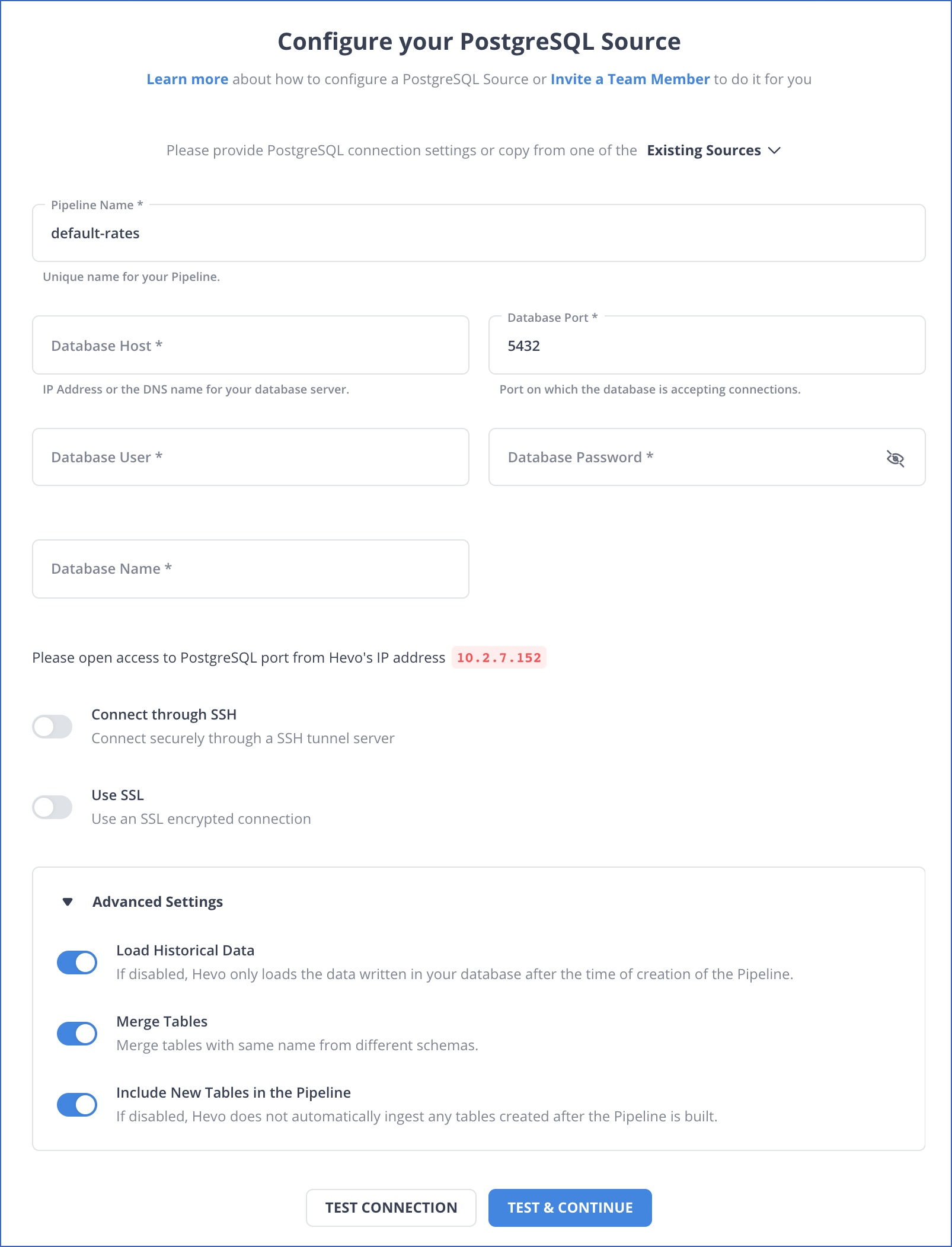
Step 2: Configure Snowflake as a Destination
Perform the following steps to configure Snowflake as a Destination in Hevo:
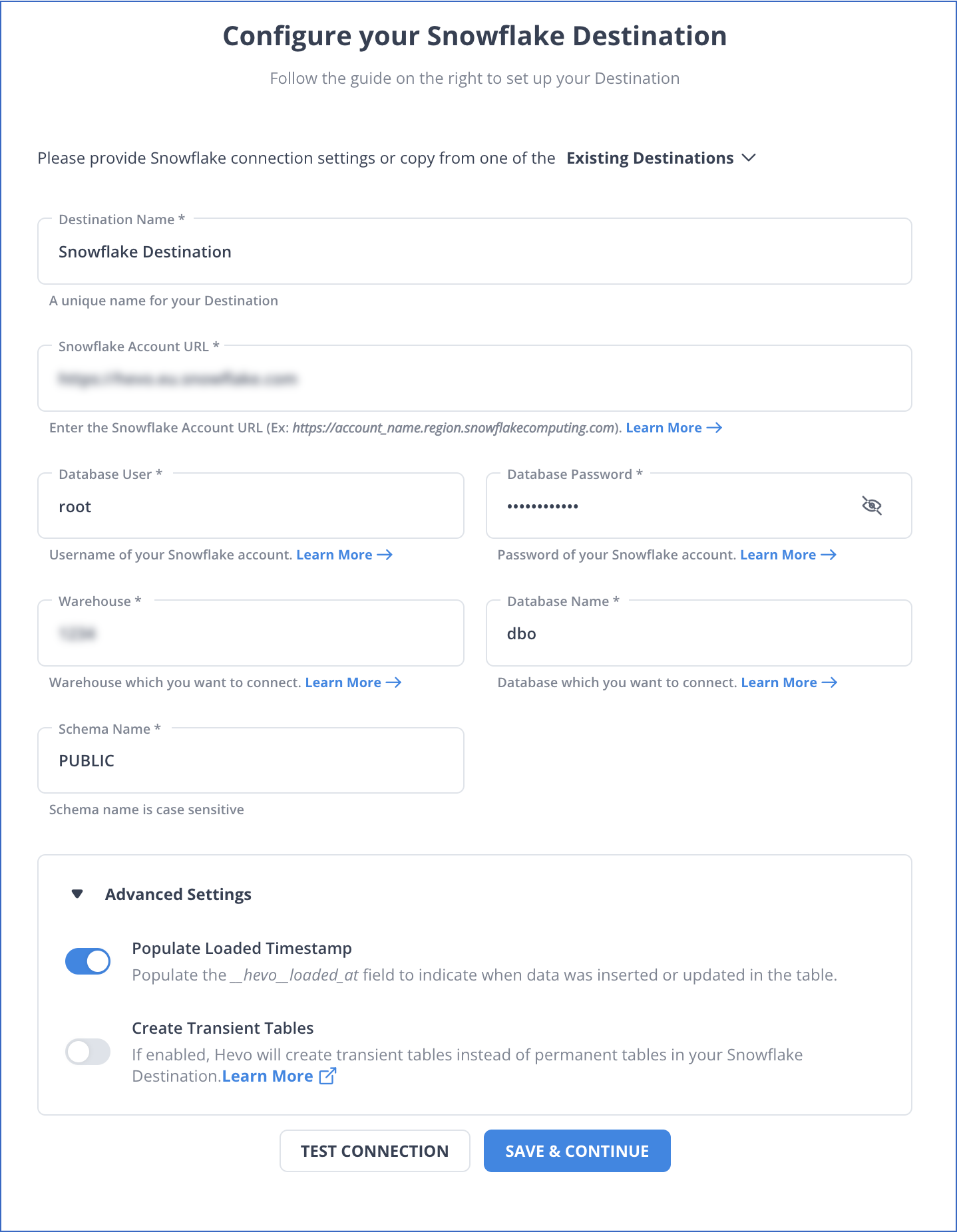
By completing the above steps, you have successfully completed Postgres Snowflake integration.
To know more, check out:
Check out some of the cool features of Hevo:
- Incremental Data Load: Hevo allows the transfer of data that has been modified in real-time. This ensures efficient utilization of bandwidth on both ends.
- Schema Management: Hevo takes away the tedious task of schema management & automatically detects the schema of incoming data and maps it to the destination schema.
- Scalable Infrastructure: Hevo has in-built integrations for 150+ sources that can help you scale your data infrastructure as required.
