Python SQL Server integration enables the development of robust, data-driven applications with enhanced analytical capabilities and seamless database operations.
For establishing a reliable connection between Python and SQL Server,
you should install pyodbc,
configure the connection string with server credentials,
initialize database cursors,
and implement query execution methods.
The setup involves installing pyodbc via pip, creating connection strings with driver specifications, establishing database connections through pyodbc.connect(), and executing SQL queries using cursor objects.
With this integration, developers can efficiently perform database operations, ensuring smooth interaction between Python scripts and SQL Server databases for advanced analytics and automation workflows.
Integrating Python with SQL Server opens up powerful possibilities for data analysis, automation, and application development. Python, known for its simplicity and versatility, combined with SQL Server’s robust database management capabilities, creates a seamless workflow for handling and analyzing large datasets. Whether you’re a data scientist, developer, or database administrator, mastering Python SQL Server integration can significantly boost your productivity. In this blog, explore the benefits of this integration and provide step-by-step guidance to set it up effectively using Pyodbc.
Table of Contents
What is SQL Server?
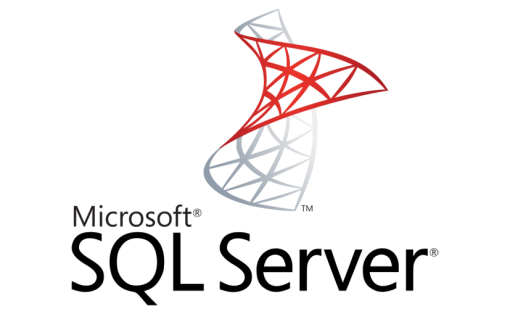
Microsoft SQL Server is a widely used Relational Database Management System (RDBMS) designed to provide data storage and retrieval facility to users as per their requirements. Read about data modeling in SQL Server Management Studio to store data in database.
The key features of Microsoft SQL Server are as follows:
- Advanced Analytics: You can easily leverage the data stored in Microsoft SQL Server to implement a comprehensive analysis as per your requirements. Moreover, you can make use of Microsoft SQL Server Analysis Service (SSAS) which to excel at Data Mining tasks.
- Accelerated Data Recovery: Microsoft SQL Server has an exceptional recovery and rollback mechanism that automatically switches on during a server crash. The process of database recovery leverages Transaction Logs which drastically decreases the delay time.
- Advanced Encryption: Since 2016, Microsoft SQL Server relies on a new Encryption Technology known as AlwaysEncrypted that offers transparent Column Encryption without providing Database Administrators any access to Decryption Keys.
- Intelligent Query Processing: Microsoft SQL Server hosts an in-built Query Optimizer that operates on an execution plan. This plan has been optimized to provide performance enhancements in terms of Dynamic Memory Grants, Batch Mode, Table Variable Deferred Compilation, etc.
Effortlessly connect and transfer data to SQL Server with Hevo’s no-code platform. Automate your data workflows and keep your data up-to-date in real-time without any manual intervention.
Why Choose Hevo?
- Seamless Integration: Connect SQL Server with over 150+ sources and destinations.
- Real-Time Sync: Ensure your SQL Server data is always current with continuous, automated updates.
- No-Code Setup: Easily manage your data pipelines without the need for coding or technical expertise.
What is Python?
Python is a versatile general-purpose Programming Language. Its small learning curve coupled with its robustness has made it one of the most popular Programming Languages today.
The following features are responsible for Python Programming Language’s popularity today:
- Large Communities: Due to Python’s immense popularity, a huge active community of programmers is available online that contributes to this language’s modules and libraries. Moreover, this vast support community is ready to help in case you or any other coder gets stuck in a programming issue.
- Beginner Friendly: The Python Programming Language offers a hassle-free environment for developers. Its straightforward workflow is suitable for everyone and entry-level coders are drawn to it. Moreover, you can use and distribute its open-source codes for commercial purposes free of cost.