Method 2: Write a Custom Code to Move Data from Postgres to Snowflake
As in the above-shown figure, the four steps to replicate Postgres to Snowflake using custom code (Method 2) are as follows:
1. Extract Data from Postgres
COPY TO command is the most popular and efficient method to extract data from the Postgres table to a file. We can also use the pg_dump utility for the first time for full data extraction. We will have a look at both methods.
a. Extract Data Using the COPY Command
As mentioned above, COPY TO is the command used to move data between Postgres tables and standard file-system files. It copies an entire table or the results of a SELECT query to a file:
COPY table or sql_query TO out_file_name WITH options.
Example:
COPY employees TO 'C:tmpemployees_db.csv' WITH DELIMITER ',' CSV HEADER;
COPY (select * from contacts where age < 45) TO 'C:tmpyoung_contacts_db.csv' WITH DELIMITER ',' CSV HEADER;
Next, we can have a look at how the COPY command can be used to extract data from multiple tables using a PL/PgSQL procedure. Here, the table named tables_to_extract contains details of tables to be exported.
CREATE OR REPLACE FUNCTION table_to_csv(path TEXT) RETURNS void AS $
declare
tables RECORD;
statement TEXT;
begin
FOR tables IN
SELECT (schema || '.' || table_name) AS table_with_schema
FROM tables_to_extract
LOOP
statement := 'COPY ' || tables.table_with_schema || ' TO ''' || path || '/' || tables.table_with_schema || '.csv' ||''' DELIMITER '';'' CSV HEADER';
EXECUTE statement;
END LOOP;
return;
end;
$ LANGUAGE plpgsql;
SELECT db_to_csv('/home/user/dir'/dump); -- This will create one csv file per table, in /home/user/dir/dump/
Sometimes, you want to extract data incrementally. To do that, add more metadata like the timestamp of the last data extraction to the table tables_to_extract and use that information while creating the COPY command to extract data changed after that timestamp.
Consider you are using a column named last_pull_time corresponding to each table in the table tables_to_extract, which stores the last successful data pull time. Each time data in the table is modified after that, the timestamp has to be pulled. The body of the loop in the procedure will change like this:
Here, a dynamic SQL is created with a predicate comparing last_modified_time_stamp from the table to be extracted and last_pull_time from table list_of_tables.
begin
FOR tables IN
SELECT (schema || '.' || table_name) AS table_with_schema, last_pull_time AS lt
FROM tables_to_extract
LOOP
statement := 'COPY (SELECT * FROM ' || tables.table_with_schema || ' WHERE last_modified_time_stamp > ' || last_pull_time ') TO ' '' || path || '/' || tables.table_with_schema || '.csv' ||''' DELIMITER '';'' CSV HEADER';
EXECUTE statement;
END LOOP;
return;
End;
b. Extract Data Using the pg_dump
As mentioned above, pg_dump is the utility for backing up a Postgres database or tables. It can also be used to extract data from the tables.
Example syntax:
pg_dump --column-inserts --data-only --table=<table> <database> > table_name.sql
Here, the output file table_name.sql will be in the form of INSERT statements like
INSERT INTO my_table (column1, column2, column3, ...)
VALUES (value1, value2, value3, ...);
This output has to be converted into a CSV file with the help of a small script in your favorites like Bash or Python.
2. Postgres to Snowflake Data Types Conversion
Domain-specific logic will be applied while transferring data. Apart from that, the following things are to be noted while migrating data to avoid surprises.
- Snowflake allows almost all date/time formats. The format can be explicitly specified while loading data to the table using the File Format Option, which we will discuss in detail later. The complete list of supported date/time formats can be found.
3. Stage Data Files
Before Postgres to Snowflake replication, it needs to be uploaded to a temporary location, which is called staging. There are two types of stages – internal and external.
a. Internal Stage
Each user and table is automatically allocated an internal stage for data files. It is also possible to create named internal stages.
- The user was named and accessed as ‘@~.’
- The name of the table stage will be the same as that of the table.
- The user or table stages can’t be altered or dropped.
- The user or table stages do not support setting file format options.
As mentioned above, Internal Named Stages can be created by the user using the respective SQL statements. It provides a lot of flexibility while loading data by giving you options to assign file format and other options to named stages.
While running DDL and commands like load data, SnowSQL is quite a handy CLI client that can be used to run those commands and is available in Linux/Mac/Windows. Read more about the tool and options.
Below are some example commands to create a stage:
Create a names stage:
create or replace stage my_postgres_stage
copy_options = (on_error='skip_file')
file_format = (type = 'CSV' field_delimiter = '|' skip_header = 1);
PUT command is used to stage data files to an internal stage. The syntax of the command is as given below :
PUT file://path_to_file/filename internal_stage_name
Example:
Upload a file named cnt_data.csv in the /tmp/postgres_data/data/ directory to an internal stage named postgres_stage.
put file:////tmp/postgres_data/data/cnt_data.csv @postgres_stage;
There are many useful options that can help improve performance, such as setting parallelism while uploading the file, automatic compression of data files, etc. More information about those options is listed here.
b. External Stage
Amazon S3 and Microsoft Azure are external staging locations currently supported by Snowflake. We can create an external stage with any of those locations and load data to a Snowflake table.
To create an external stage on S3, IAM credentials have to be given. If the data is encrypted, then encryption keys should also be given.
create or replace stage postgre_ext_stage url='s3://snowflake/data/load/files/'
credentials=(aws_key_id='111a233b3c' aws_secret_key='abcd4kx5y6z');
encryption=(master_key = 'eSxX0jzYfIjkahsdkjamtnBKONDwOaO8=');
Data to the external stage can be uploaded using AWS or Azure web interfaces. For S3, you can upload it using the AWS web console or any AWS SDK or third-party tools.
4. Copy Staged Files from Postgres to Snowflake Table
COPY INTO is the command used to load the contents of the staged file(s) from Postgres to the Snowflake table. To execute the command, computing resources in the form of virtual warehouses are needed. You know more about it this command in the Snowflake ETL best practices.
Example:
To load from a named internal stage
COPY INTO postgres_table
FROM @postgres_stage;
Loading from the external stage. Only one file is specified.
COPY INTO my_external_stage_table
FROM @postgres_ext_stage/tutorials/dataloading/contacts_ext.csv;
You can even copy directly from an external location:
COPY INTO postgres_table
FROM s3://mybucket/snow/data/files
credentials = (aws_key_id='$AWS_ACCESS_KEY_ID' aws_secret_key='$AWS_SECRET_ACCESS_KEY')
encryption = (master_key = 'eSxX0jzYfIdsdsdsamtnBKOSgPH5r4BDDwOaO8=')
file_format = (format_name = csv_format);
Files can be specified using patterns.
COPY INTO pattern_table
FROM @postgre_stage
file_format = (type = 'CSV')
pattern='.*/.*/.*[.]csv[.]gz';
Check out the full list of options. So, now you have finally loaded data from Postgres to Snowflake.
5. Update Snowflake Table
We have discussed how to extract data incrementally from PostgreSQL. Now we will look at how to migrate data from Postgres to Snowflake effectively.
As we discussed in the introduction, Snowflake is not based on any big data framework and does not have any limitations for row-level updates like in systems like Hive. It supports row-level updates making delta data migration much easier. Basic idea is to load incrementally extracted data into an intermediate table and modify records in the final table as per data in the intermediate table.
There are three popular methods to modify the final table once data is loaded into the intermediate table.
- Update the rows in the final table and insert new rows from the intermediate table which are not in the final table.
UPDATE final_target_table t
SET t.value = s.value
FROM intermed_delta_table in
WHERE t.id = in.id;
INSERT INTO final_target_table (id, value)
SELECT id, value
FROM intermed_delta_table
WHERE NOT id IN (SELECT id FROM final_target_table);
- Delete all records from the final table which are in the intermediate table. Then insert all rows from the intermediate table to the final table.
DELETE .final_target_table f
WHERE f.id IN (SELECT id from intermed_delta_table);
INSERT final_target_table (id, value)
SELECT id, value
FROM intermed_table;
- MERGE statement: Inserts and updates can be done with a single MERGE statement and it can be used to apply changes in the intermediate table to the final table with one SQL statement.
MERGE into final_target_table t1 using intermed_delta_table t2 on t1.id = t2.id
WHEN matched then update set value = t2.value
WHEN not matched then INSERT (id, value) values (t2.id, t2.value);
Limitations of Using Custom Scripts for Postgres to Snowflake Connection
Here are some of the limitations associated with the use of custom scripts to connect Postgres to Snowflake.