- Robust Applications: Its simple syntax operates on natural human-readable language making it the go-to choice of projects on Python Programming Language, which is faster as compared to other Programming Languages. Furthermore, its versatile nature makes it the ideal choice for Web Development and Machine Learning projects. Read about setting up ETL using python.
What are the Benefits of Python SQL Server Connection?
Combining Python Programming Language with SQL Servers will enhance your business in the following ways:
- A Python SQL Server Integration allows AI developers to leverage the SQL Server’s functionality directly from their Python code. This will make it much easier for these developers to embed data analytics, AI functionality, and machine learning features into an existing application’s code.
- The Python SQL Server connection provides a significant edge for development work. Other languages like R, when integrated with SQL Server, enhance only the analytical aspect of programming. However, Python, when used along with SQL Servers, allows developers to perform real-time data streaming and operations much more easily.
- The Python SQL Server integration setup provides faster data processing than a network-based system over TCP, HTTP, etc. This is because it utilizes local platform resources, including the file system, GPU, etc., for building real-time integrations and relies on universal data formats such as JSON and XML.
How to Set Up Python SQL Server Integration using Pyodbc
Prerequisites
- Python installed on your device
- SQL installed on your device
- Access to Jupyter Notebook or any IDE
Step 1: Install pyodbc on your device
Step 1. a) Open your Command Prompt and type in the following code to successfully install pyodbc, allowing us to connect to the SQL Server database.
Pip install pyodbc

Step 2: Open Jupyter Notebook to type in the Python Script
Step 2. a) To access jupyter notebook, go to your command prompt and type in jupyter notebook.
Step 2. b) Import the following
- pyodbc
- pandas
Step 2. c) Now we will type the following connection string, I have given the connection string below.
connection_string = ("Driver={};"
"Server=DatabaseServerName;"
"Database=<Database_name>;"
"Trusted_Connection=yes;")
Note:
- In the Driver, you must type the driver name installed in your SQL Server.
- You have to paste the SQL Server address into the server, which you can find by logging in to your SQL Server Management Studio.
- Next is the database name that you want to connect