Complexity
This method necessitates a solid grasp of PostgreSQL and Snowflake, including their respective data types, SQL syntax, and file-handling features. Some may find this to be a challenging learning curve because not all may have substantial familiarity with SQL or database management.
Time-consuming
It can take a while to write scripts and troubleshoot any problems that may occur, particularly with larger databases or more intricate data structures.
Error-prone
In human scripting, mistakes can happen. A small error in the script might result in inaccurate or corrupted data.
No Direct Support
You cannot contact a specialized support team in the event that you run into issues. For help with any problems, you’ll have to rely on the manuals, community forums, or internal knowledge.
Scalability Issues
The scripts may need to be modified or optimized to handle larger datasets as the volume of data increases. Without substantial efforts, this strategy might not scale effectively.
Inefficiency with Large Datasets
It might not be the most effective method to move big datasets by exporting them to a file and then importing them again, especially if network bandwidth is limited. Methods of direct data transmission could be quicker.
Postgres to Snowflake Data Replication Use Cases
Let’s look into some use cases of Postgres-Snowflake replication.
Transferring Postgres data to Snowflake
Are you feeling constrained by your Postgres configuration on-premises? Transfer your data to Snowflake’s endlessly scalable cloud platform with ease. Take advantage of easy performance enhancements, cost-effectiveness, and the capacity to manage large datasets.
Data Warehousing
Integrate data into Snowflake’s robust data warehouse from a variety of sources, including Postgres. This can help uncover hidden patterns, get a better understanding of your company, and strengthen strategic decision-making.
Advanced Analytics
Utilize Snowflake’s quick processing to run complex queries and find minute patterns in your Postgres data. This can help you stay ahead of the curve, produce smart reports, and gain deeper insights.
Artificial Intelligence and Machine Learning
Integrate your Postgres data seamlessly with Snowflake’s machine-learning environment. As a result, you can develop robust models, provide forecasts, and streamline processes to lead your company toward data-driven innovation.
Collaboration and Data Sharing
Colleagues and partners can securely access your Postgres data within the collaborative Snowflake environment. Hence, this integration helps promote smooth communication and expedite decision-making and group achievement.
Backup and Disaster Recovery
Transfer your Postgres data to the dependable and safe cloud environment offered by Snowflake. You can be assured that your data is constantly accessible and backed up, guaranteeing company continuity even in the event of unanticipated events.
Additional Resources for PostgreSQL Integrations and Migrations
- PostgreSQL to Oracle Migration
- Connect PostgreSQL to MongoDB
- Connect PostgreSQL to Redshift
- Integrate Postgresql to Databricks
- Export a PostgreSQL Table to a CSV File
Conclusion
High-performing data warehouse solutions like Snowflake are becoming more popular and becoming an integral part of a modern analytics pipeline. Migrating data from various data sources to this kind of cloud-native solution i.e from Postgres to Snowflake, requires expertise in the cloud, data security, and many other things like metadata management.
Find out how to move data from Azure PostgreSQL to Snowflake to enhance your analytics capabilities. Our resource provides simple steps for effective data migration and integration.
If you are looking for an ETL tool that facilitates the automatic migration and transformation of data from Postgres to Snowflake, then Hevo is the right choice for you. Hevo is a No-code Data Pipeline. It supports pre-built integration from 150+ data sources at a reasonable price. With Hevo, you can perfect, modify and enrich your data conveniently.
FAQ on PostgreSQL to Snowflake
How to migrate data from PostgreSQL to Snowflake?
To migrate data from PostgreSQL to Snowflake, you can follow these steps:
1. Connect PostgreSQL as Source with Hevo and fill in your details
2. Configure Snowflake as your Target Destination
Is PostgreSQL compatible with Snowflake?
PostgreSQL is not natively compatible with Snowflake, but you can migrate data between the two using CSV files, ETL tools, or custom scripts. Snowflake supports various tools and connectors to facilitate migration, making it relatively easy to move data.
How to migrate data between PostgreSQL databases?
To migrate data between PostgreSQL databases, you can use pg_dump
to export the database or selected tables and then use psql
or pg_restore
to import the data into another PostgreSQL instance. You can also use tools like pgAdmin
or third-party migration tools for more complex scenarios.