- Trusted Connection is yes if you are using Windows, If you have a separate server ID, you have to put that in your script
Step 2. d) After you have written the script, go to your Jupyter notebook and paste it there. Now, to establish the connection, you need to run the following command:
pyodbc.connect(connection_string)
Step 3: Query your SQL Data
Step 3. a) To execute any query, we need to initialize a cursor so that we can run our queries within that cursor.
Step 3. b) To create a cursor, run the following command:
Cursor = Connection.cursor()
Step 3. c) To execute a SQL query, run the following command:
Cursor.execute(“Select * from Dept”)
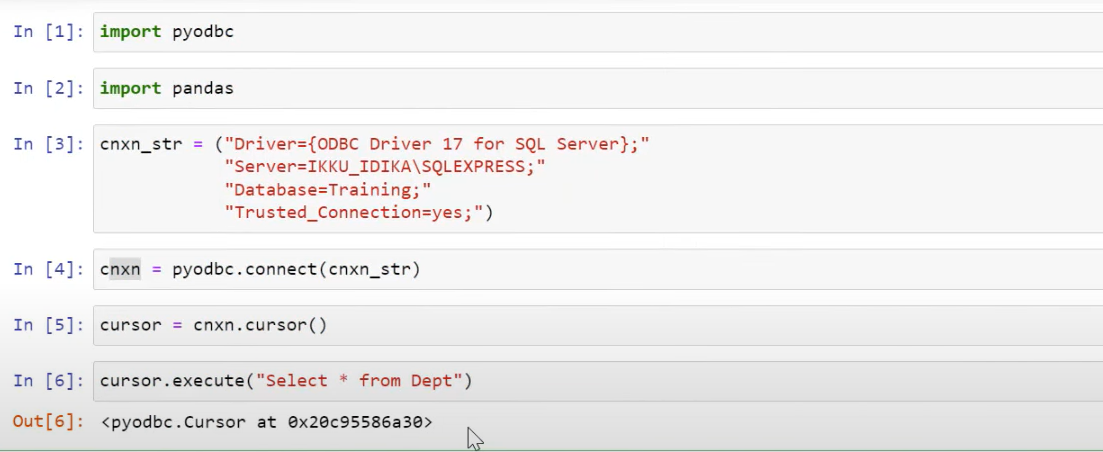
Step 4: Run the SQL Query
Step 4. a) Make a dataframe and run the query to fetch data from the Dept table
df = pd.read_sql(“Select * from Dept”,connection)
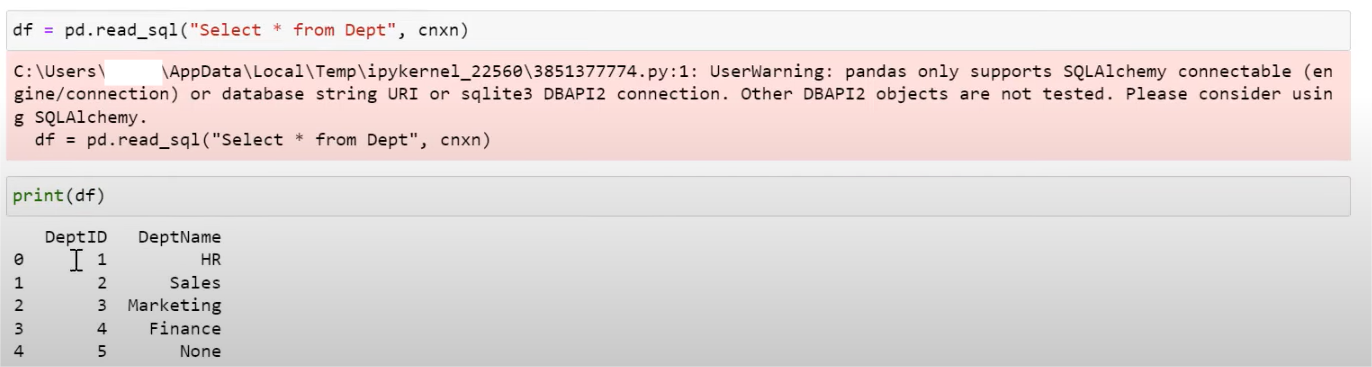
You can also read more about:
- S3 to SQL Server
- Salesforce to SQL Server
- SQL Server to S3
- Postgres on Google Cloud SQL to SQL Server
Conclusion
Now, to run SQL queries or perform Data Analytics on your SQL Server data, you first need to export this data to a Data Warehouse. This will require you to custom-code complex scripts to develop the ETL processes. Hevo Data is the only real-time ELT No-code Data Pipeline platform that cost-effectively automates data pipelines that are flexible to your needs.
It can automate your data transfer process, hence allowing you to focus on other aspects of your business like Analytics, Customer Management, etc. This platform allows you to transfer data from sources like Microsoft SQL to Cloud-based Data Warehouses like Amazon Redshift, Snowflake, Google BigQuery, etc. It will provide you with a hassle-free experience and make your work life much easier.
Try Hevo and see the magic for yourself. Sign up for a free 14-day trial to streamline your data integration process. You may examine Hevo’s pricing plans and decide on the best plan for your business needs.
FAQ on Python to SQL Server
How to connect to a SQL Server database using Python?
1. Install pyodbc
2. Connect to SQL server
How to connect SQL file to Python?
If you have SQL queries saved in a .sql file, you can read the file and execute the queries using the database connection.
How to connect MySQL server with Python?
1. Install mysql-connector-python
2. Connect to MySQL Server
How to run a SQL Server query in Python?
query = “SELECT * FROM your_table_name”
cursor.execute(query)
rows = cursor.fetchall()
for row in rows:
print(row)